Learning-to-Spell: Weak Supervision based Query Correction in E-Commerce Search with Small Strong Labels
Conference on Information and Knowledge Management(2022)
摘要
ABSTRACTFor an E-commerce search engine, users finding the right product critically depend on spell correction. A misspelled query can fetch totally unrelated results which in turn leads to a bad customer experience. Around 32% of queries have spelling mistakes on our e-commerce search engine. The spell problem becomes more challenging when most spell errors arise from customers with little or no exposure to the English language besides the usual source of accidental mistyping on keyboard. These spell errors are heavily influenced by the colloquial and spoken accents of the customers. This limits the benefit from using generic spell correction systems which are learnt from cleaner English sources like Brown Corpus and Wikipedia with a very low focus on phonetic/vernacular spell errors. In this work, we present a novel approach towards spell correction that effectively solves a very diverse set of spell errors and outperforms several state-of-the-art systems in the domain of E-commerce search. Our strategy combines Learning-to-Rank on a small strongly labelled data with multiple learners trained with weakly labelled data. We report the effectiveness of our solution WellSpell (Weak and strong Labels for Learning to Spell) with both the offline evaluations and online A/B experiment.
更多查看译文
关键词
query correction,weak supervision,search,small strong labels,learning-to-spell,e-commerce
AI 理解论文
溯源树
样例
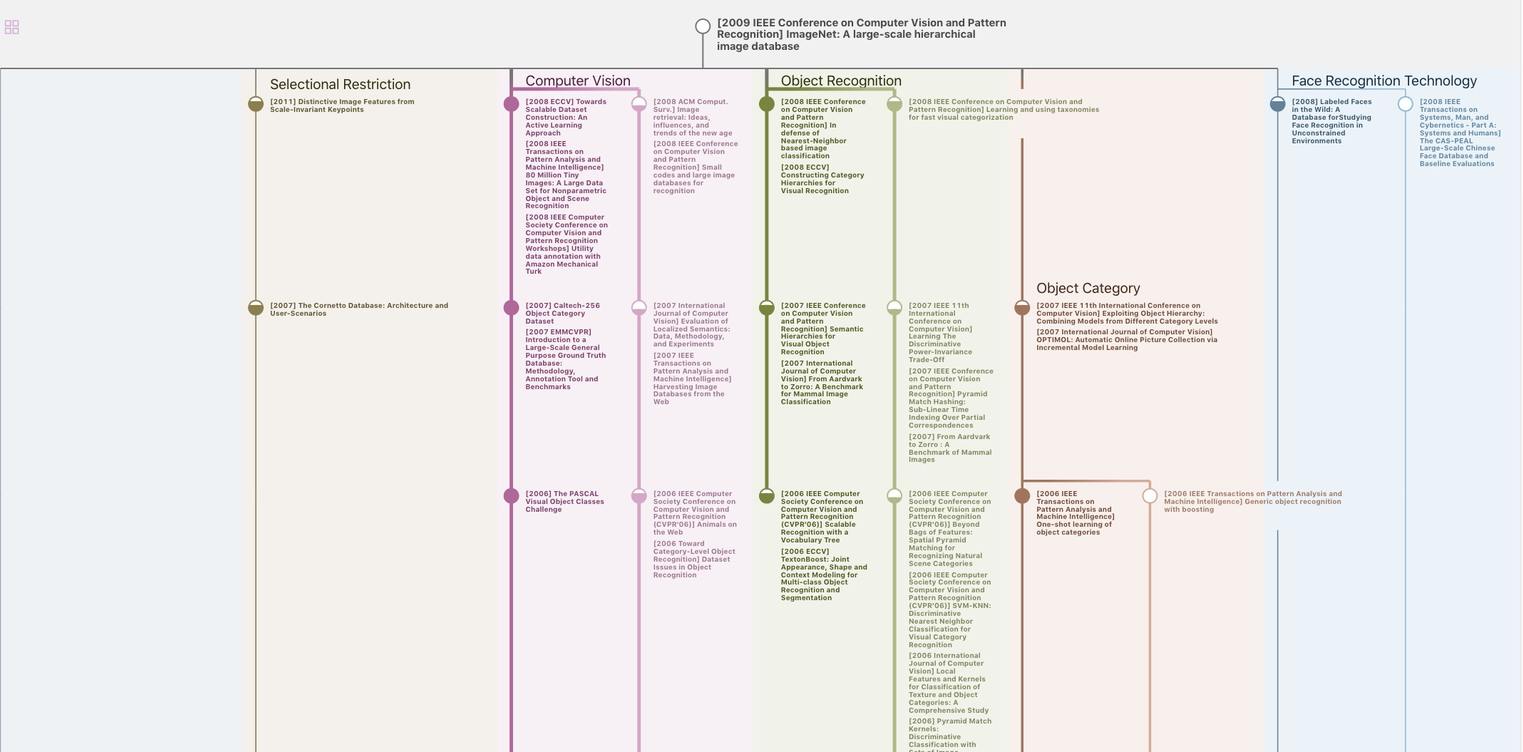
生成溯源树,研究论文发展脉络
Chat Paper
正在生成论文摘要