SummerTime: Variable-length Time Series Summarization with Application to Physical Activity Analysis
ACM Transactions on Computing for Healthcare(2022)
摘要
AbstractSummerTime seeks to summarize global time-series signals and provides a fixed-length, robust representation of the variable-length time series. Many machine learning methods depend on data instances with a fixed number of features. As a result, those methods cannot be directly applied to variable-length time series data. Existing methods such as sliding windows can lose minority local information. Summarization conducted by the SummerTime method will be a fixed-length feature vector which can be used in place of the time series dataset for use with classical machine learning methods. We use Gaussian Mixture models (GMM) over small same-length disjoint windows in the time series to group local data into clusters. The time series’ rate of membership for each cluster will be a feature in the summarization. By making use of variational methods, GMM converges to a more robust mixture, meaning the clusters are more resistant to noise and overfitting. Further, the model is naturally capable of converging to an appropriate cluster count. We validate our method on a challenging real-world dataset, an imbalanced physical activity dataset with a variable-length time series structure. We compare our results to state-of-the-art studies and show high-quality improvement by classifying with only the summarization.
更多查看译文
关键词
physical activity,analysis,series,variable-length
AI 理解论文
溯源树
样例
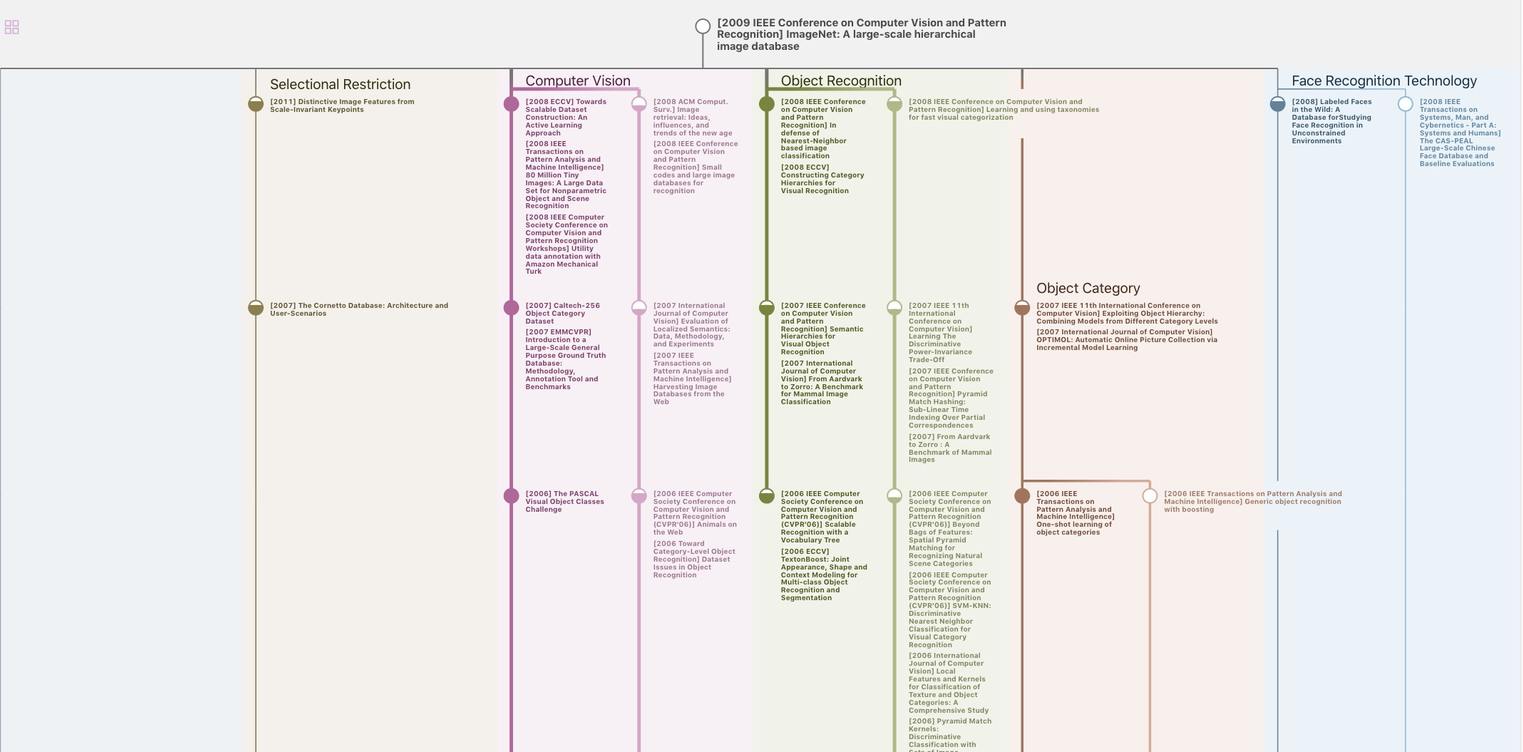
生成溯源树,研究论文发展脉络
Chat Paper
正在生成论文摘要