Self-supervise d semi-supervise d nonnegative matrix factorization for data clustering
Pattern Recognition(2023)
摘要
Semi-supervised nonnegative matrix factorization exploits the strengths of matrix factorization in suc-cessfully learning part-based representation and is also able to achieve high learning performance when facing a scarcity of labeled data and a large amount of unlabeled data. Its major challenge lies in how to learn more discriminative representations from limited labeled data. Furthermore, self-supervised learn-ing has been proven very effective at learning representations from unlabeled data in various learning tasks. Recent research works focus on utilizing the capacity of self-supervised learning to enhance semi-supervised learning. In this paper, we design an effective Self-Supervised Semi-Supervised Nonnegative Matrix Factorization (S4NMF) in a semi-supervised clustering setting. The S4NMF directly extracts a con-sensus result from ensembled NMFs with similarity and dissimilarity regularizations. In an iterative pro-cess, this self-supervisory information will be fed back to the proposed model to boost semi-supervised learning and form more distinct clusters. The proposed iterative algorithm is used to solve the given problem, which is defined as an optimization problem with a well-formulated objective function. In ad-dition, the theoretical and empirical analyses investigate the convergence of the proposed optimization algorithm. To demonstrate the effectiveness of the proposed model in semi-supervised clustering, we con-duct extensive experiments on standard benchmark datasets. The source code for reproducing our results can be found at https://github.com/ChavoshiNejad/S4NMF .(c) 2022 Elsevier Ltd. All rights reserved.
更多查看译文
关键词
Nonnegative matrix factorization,Semi-supervised learning,Self-supervised learning,Ensemble clustering
AI 理解论文
溯源树
样例
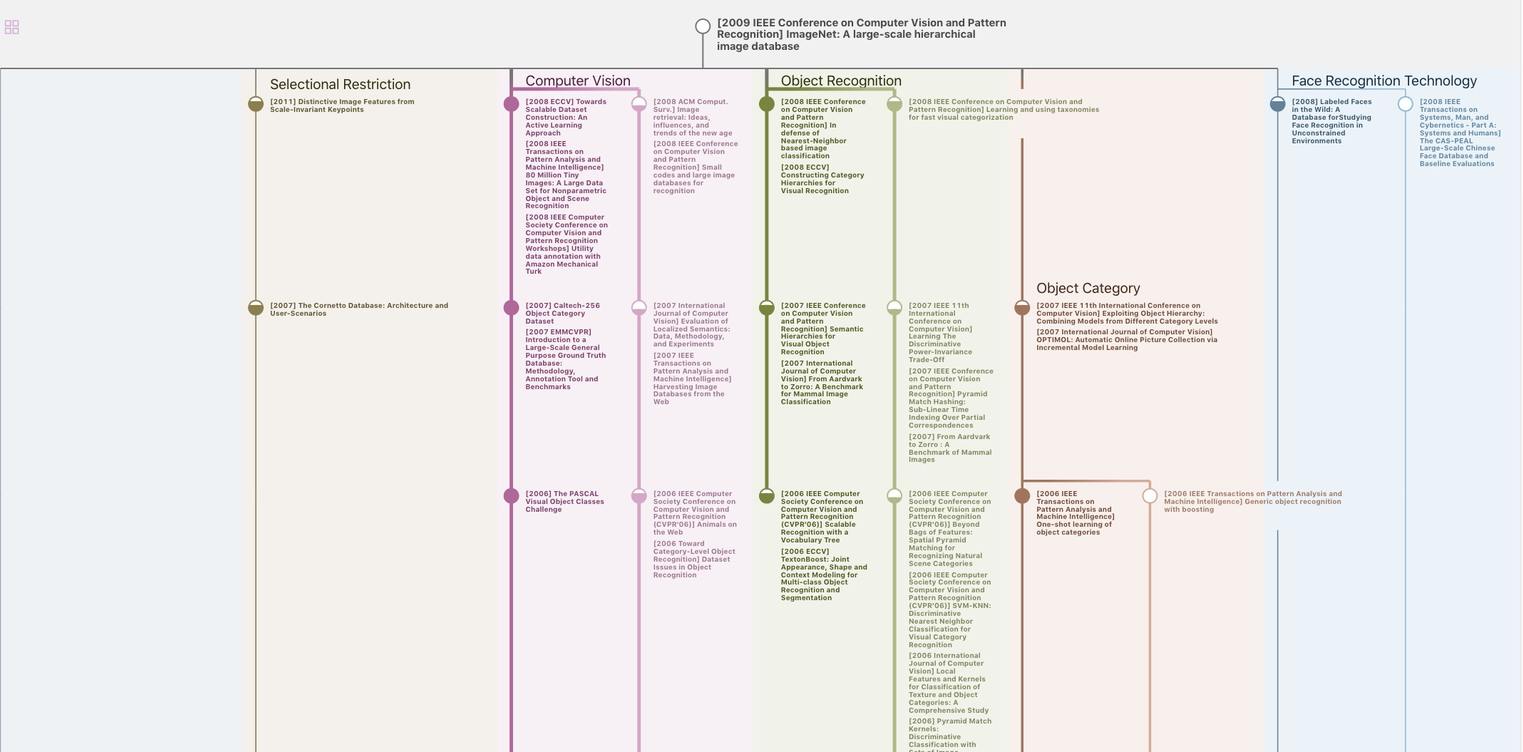
生成溯源树,研究论文发展脉络
Chat Paper
正在生成论文摘要