Deep-Learning-Based Few-Angle Cardiac SPECT Reconstruction Using Transformer
IEEE Transactions on Radiation and Plasma Medical Sciences(2023)
摘要
Convolutional neural networks (CNNs) have been extremely successful in various medical imaging tasks. However, because the size of the convolutional kernel used in a CNN is much smaller than the image size, CNN has a strong spatial inductive bias and lacks a global understanding of the input images. Vision Transformer, a recently emerged network structure in computer vision, can potentially overcome the limitations of CNNs for image-reconstruction tasks. In this work, we proposed a slice-by-slice Transformer network (SSTrans-3D) to reconstruct cardiac single-photon emission computed tomography (SPECT) images from 3-D few-angle data. To be specific, the network reconstructs the whole 3-D volume using a slice-by-slice scheme. By doing so, SSTrans-3D alleviates the memory burden required by 3-D reconstructions using Transformer. The network can still obtain a global understanding of the image volume with the Transformer attention blocks. Finally, already reconstructed slices are used as the input to the network so that SSTrans-3D can potentially obtain more informative features from these slices. Validated on porcine, phantom, and human studies acquired using a GE dedicated cardiac SPECT scanner, the proposed method produced images with clearer heart cavity, higher cardiac defect contrast, and more accurate quantitative measurements on the testing data as compared with a deep U-net.
更多查看译文
关键词
Dedicated cardiac single-photon emission computed tomography (SPECT),deep learning,few-angle imaging,GE Discovery NM 530/570c,Transformer
AI 理解论文
溯源树
样例
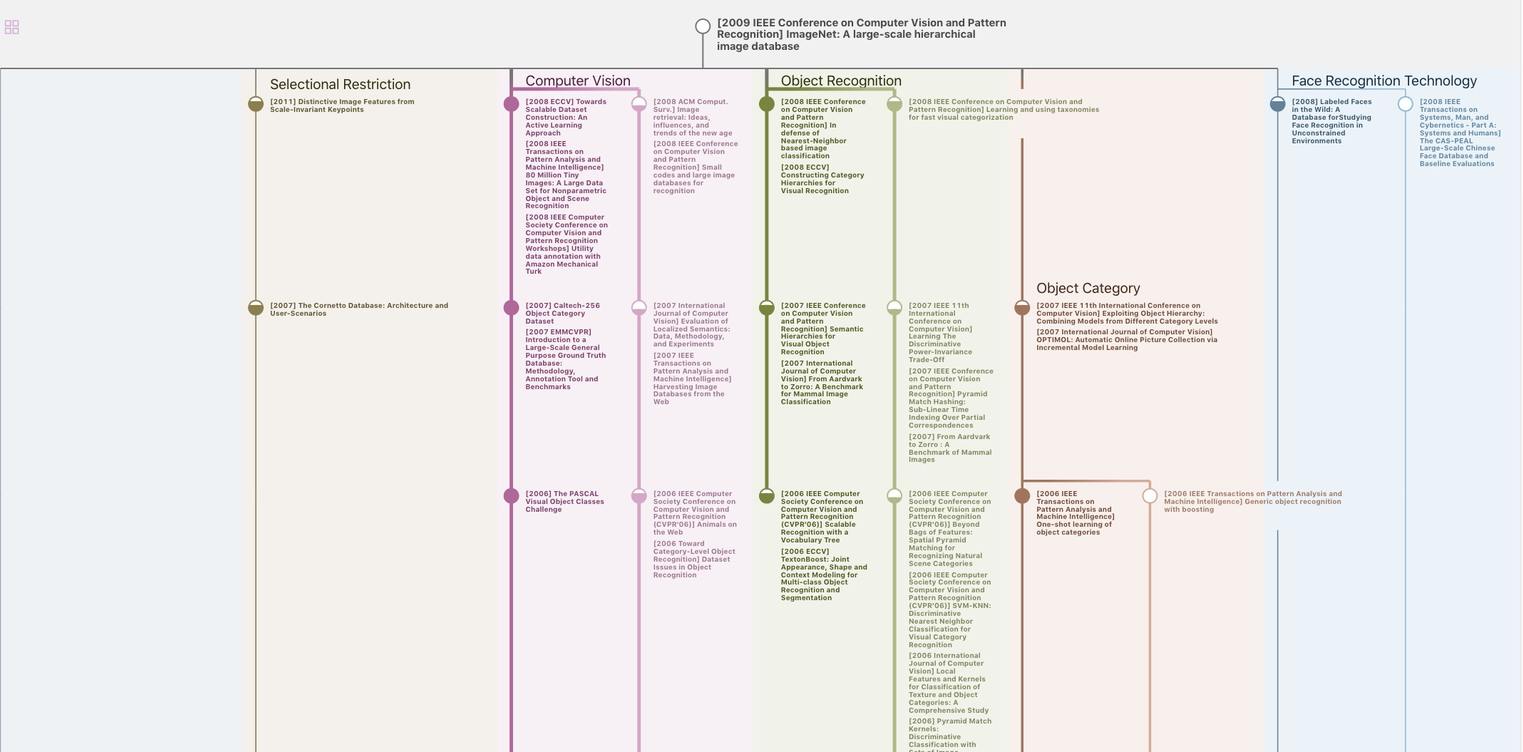
生成溯源树,研究论文发展脉络
Chat Paper
正在生成论文摘要