EmbedFormer: Embedded Depth-Wise Convolution Layer for Token Mixing.
Sensors(2022)
摘要
Visual Transformers (ViTs) have shown impressive performance due to their powerful coding ability to catch spatial and channel information. MetaFormer gives us a general architecture of transformers consisting of a token mixer and a channel mixer through which we can generally understand how transformers work. It is proved that the general architecture of the ViTs is more essential to the models' performance than self-attention mechanism. Then, Depth-wise Convolution layer (DwConv) is widely accepted to replace local self-attention in transformers. In this work, a pure convolutional "transformer" is designed. We rethink the difference between the operation of self-attention and DwConv. It is found that the self-attention layer, with an embedding layer, unavoidably affects channel information, while DwConv only mixes the token information per channel. To address the differences between DwConv and self-attention, we implement DwConv with an embedding layer before as the token mixer to instantiate a MetaFormer block and a model named EmbedFormer is introduced. Meanwhile, SEBlock is applied in the channel mixer part to improve performance. On the ImageNet-1K classification task, EmbedFormer achieves top-1 accuracy of 81.7% without additional training images, surpassing the Swin transformer by +0.4% in similar complexity. In addition, EmbedFormer is evaluated in downstream tasks and the results are entirely above those of PoolFormer, ResNet and DeiT. Compared with PoolFormer-S24, another instance of MetaFormer, our EmbedFormer improves the score by +3.0% box AP/+2.3% mask AP on the COCO dataset and +1.3% mIoU on the ADE20K.
更多查看译文
关键词
CNN,computer vision,deep learning,vision transformer
AI 理解论文
溯源树
样例
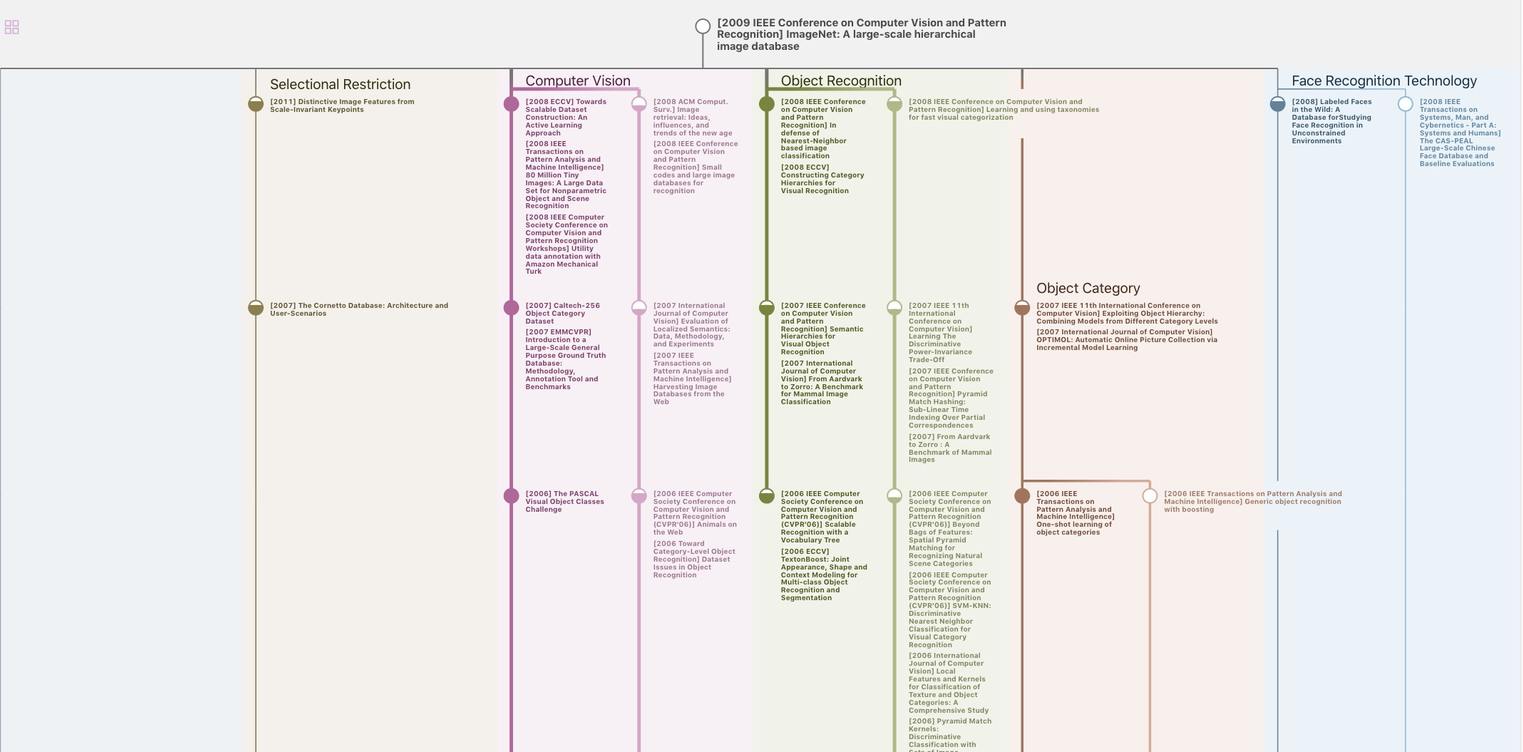
生成溯源树,研究论文发展脉络
Chat Paper
正在生成论文摘要