Deep Hierarchy Quantization Compression algorithm based on Dynamic Sampling
CoRR(2022)
摘要
Unlike traditional distributed machine learning, federated learning stores data locally for training and then aggregates the models on the server, which solves the data security problem that may arise in traditional distributed machine learning. However, during the training process, the transmission of model parameters can impose a significant load on the network bandwidth. It has been pointed out that the vast majority of model parameters are redundant during model parameter transmission. In this paper, we explore the data distribution law of selected partial model parameters on this basis, and propose a deep hierarchical quantization compression algorithm, which further compresses the model and reduces the network load brought by data transmission through the hierarchical quantization of model parameters. And we adopt a dynamic sampling strategy for the selection of clients to accelerate the convergence of the model. Experimental results on different public datasets demonstrate the effectiveness of our algorithm.
更多查看译文
关键词
compression,sampling,hierarchy
AI 理解论文
溯源树
样例
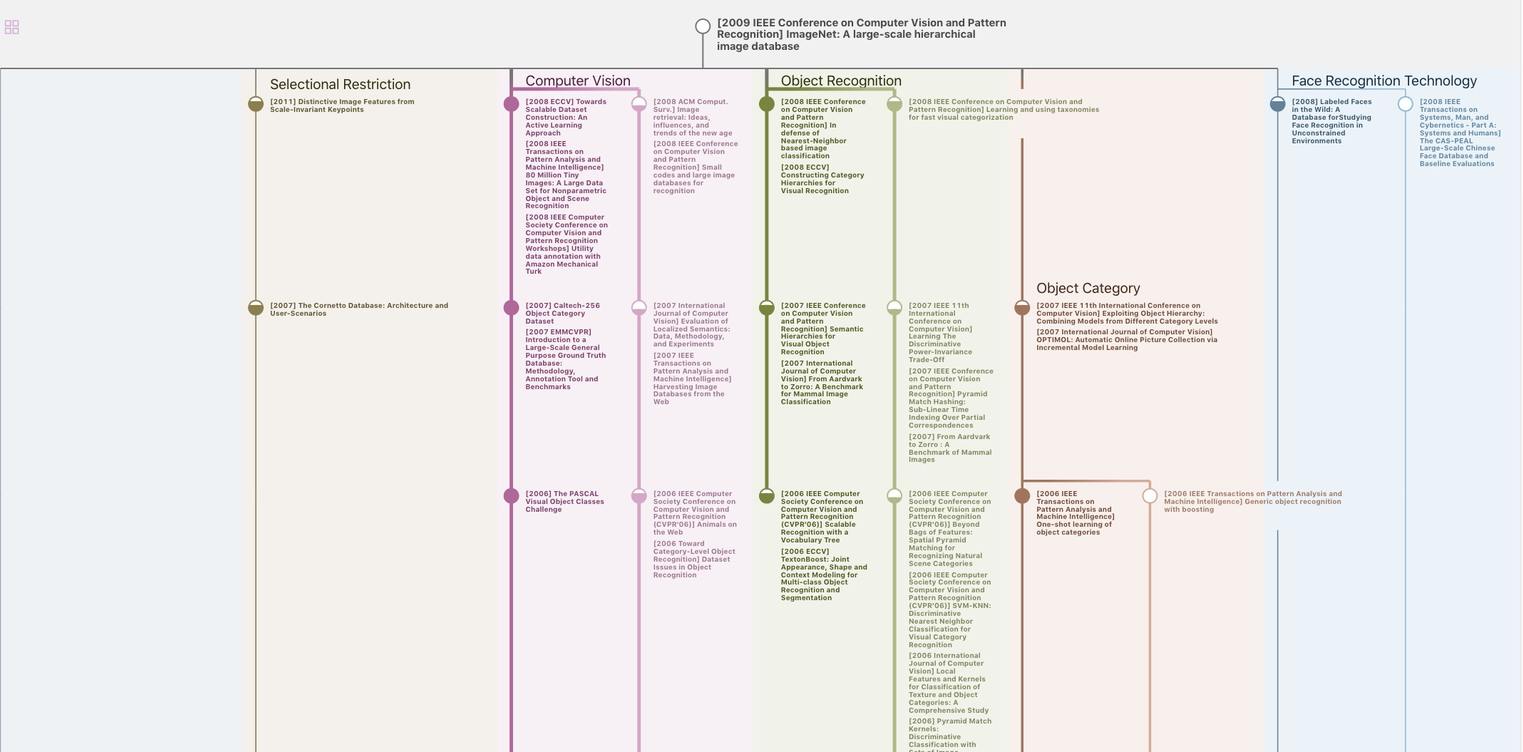
生成溯源树,研究论文发展脉络
Chat Paper
正在生成论文摘要