Singular Value Decomposition and Manifold Regulation-based Multi-label Classification.
International Conference on Bioinformatics and Intelligent Computing (BIC)(2022)
摘要
In multi-label classification, an instance may contain multiple labels simultaneously, so it is applied widely in many aspects such as product recommendation, biological function prediction and document annotation. However, the high-dimensional problem of feature space and sparseness problem of label space bring great challenges to multi-label classification. To solve these problems, this paper proposes a Singular Value Decomposition and Manifold Regulation-based Multi-label Classification (SDMR) framework. In this framework, the label space is transformed into latent label space by singular value decomposition (SVD). Then an improved principal component analysis method based on manifold regularization (PCAM) is proposed, which can find a few effective features to maximize the dependence between low-dimensional features and latent labels. Finally, a powerful multi-label classifier is learned from low-dimensional spaces. To verify the effectiveness of the proposed algorithm, extensive experiments are conducted on ten real-world multi-label data sets. Compared with the traditional multi-label classification algorithms, the proposed algorithm through dual spaces reduction can achieve better classification performance.
更多查看译文
AI 理解论文
溯源树
样例
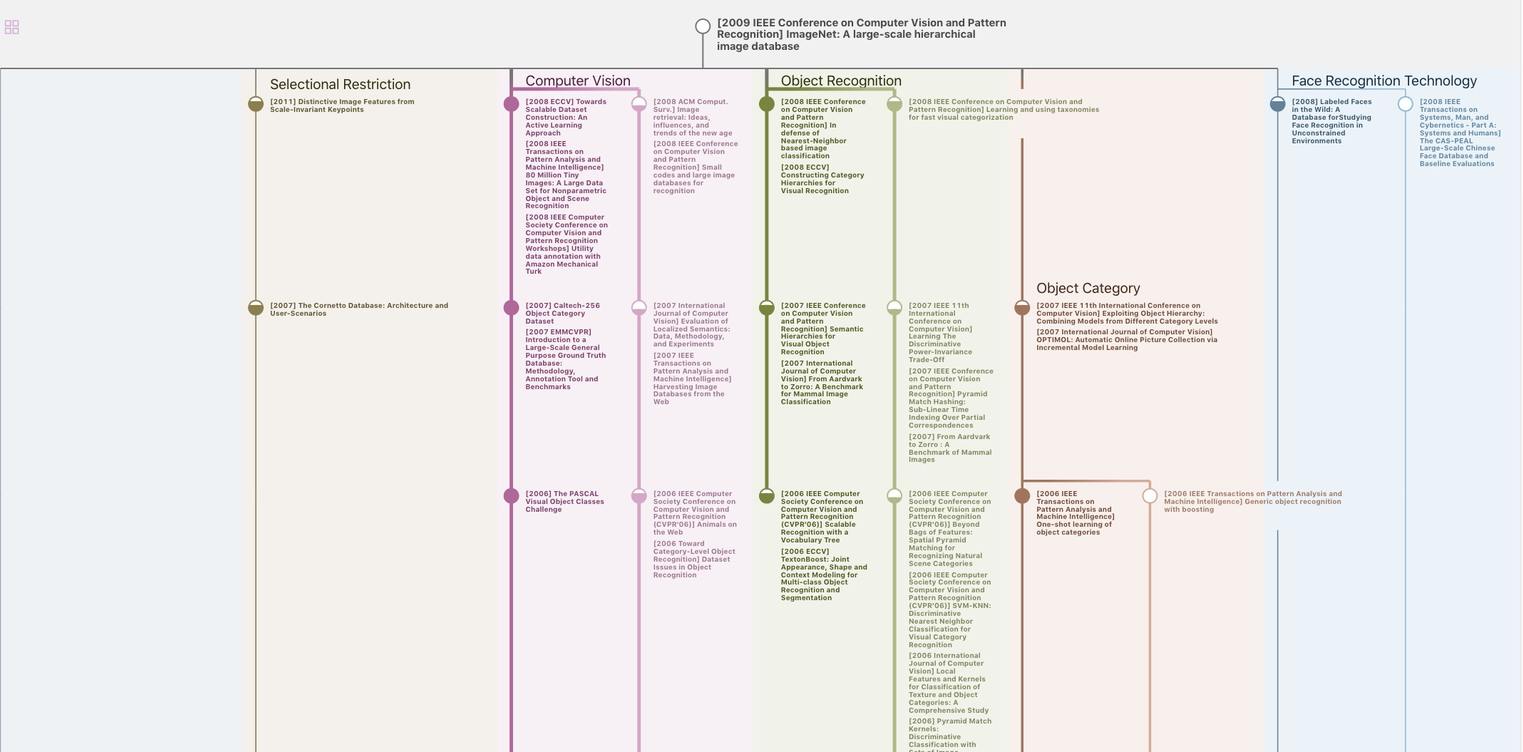
生成溯源树,研究论文发展脉络
Chat Paper
正在生成论文摘要