A molecular classification of gastric cancer associated with distinct clinical outcomes and validated by an XGBoost-based prediction model.
Molecular therapy. Nucleic acids(2023)
摘要
Gastric cancer (GC) is a heterogeneous disease and a leading cause of cancer-related deaths. Discovering robust, clinically relevant molecular classifications is critical for guiding personalized therapies for GC. Here, we propose a refined molecular classification scheme for GC using integrated optimal algorithms and multi-omics data. Based on the important features of mRNA, microRNA, and DNA methylation data selected by the multivariate Cox regression model, three subtypes linked to distinct clinical outcomes were identified by combining similarity network fusion and consensus clustering methods. Three subtypes were validated by an extreme gradient boosting machine learning prediction model with 125 differentially expressed genes in multiple independent cohorts. The molecular characteristics of mutation signatures, characteristic gene sets, driver genes, and chemotherapy sensitivity for each subtype were also identified: subtype 1 was associated with favorable prognosis and characterized by high ARID1A and PIK3CA mutations, subtype 2 was associated with a poor prognosis and harbored high recurrent TP53 mutations, and subtype 3 was associated with high CHD1, APOA1 mutations, and a poor prognosis. The proposed three-subtype scheme achieved a better clinical prediction performance (area under the curve value = 0.71) than The Cancer Genome Atlas classification, which may provide a practical subtyping framework to improve the treatment of GC.
更多查看译文
关键词
MT: Bioinformatics,gastric cancer,molecular classification,precision oncology,prediction model,prognostic marker
AI 理解论文
溯源树
样例
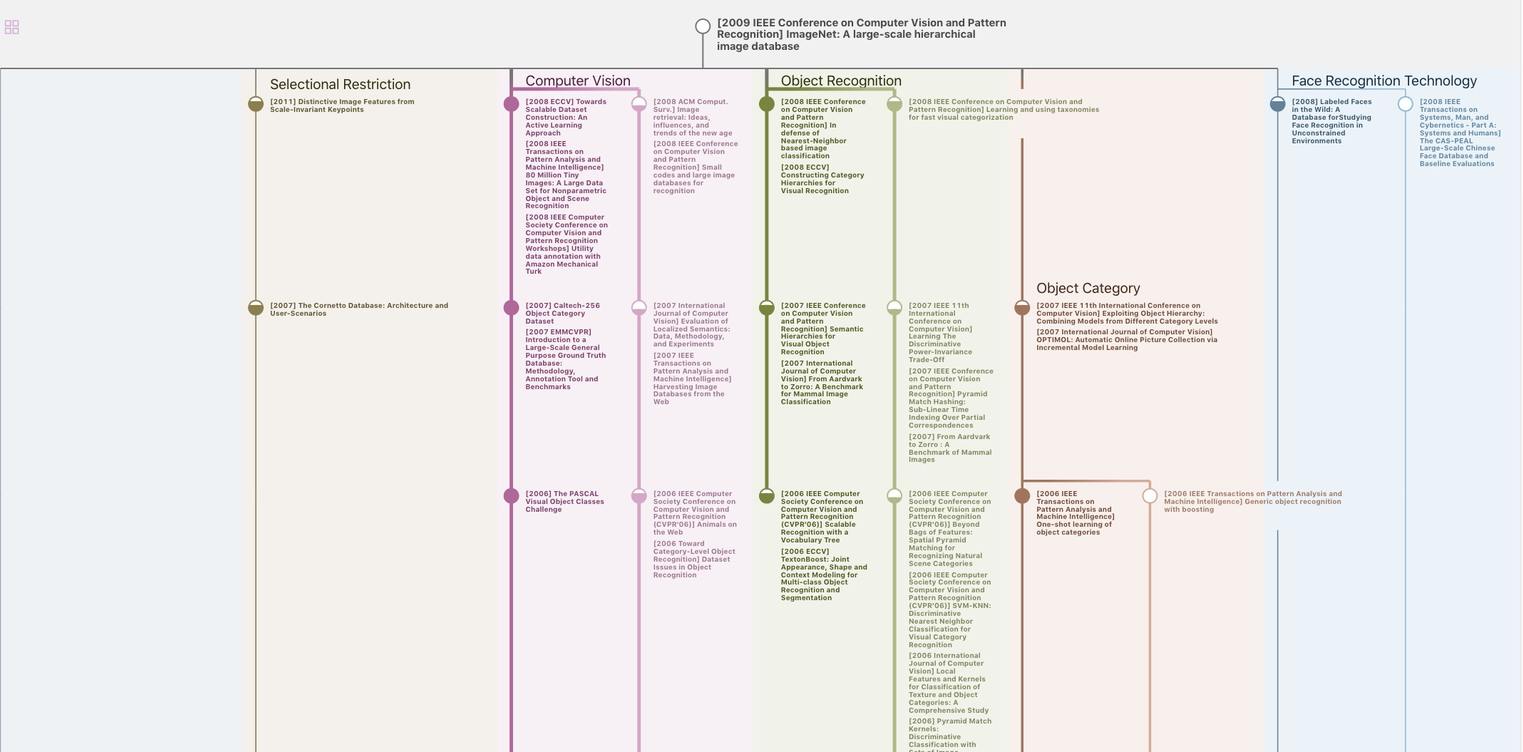
生成溯源树,研究论文发展脉络
Chat Paper
正在生成论文摘要