Targeted Policy Recommendations using Outcome-aware Clustering.
ACM SIGCAS Conference on Computing and Sustainable Societies (COMPASS)(2022)
摘要
Policy recommendations using observational data typically rely on estimating an econometric model on a sample of observations drawn from an entire population. However, different policy actions could potentially be optimal for different subgroups of a population. In this paper, we propose outcome-aware clustering, a new methodology to segment a population into different clusters and derive cluster-level policy recommendations. Outcome-aware clustering differs from conventional clustering algorithms across two basic dimensions. First, given a specific outcome of interest, outcome-aware clustering segments the population based on selecting a small set of features that closely relate with the outcome variable. Second, the clustering algorithm aims to generate near-homogeneous clusters based on a combination of cluster size-balancing constraints, inter and intra-cluster distances in the reduced feature space. We generate targeted policy recommendations for each outcome-aware cluster based on a standard multivariate regression of a condensed set of actionable policy features (which may partially overlap or differ from the features used for segmentation) from the observational data. We implement our outcome-aware clustering method on the Living Standards Measurement Study - Integrated Surveys on Agriculture (LSMS-ISA) dataset to generate targeted policy recommendations for improving farmers outcomes in sub-Saharan Africa. Based on a detailed analysis of the LSMS-ISA, we derive outcome-aware clusters of farmer populations across three sub-Saharan African countries and show that the targeted policy recommendations at the cluster level significantly differ from policies that are generated at the population level.
更多查看译文
AI 理解论文
溯源树
样例
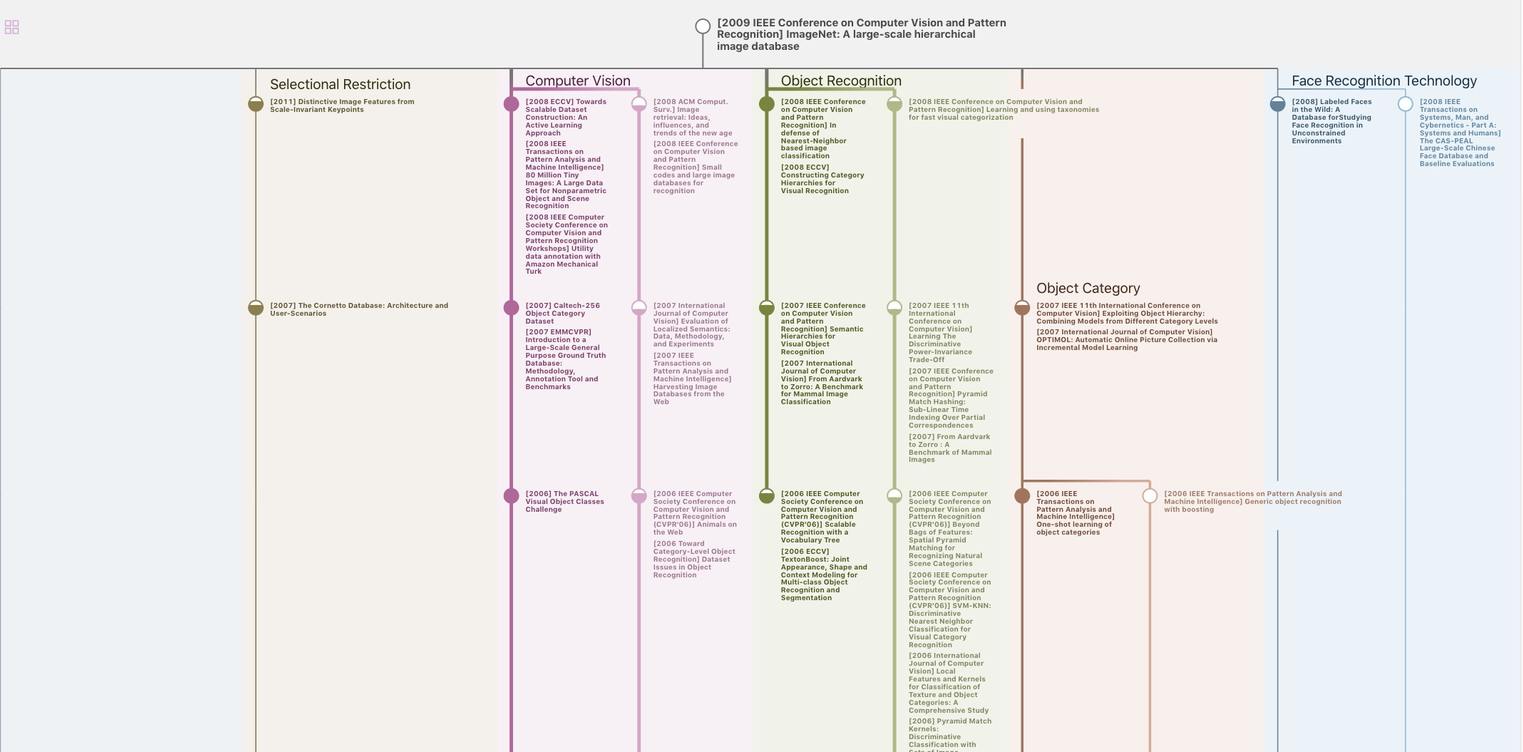
生成溯源树,研究论文发展脉络
Chat Paper
正在生成论文摘要