Serving unseen deep learning models with near-optimal configurations: a fast adaptive search approach
International Conference on Management of Data(2022)
摘要
ABSTRACTPublic clouds provide a bewildering choice of configurations for Deep Learning (DL) models, and the choice of configuration will significantly impact the performance and budget. However, it is an obvious challenge to recommend a near-optimal configuration for a particular DL model from a wide range of candidates. The huge search overhead of finding such a configuration is the notorious cold start problem in state-of-the-art efforts, and this problem becomes more severe when they are faced with unseen DL models. In this paper, we present Falcon, a novel configuration recommender system that can quickly adapt to unseen DL models. Through a large-scale evaluation, we find that there are some Key Operators (KOPs) that can be used to estimate the performance of DL models, and their resource sensitivity can be represented by four typical Key Operator Resource Curves (KOP-RCs). This work can effectively alleviate the cold start problem, because an unseen DL model can be characterized by its KOPs and corresponding KOP-RCs, and this characterization can be constructed as a tree structure in which near-optimal configurations can be searched quickly through a combination of Monte Carlo Tree Search and Bayesian optimization (MCTS-BO). Experiments show that Falcon can effectively reduce the search overhead for unseen DL models by up to 80% compared to state-of-the-art efforts.
更多查看译文
AI 理解论文
溯源树
样例
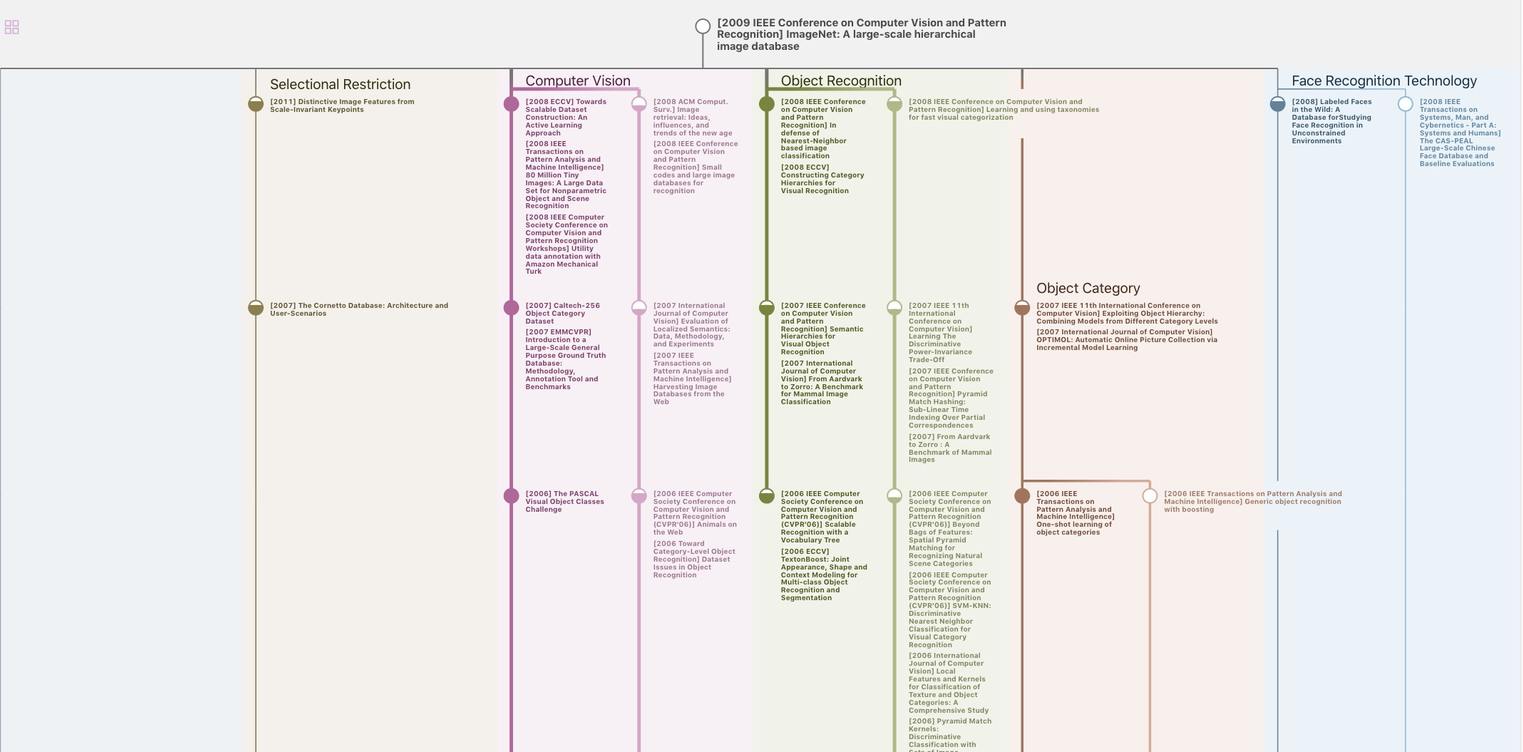
生成溯源树,研究论文发展脉络
Chat Paper
正在生成论文摘要