Multi-level collaborative short-term load forecasting
2022 25th International Conference on Electrical Machines and Systems (ICEMS)(2022)
摘要
Considering that the campus electric load presents diversity, the accuracy and rapidity of load prediction will directly affect the safety and economy of the electric power system. In order to better explore the effective information contained in the electric load data, improve the accuracy of short-term load prediction, and analyze the campus electricity consumption pattern, this paper proposes a multi-level collaborative short-term load prediction model. The improved PCAG-KM algorithm is proposed for the problems of determining the optimal cluster set number k and selecting the initial clustering center for the traditional K-Means clustering algorithm. To improve the accuracy of the short-term load forecasting model, a combined forecasting method (VMDsnap-LSTM) based on the improved variational modal technique with a snapshot feedback mechanism combined with a long and short term memory network is proposed. Experimental results show the effectiveness of the combined model. The paper uses the actual station load of the university for arithmetic testing, and the experimental results show the effectiveness of this model..
更多查看译文
关键词
load,multi-level,short-term
AI 理解论文
溯源树
样例
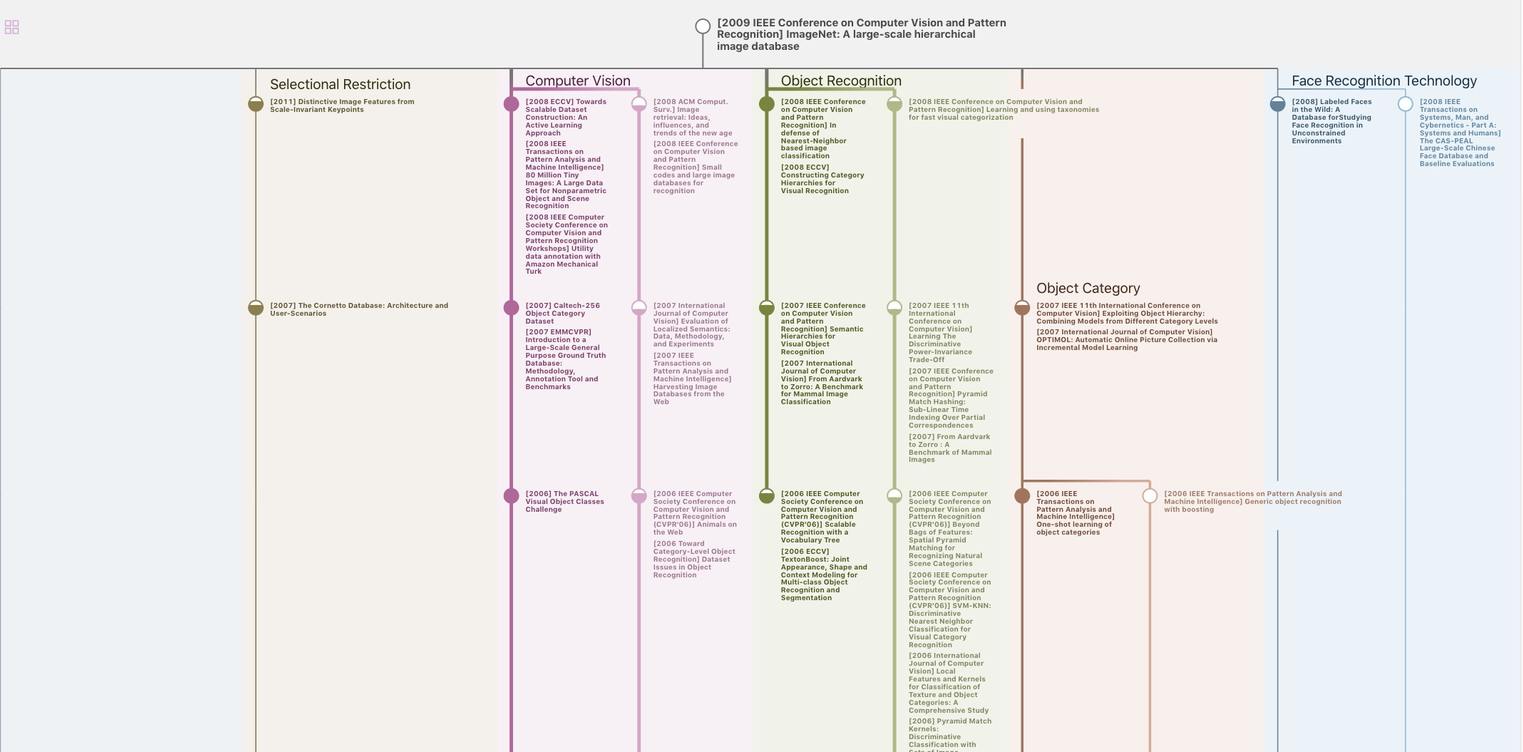
生成溯源树,研究论文发展脉络
Chat Paper
正在生成论文摘要