Random Linear Estimation With Rotationally-Invariant Designs: Asymptotics at High Temperature
IEEE TRANSACTIONS ON INFORMATION THEORY(2024)
Abstract
We study estimation in the linear model y = A beta* + epsilon , in a Bayesian setting where beta* has an entrywise i.i.d. prior and the design A is rotationally-invariant in law. In the large system limit as dimension and sample size increase proportionally, a set of related conjectures have been postulated for the asymptotic mutual information, Bayes-optimal mean squared error, and TAP mean-field equations that characterize the Bayes posterior mean of beta*. In this work, we prove these conjectures for a general class of signal priors and for arbitrary rotationally-invariant designs A, under a "high-temperature" condition that restricts the range of eigenvalues of A(T) A and encompasses regimes of sufficiently low signal-to-noise ratio. Our proof uses a conditional second-moment method argument, where we condition on the iterates of a version of the Vector AMP algorithm for solving the TAP mean-field equations.
MoreTranslated text
Key words
Estimation,Bayes methods,multiaccess communication,mutual information
AI Read Science
Must-Reading Tree
Example
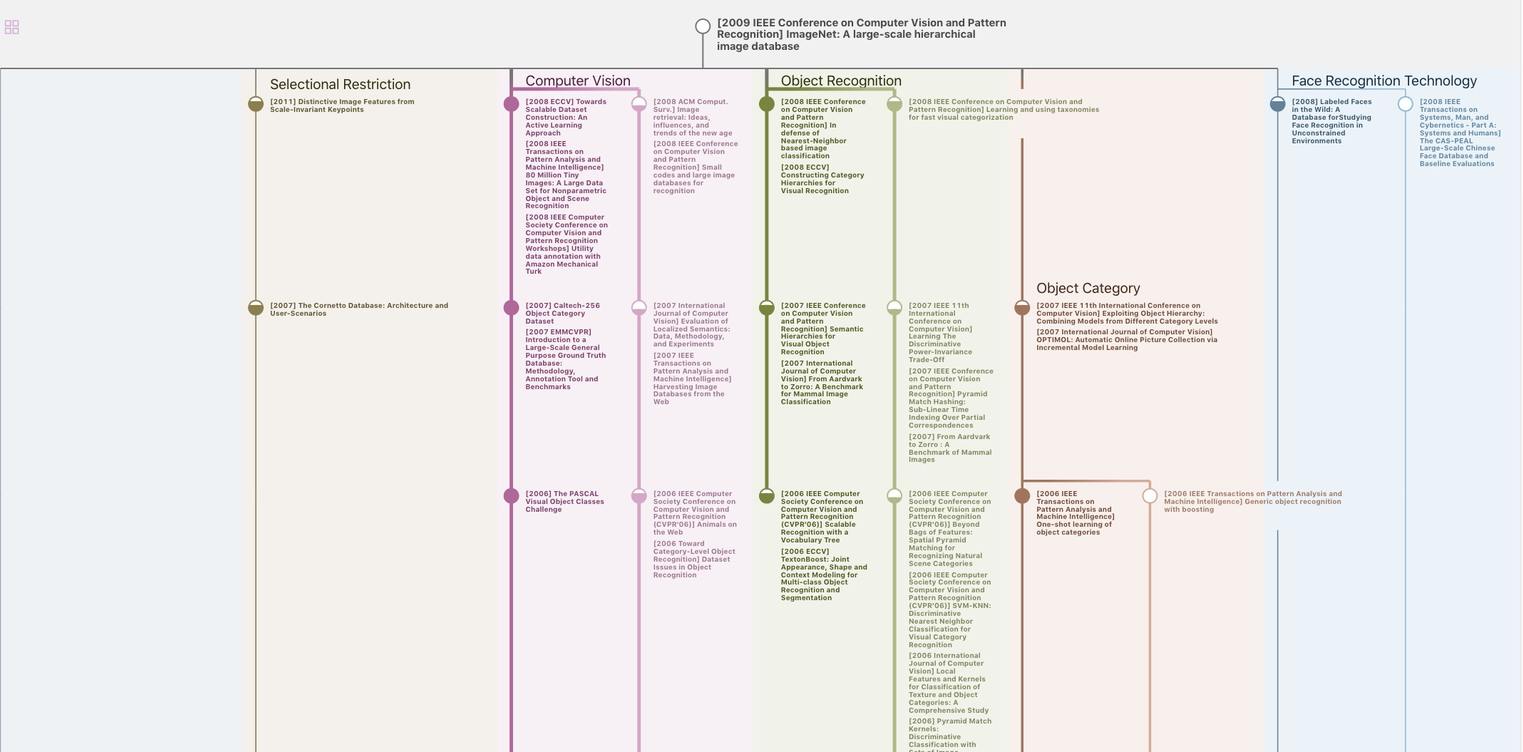
Generate MRT to find the research sequence of this paper
Chat Paper
Summary is being generated by the instructions you defined