MoQuad: Motion-focused Quadruple Construction for Video Contrastive Learning
arxiv(2022)
摘要
Learning effective motion features is an essential pursuit of video representation learning. This paper presents a simple yet effective sample construction strategy to boost the learning of motion features in video contrastive learning. The proposed method, dubbed Motion-focused Quadruple Construction (MoQuad), augments the instance discrimination by meticulously disturbing the appearance and motion of both the positive and negative samples to create a quadruple for each video instance, such that the model is encouraged to exploit motion information. Unlike recent approaches that create extra auxiliary tasks for learning motion features or apply explicit temporal modelling, our method keeps the simple and clean contrastive learning paradigm (i.e.,SimCLR) without multi-task learning or extra modelling. In addition, we design two extra training strategies by analyzing initial MoQuad experiments. By simply applying MoQuad to SimCLR, extensive experiments show that we achieve superior performance on downstream tasks compared to the state of the arts. Notably, on the UCF-101 action recognition task, we achieve 93.7% accuracy after pre-training the model on Kinetics-400 for only 200 epochs, surpassing various previous methods
更多查看译文
关键词
video contrastive
AI 理解论文
溯源树
样例
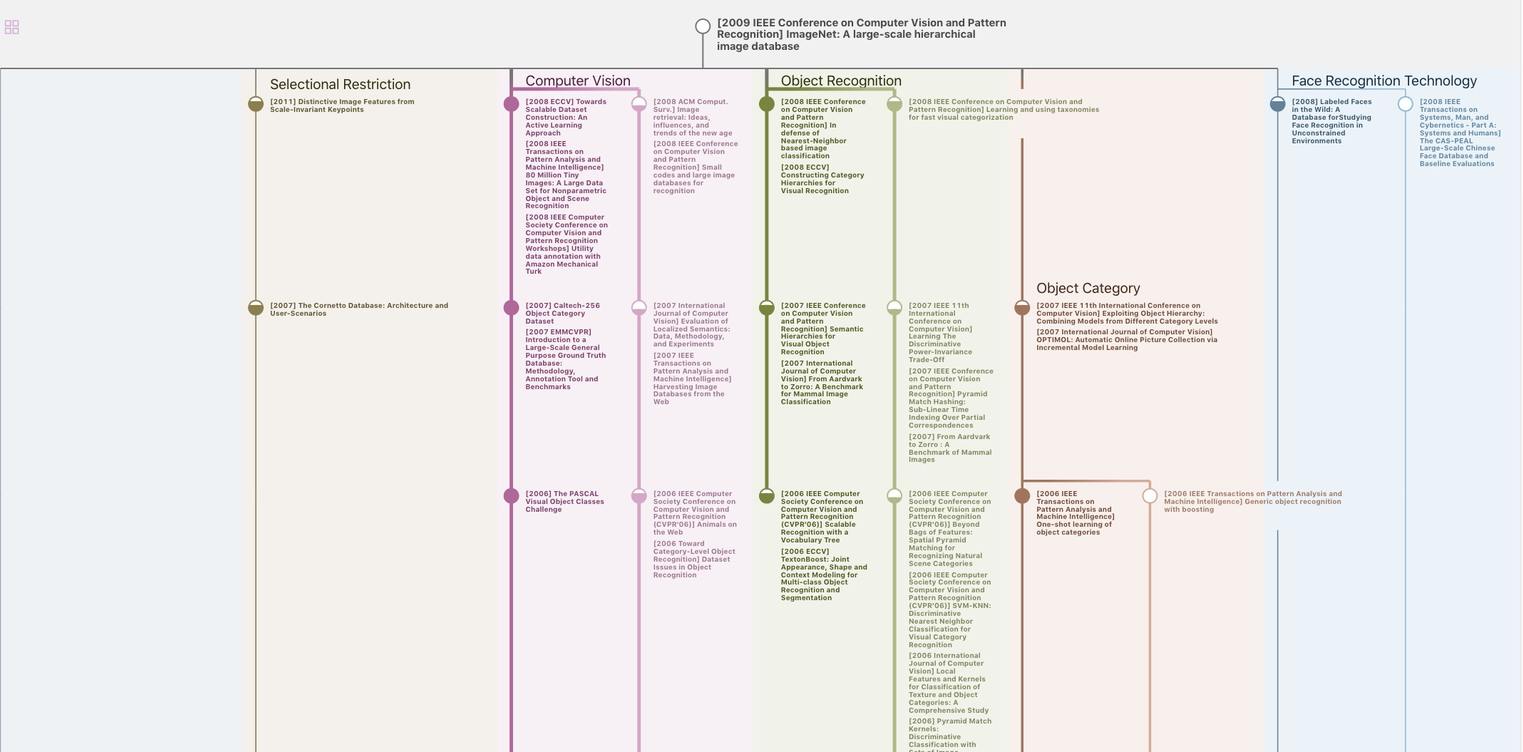
生成溯源树,研究论文发展脉络
Chat Paper
正在生成论文摘要