Bayesian Estimation of Experimental Parameters in Stochastic Inertial Systems: Theory, Simulations, and Experiments with Objects Levitated in Vacuum
arxiv(2023)
摘要
High-quality nanomechanical oscillators can sensitively probe force, mass, or displacement in experi-ments bridging the gap between the classical and quantum domain. Dynamics of these stochastic systems is inherently determined by the interplay between acting external forces, viscous dissipation, and ran -dom driving by the thermal environment. The relevance of inertia then dictates that both position and momentum must, in principle, be known to fully describe the system, which makes its quantitative exper-imental characterization rather challenging. We introduce a general method of Bayesian inference of the force field and environmental parameters in stochastic inertial systems that operates solely on the time series of recorded noisy positions of the system. The method is first validated on simulated trajectories of model stochastic harmonic and anharmonic oscillators with damping. Subsequently, the method is applied to experimental trajectories of particles levitating in tailored optical fields and used to characterize the dynamics of particle motion in a nonlinear Duffing potential, a static or time-dependent double-well poten-tial, and a nonconservative force field. The presented inference procedure does not make any simplifying assumptions about the nature or symmetry of the acting force field and provides robust results with tra-jectories 2 orders of magnitude shorter than those typically required by alternative inference schemes. In addition to being a powerful tool for quantitative data analysis, it can also guide experimentalists in choosing appropriate sampling frequency (at least 20 measured points per single characteristic period) and length of the measured trajectories (at least 10 periods) to estimate the force field and environmental characteristics with a desired accuracy and precision.
更多查看译文
关键词
stochastic inertial
AI 理解论文
溯源树
样例
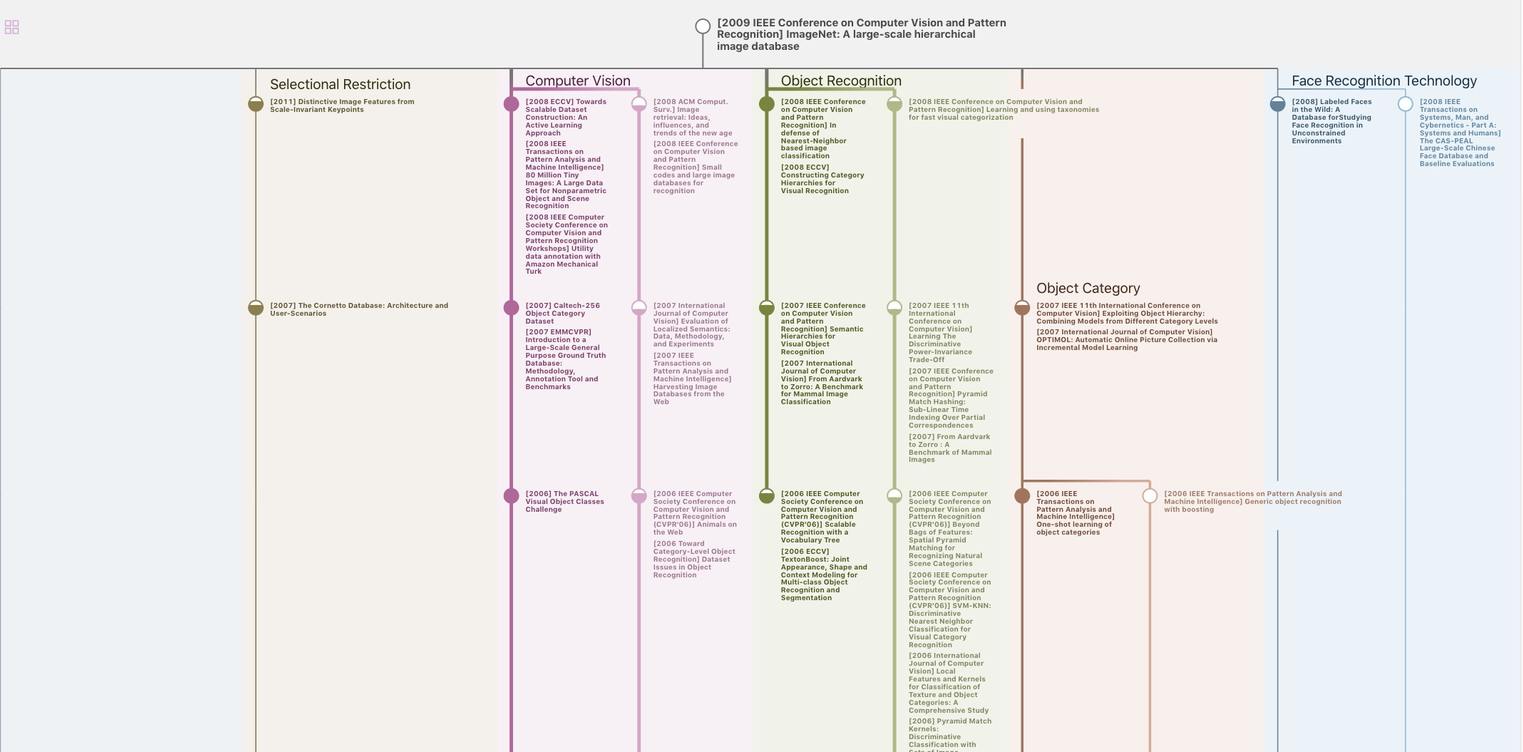
生成溯源树,研究论文发展脉络
Chat Paper
正在生成论文摘要