Active Testing for an Emerging Epidemic.
2022 IEEE International Conference on E-health Networking, Application & Services (HealthCom)(2022)
摘要
Identifying disease carriers is a key barrier to effectively control an epidemic outbreak, especially when many carriers are asymptomatic, have minor symptoms, or have a delayed symptom onset. Current isolation policies largely operate at the two ends of the spectrum: isolate almost everyone (lock-down) or isolate only those with severe symptoms. This leads to high misclassification costs. To address this issue, we develop an active learning approach. Active learning is useful when labeling is expensive and there is a limited budget; an active learning algorithm selects which data points to label in order to build the best training dataset for machine learning. We present the novel Active Testing protocol to combine 1) an online, disease-carrier classification model trained on symptom data paired with 2) an active learning based disease testing policy, that results in lower misclassification costs than either of the two extreme isolation policies. Coupling these two components enables our protocol to pick the best testing kit allocation policy to train the carrier classification model and minimize the total decision-theoretic, isolation misclassification cost. We accomplish this with a novel, cost-aware active learning algorithm, and demonstrate its effectiveness compared to existing algorithms in the class-imbalanced setting of disease-carrier classification.
更多查看译文
AI 理解论文
溯源树
样例
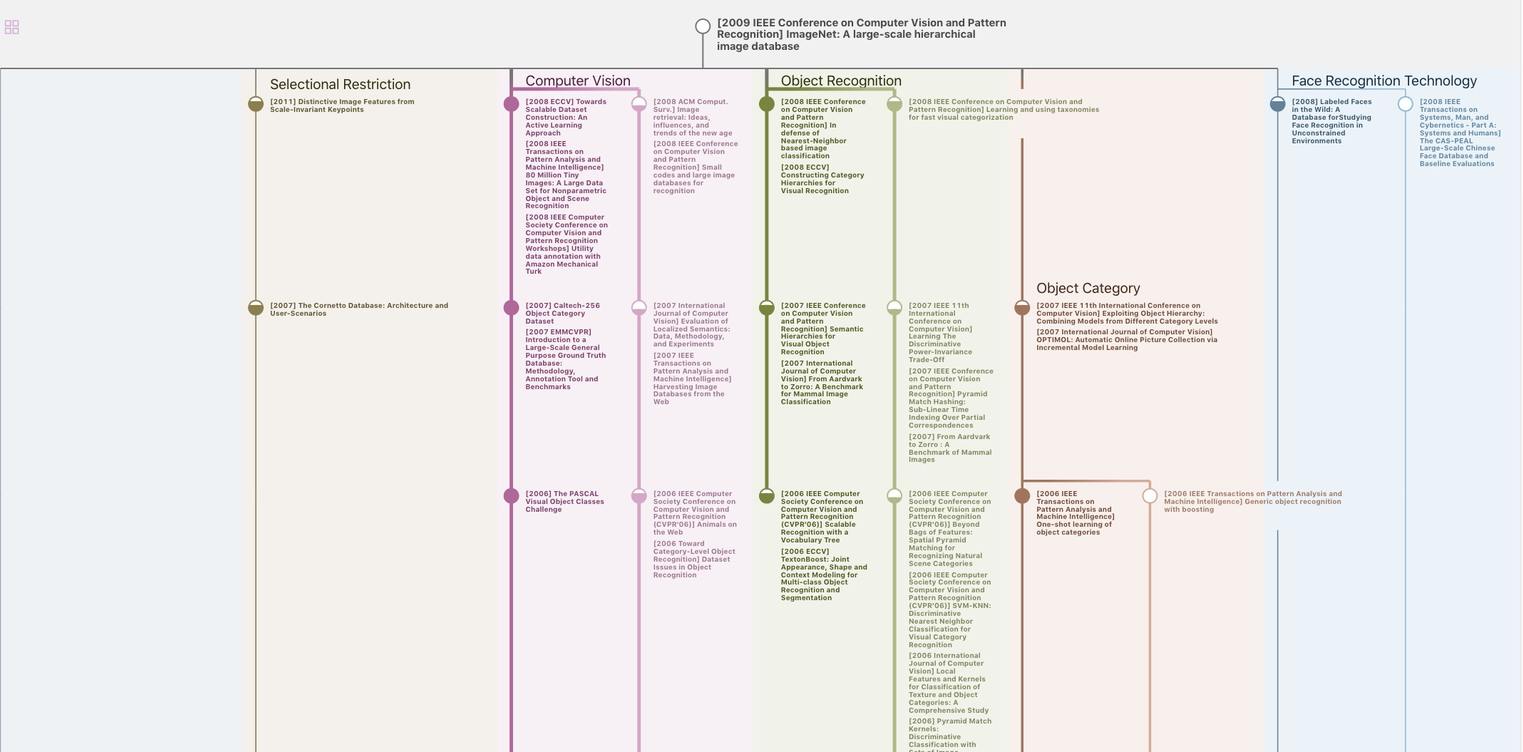
生成溯源树,研究论文发展脉络
Chat Paper
正在生成论文摘要