Tutorial on Deep Learning Interpretation: A Data Perspective
Conference on Information and Knowledge Management(2022)
摘要
ABSTRACTDeep learning models have achieved exceptional predictive performance in a wide variety of tasks, ranging from computer vision, natural language processing, to graph mining. Many businesses and organizations across diverse domains are now building large-scale applications based on deep learning. However, there are growing concerns, regarding the fairness, security, and trustworthiness of these models, largely due to the opaque nature of their decision processes. Recently, there has been an increasing interest in explainable deep learning that aims to reduce the opacity of a model by explaining its behavior, its predictions, or both, thus building trust between human and complex deep learning models. A collection of explanation methods have been proposed in recent years that address the problem of low explainability and opaqueness of models. In this tutorial, we introduce recent explanation methods from a data perspective, targeting models that process image data, text data, and graph data, respectively. We will compare their strengths and limitations, and offer real-world applications.
更多查看译文
关键词
Interpretation, Explainable AI, Deep Learning, Perturbation
AI 理解论文
溯源树
样例
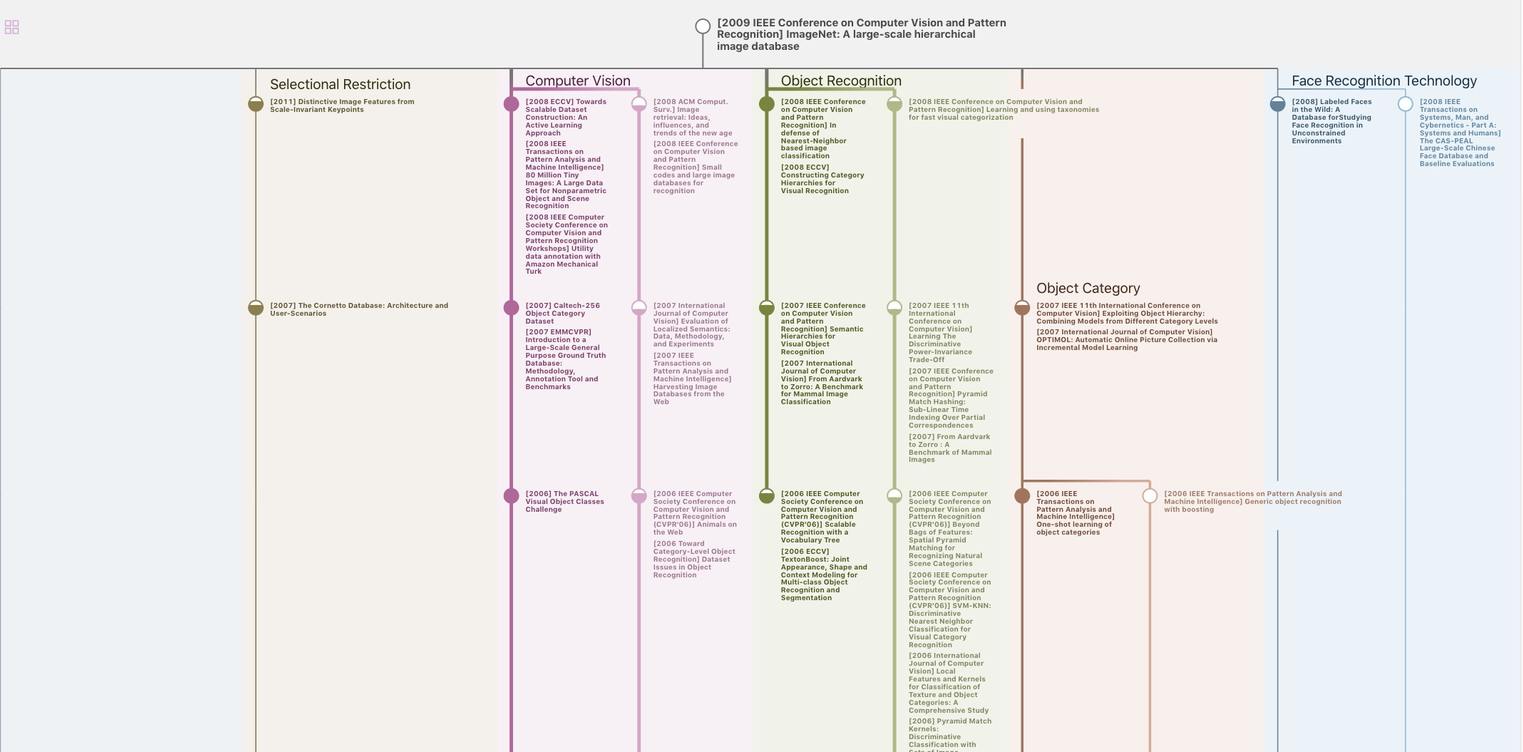
生成溯源树,研究论文发展脉络
Chat Paper
正在生成论文摘要