Query Rewriting in TaoBao Search
PROCEEDINGS OF THE 31ST ACM INTERNATIONAL CONFERENCE ON INFORMATION AND KNOWLEDGE MANAGEMENT, CIKM 2022(2022)
Key words
Query Rewriting,Lexical Match,E-commerce Search
AI Read Science
Must-Reading Tree
Example
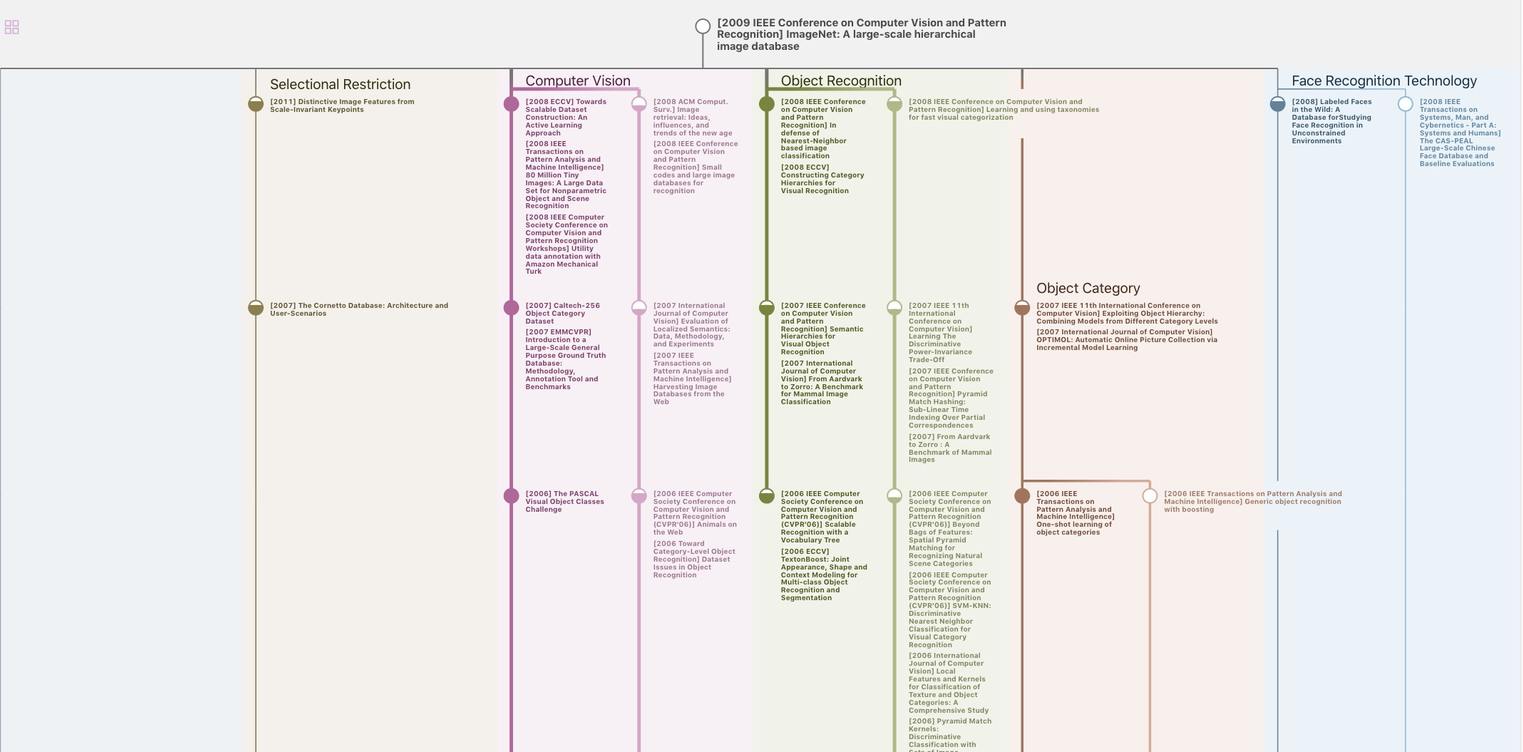
Generate MRT to find the research sequence of this paper
Chat Paper
Summary is being generated by the instructions you defined