LRDNet: Lightweight LiDAR Aided Cascaded Feature Pools for Free Road Space Detection
IEEE Transactions on Multimedia(2022)
摘要
Humans have long fantasized about self-driving vehicles for the sake of luxury, style, safety, and ease. Free road space detection for collision avoidance and path planning is a vital part of autonomous driving vehicles. Despite many researchers focusing on free road space detection, it remains an open and challenging problem for real-world applications. Many studies have attempted to fuse depth and LiDAR features with visual features to improve the overall performance of free road space detection. However, there is no guideline on how such features should be fused to complement the visual features. Additionally, most of the previously proposed methods are computationally expensive and not suitable for real-life applications. The main motivation of this study is to realize a lightweight model that addresses these problems without compromising performance. As the LiDAR and visual features exist in different spaces, the proposed method attempts to learn various transformation and fusion operations from LiDAR features to complement the visual features. To validate the performance of the proposed method, we conduct comprehensive experiments on prominent benchmark datasets. The results of the experiments reveal the superior performance of the proposed model while being lightweight. LRDNet ranks third overall (with a minor difference) and second among LiDAR-based methods on the KITTI road benchmark dataset. Furthermore, the proposed model is the least computationally expensive among state-of-the-art methods and can be considered an optimal trade-off between speed and accuracy.
更多查看译文
关键词
Road detection,free road space,feature pools,road segmentation,LiDAR,KITTI road
AI 理解论文
溯源树
样例
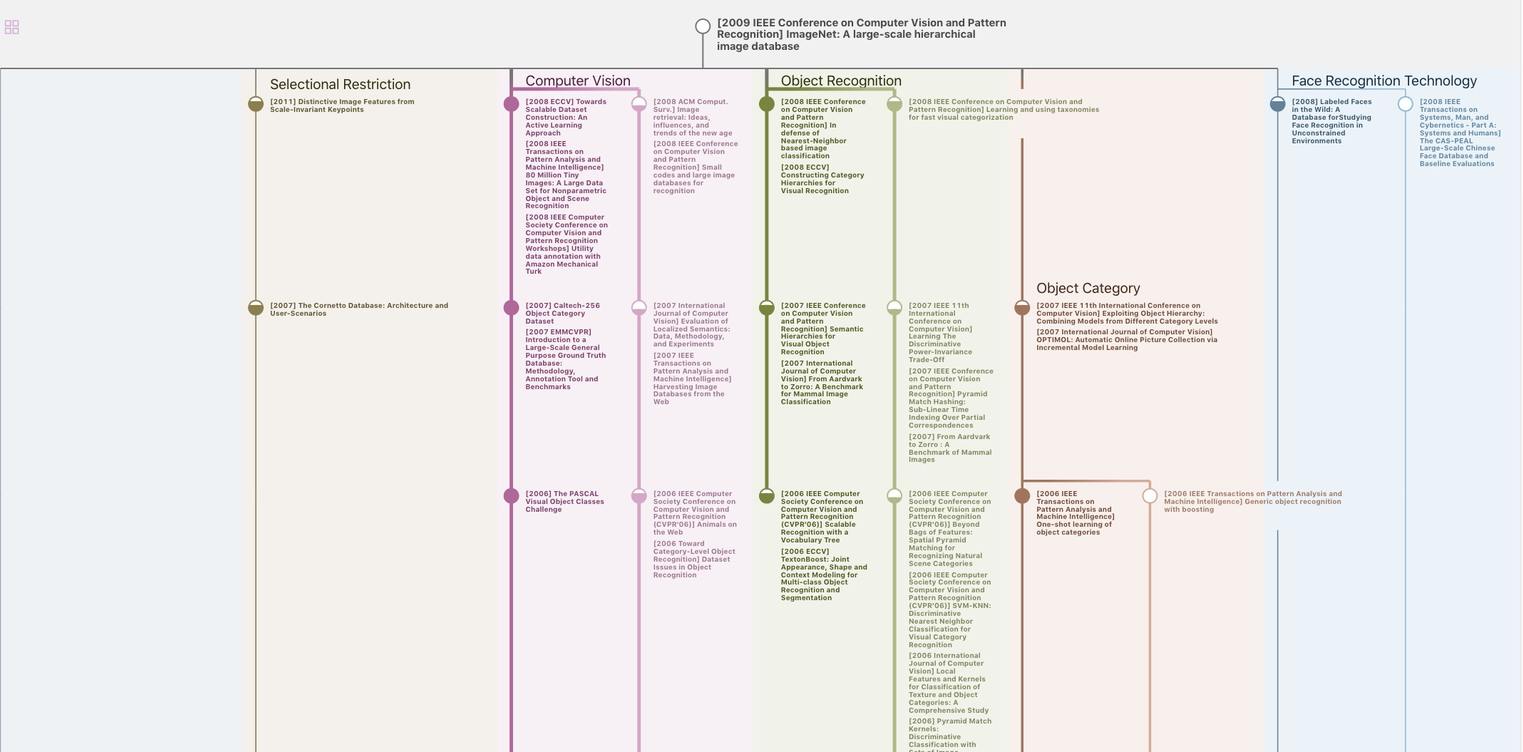
生成溯源树,研究论文发展脉络
Chat Paper
正在生成论文摘要