Combinatorial Coverage for Assured Autonomy
2022 IEEE International Symposium on Software Reliability Engineering Workshops (ISSREW)(2022)
摘要
With the advancement of Artificial Intelligence and Ma-chine Learning (AI/ML), we are observing a rapid increase of autonomous systems in safety-critical domains, such as smart medical equipment, self-driving vehicles, and unmanned aircraft. These systems are required to be made ultra reliable using state of the art verification and validation methodologies. Existing verification, validation, and assurance efforts, such as DO-178C guidance for avionics software, depend on structural coverage based testing, such as MC/DC coverage. Such structural coverage criteria require that test cases are chosen to ensure that a specified level of statements, decisions, and paths are systematically exercised. Neural network and other machine learning based systems, however, are not well suited to be tested with such structural coverage dependent criteria [1], [2]. This is because the performance of machine learning functions such as neural networks depends on the data used to train and test the model, rather than in specifically coded behavior. Behaviors of such systems will change depending on inputs used in the training.
更多查看译文
关键词
Combinatorial Testing,Assured Autonomy
AI 理解论文
溯源树
样例
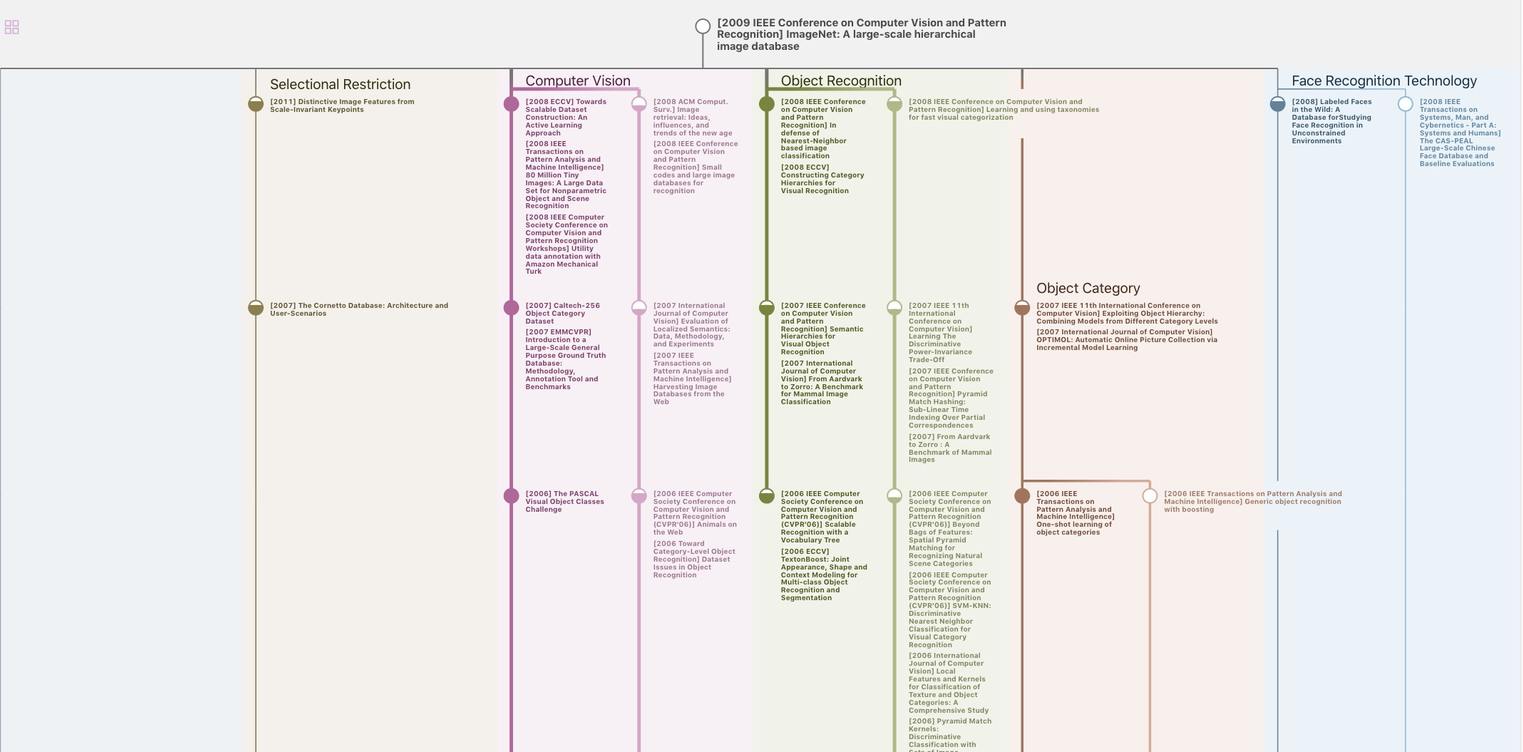
生成溯源树,研究论文发展脉络
Chat Paper
正在生成论文摘要