A 3D Clinical Face Phenotype Space of Genetic Syndromes using a Triplet-Based Singular Geometric Autoencoder
biorxiv(2024)
摘要
Clinical diagnosis of syndromes benefits strongly from objective facial phenotyping. This study introduces a novel approach to enhance clinical diagnosis through the development and exploration of a low-dimensional metric space referred to as the clinical face phenotypic space (CFPS). As a facial matching tool for clinical genetics, such CFPS can enhance clinical diagnosis. It helps to interpret facial dysmorphisms of a subject by placing them within the space of known dysmorphisms. In this paper, a triplet loss-based autoencoder developed by geometric deep learning (GDL) is trained using multi-task learning, which combines supervised and unsupervised learning approaches. Experiments are designed to illustrate the following properties of CFPSs that can aid clinicians in narrowing down their search space: a CFPS can 1) classify syndromes accurately, 2) generalize to novel syndromes, and 3) preserve the relatedness of genetic diseases, meaning that clusters of phenotypically similar disorders reflect functional relationships between genes. The proposed model consists of three main components: an encoder based on GDL optimizing distances between groups of individuals in the CFPS, a decoder enhancing classification by reconstructing faces, and a singular value decomposition layer maintaining orthogonality and optimal variance distribution across dimensions. This allows for the selection of an optimal number of CFPS dimensions as well as improving the classification capacity of the CFPS.
### Competing Interest Statement
The authors have declared no competing interest.
更多查看译文
AI 理解论文
溯源树
样例
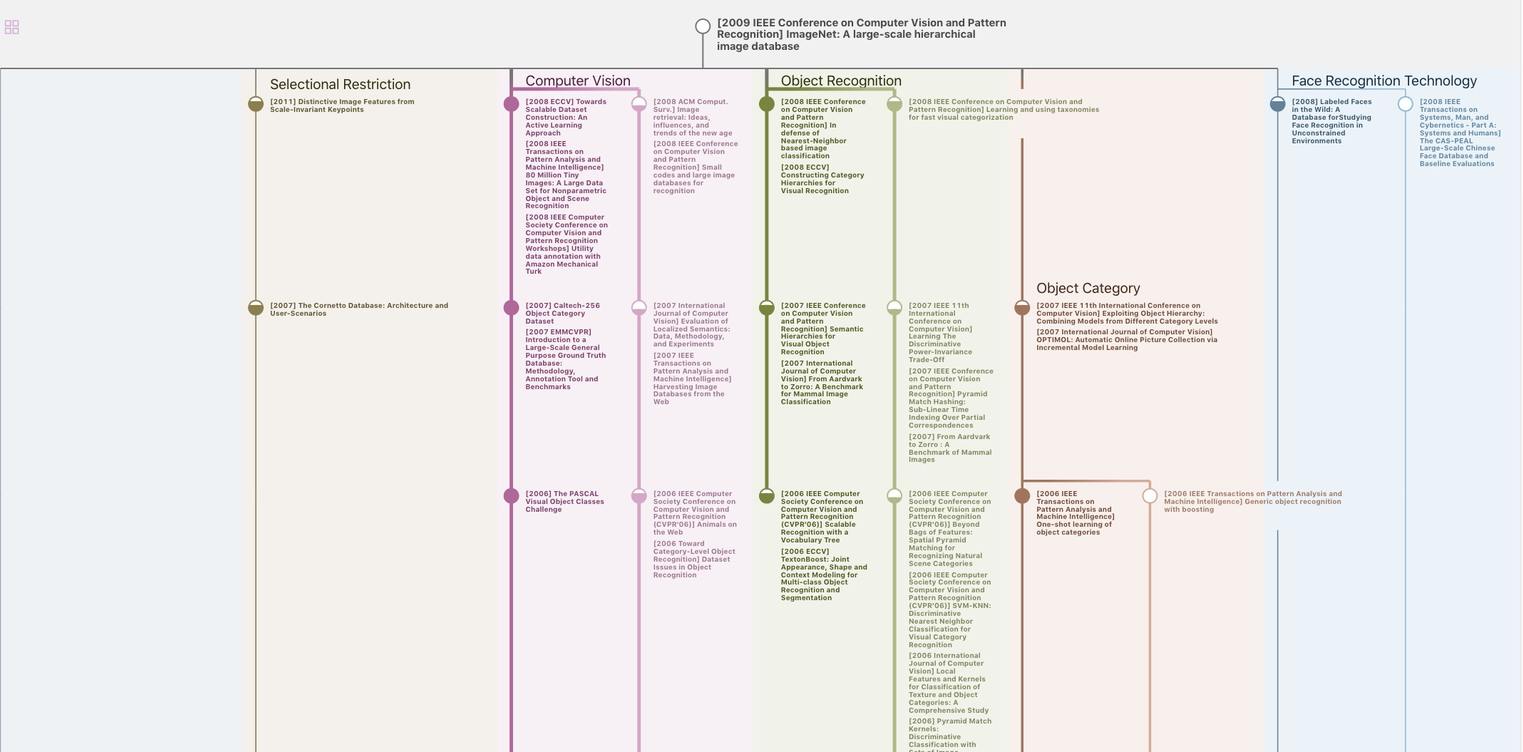
生成溯源树,研究论文发展脉络
Chat Paper
正在生成论文摘要