Temporal predictions of periodic flows using a mesh transformation and deep learning-based strategy
Aerospace Science and Technology(2022)
摘要
This paper focuses on the temporal prediction of unsteady flow based on a combination of mesh transformation and deep learning technology. To this end, a body-fitted mesh interpolation was first implemented to extract the flow information in the region of interest and maintain the detailed flow information in the boundary layer. Subsequently, a mesh transformation technique was applied to map the physical coordinates into the curvilinear coordinates to obtain a structural uniform mesh, which is facilitated for the implementation the structural neural networks. Ultimately, two advanced neural networks, i.e., Unet and Fourier Neural Operator (FNO), were used for establishing the relationship between flow at previous time steps and that at future time steps. Two flow configurations numerically simulated-i.e., a laminar flow around a cylinder at Re = 200 and a transonic buffet of a supercritical airfoil at Ma = 0.73-were selected as the benchmarks to demonstrate the effectiveness and robustness of the proposed strategy. The results indicated that the flow at future time steps can be accurately obtained using that at the previous time steps via both neural networks, even in a complicated flow scenario. The velocity and pressure of the periodic flow can be faithfully predicted with a remarkable accuracy. Additionally, the derived flow physical quantities were comprehensively compared, which demonstrated that better performance can be achieved using FNO with a lower relative loss and a shorter training time-consuming. (c) 2022 Elsevier Masson SAS. All rights reserved.
更多查看译文
关键词
Unsteady flow prediction,Mesh transformation,Deep learning,Data driven
AI 理解论文
溯源树
样例
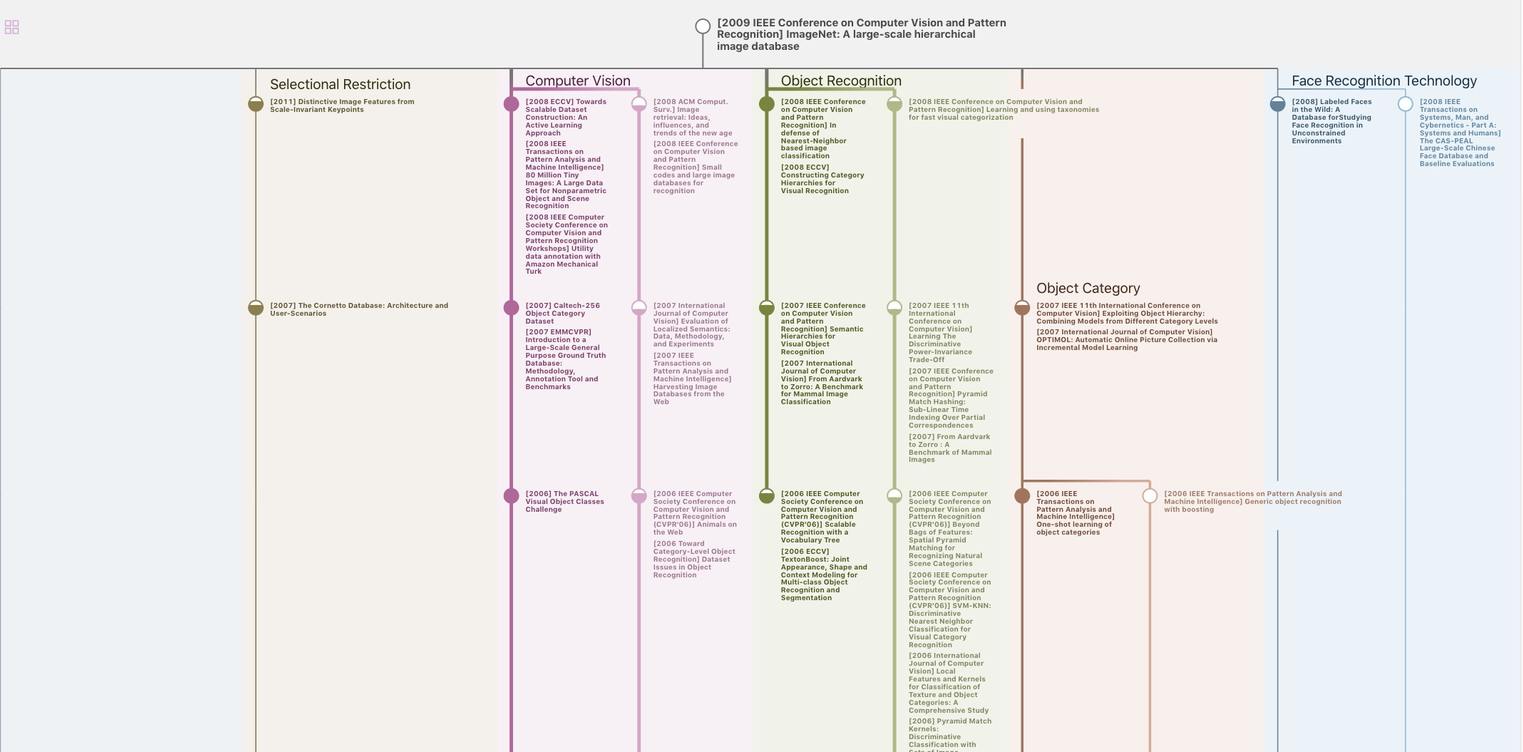
生成溯源树,研究论文发展脉络
Chat Paper
正在生成论文摘要