Incremental Low-Rank Dynamic Mode Decomposition Model for Efficient Forecast Dissemination and Onboard Forecasting
OCEANS 2022, Hampton Roads(2022)
摘要
Onboard forecasting is challenging but essential for unmanned autonomous ocean platforms. Due to the numerous operational constraints of these platforms, efficient adaptive Reduced-Order Models (ROMs) are needed. In this work, we employ the incremental Low-Rank Dynamic Mode Decomposition (iLRDMD), which is an adaptive, data-driven, DMD-based ROM that enables efficient forecast compression, transmission, and onboard forecasting. We demonstrate the algorithm on 3D multivariate Hybrid Coordinate Ocean Model (HYCOM) ocean fields in the Middle Atlantic Ridge (MAR) region. We further demonstrate that these iLRDMD ocean forecasts can be used for interdisciplinary applications such as underwater acoustics predictions. Here, acoustics fields computed from the ocean iLRDMD forecasts are compared to those computed from HYCOM fields. We also illustrate the application of a joint ocean-acoustics iLRDMD model for predetermined acoustics configurations. In the MAR region, we find that iLRDMD models are sufficiently accurate and efficient for onboard ocean and acoustic forecasting of temperature, salinity, velocity, and transmission loss fields.
更多查看译文
关键词
reduced-order model,Dynamic Mode Decomposition,forecast dissemination,communication,AUVs,autonomy,underwater acoustics
AI 理解论文
溯源树
样例
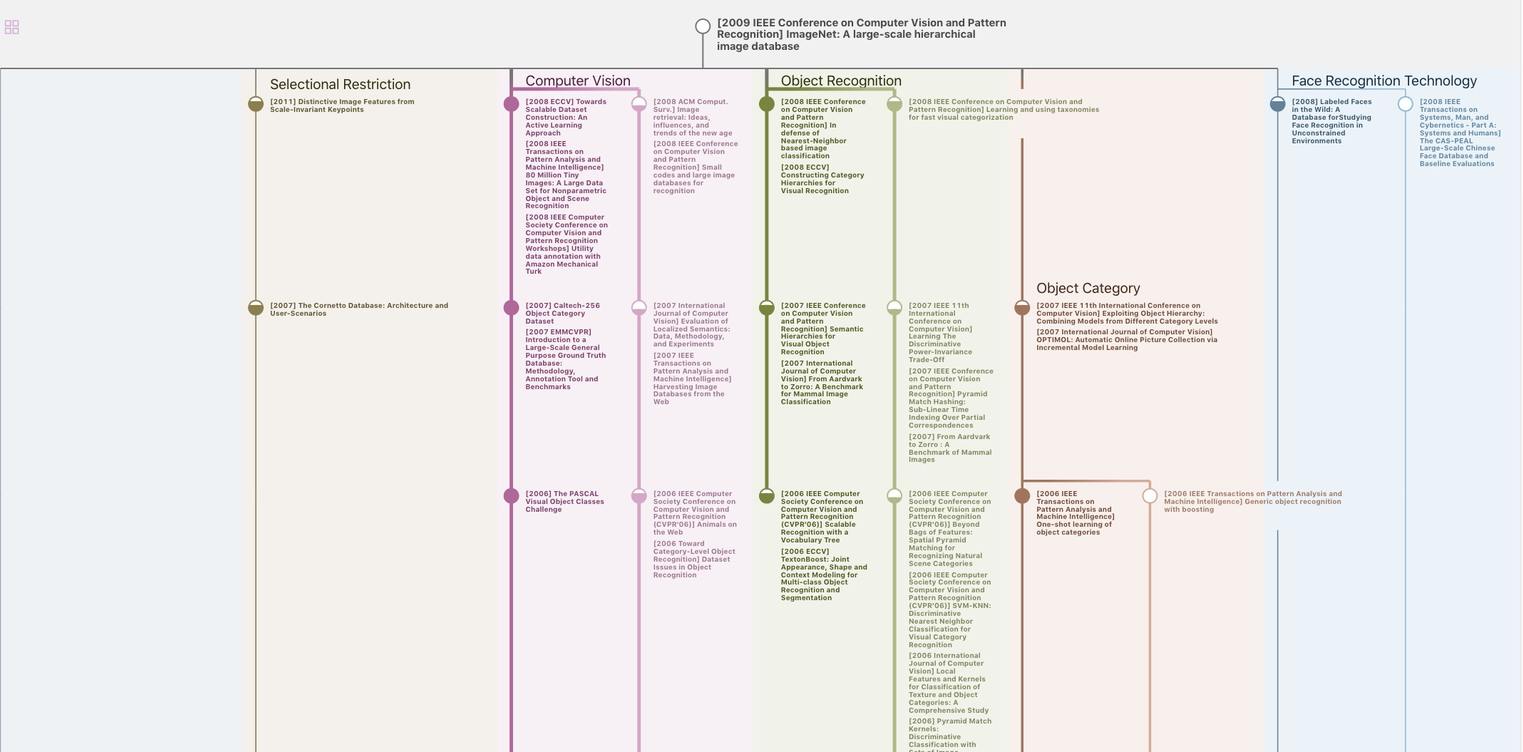
生成溯源树,研究论文发展脉络
Chat Paper
正在生成论文摘要