Curriculum Reinforcement Learning Based on K-Fold Cross Validation.
Entropy(2022)
摘要
With the continuous development of deep reinforcement learning in intelligent control, combining automatic curriculum learning and deep reinforcement learning can improve the training performance and efficiency of algorithms from easy to difficult. Most existing automatic curriculum learning algorithms perform curriculum ranking through expert experience and a single network, which has the problems of difficult curriculum task ranking and slow convergence speed. In this paper, we propose a curriculum reinforcement learning method based on K-Fold Cross Validation that can estimate the relativity score of task curriculum difficulty. Drawing lessons from the human concept of curriculum learning from easy to difficult, this method divides automatic curriculum learning into a curriculum difficulty assessment stage and a curriculum sorting stage. Through parallel training of the teacher model and cross-evaluation of task sample difficulty, the method can better sequence curriculum learning tasks. Finally, simulation comparison experiments were carried out in two types of multi-agent experimental environments. The experimental results show that the automatic curriculum learning method based on K-Fold cross-validation can improve the training speed of the MADDPG algorithm, and at the same time has a certain generality for multi-agent deep reinforcement learning algorithm based on the replay buffer mechanism.
更多查看译文
关键词
K-fold cross validation,automatic curriculum learning,deep reinforcement learning,replay buffer
AI 理解论文
溯源树
样例
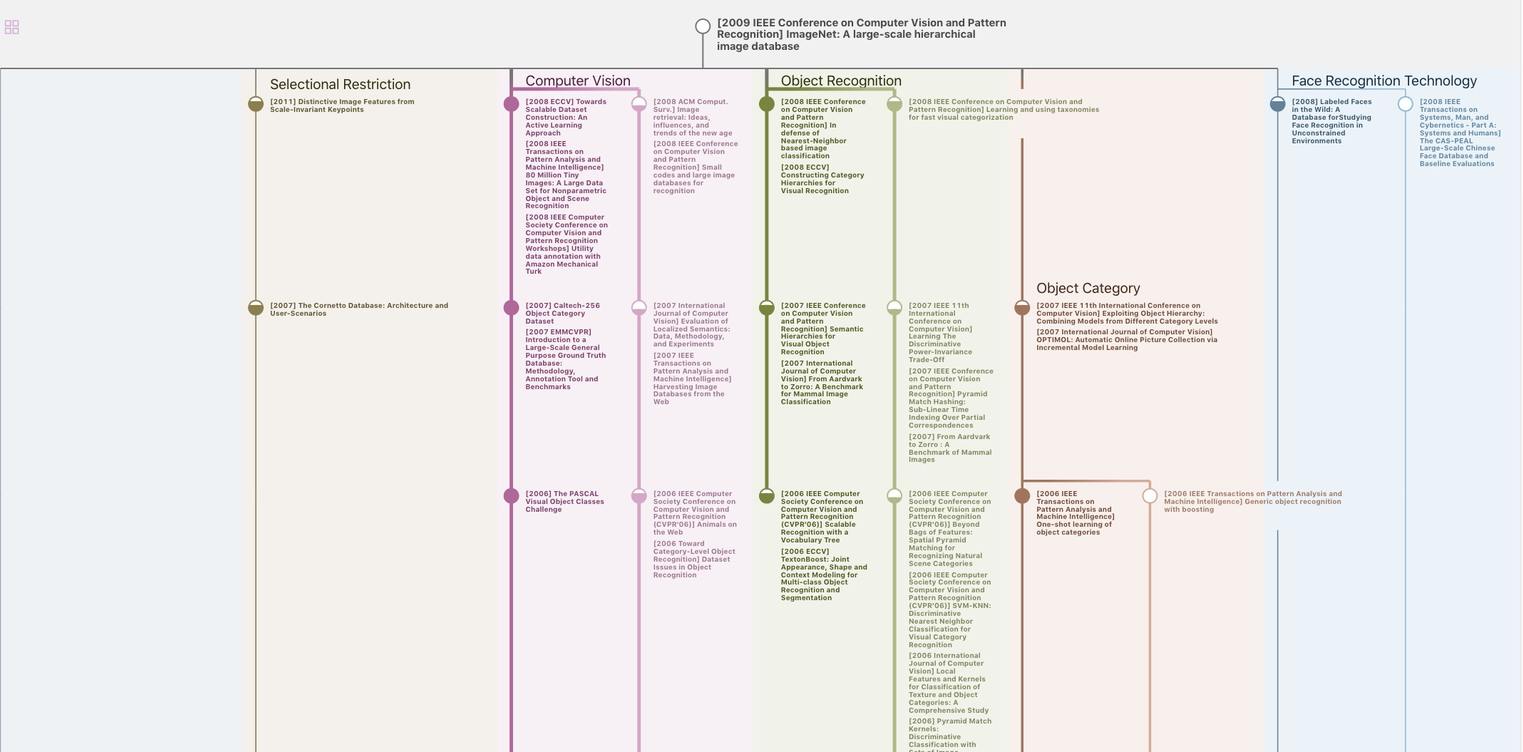
生成溯源树,研究论文发展脉络
Chat Paper
正在生成论文摘要