Cross-Domain Character Recognition through Latent Space Alignment.
ICBDT(2022)
摘要
Deep neural networks have proved its capability in many machine learning tasks. The effectiveness of deep neural networks in real-world applications, however, is greatly affected by the distribution discrepancy between the training and testing data. To address the issue, domain adaptation methods have been studied. In this work, we propose a novel unsupervised domain adaptation method which combines the feature learning and the distribution estimation into one learning framework, enabling automatic update of feature representations through fine-tuning parameterized distributions. As such, our model can produce an unified distribution to represent both source and target samples. Furthermore, two new regularizers are integrated into the optimization objective to minimize the divergence of the unified distribution from those of source and target domains. Experiments on character reconstruction show that our method demonstrates much better learning ability compared to the existing variational autoencoder. More importantly, our method improves recognition accuracy by more than 5% from that of the state-of-the-art methods in domain adaptation tasks built upon popular character datasets.
更多查看译文
AI 理解论文
溯源树
样例
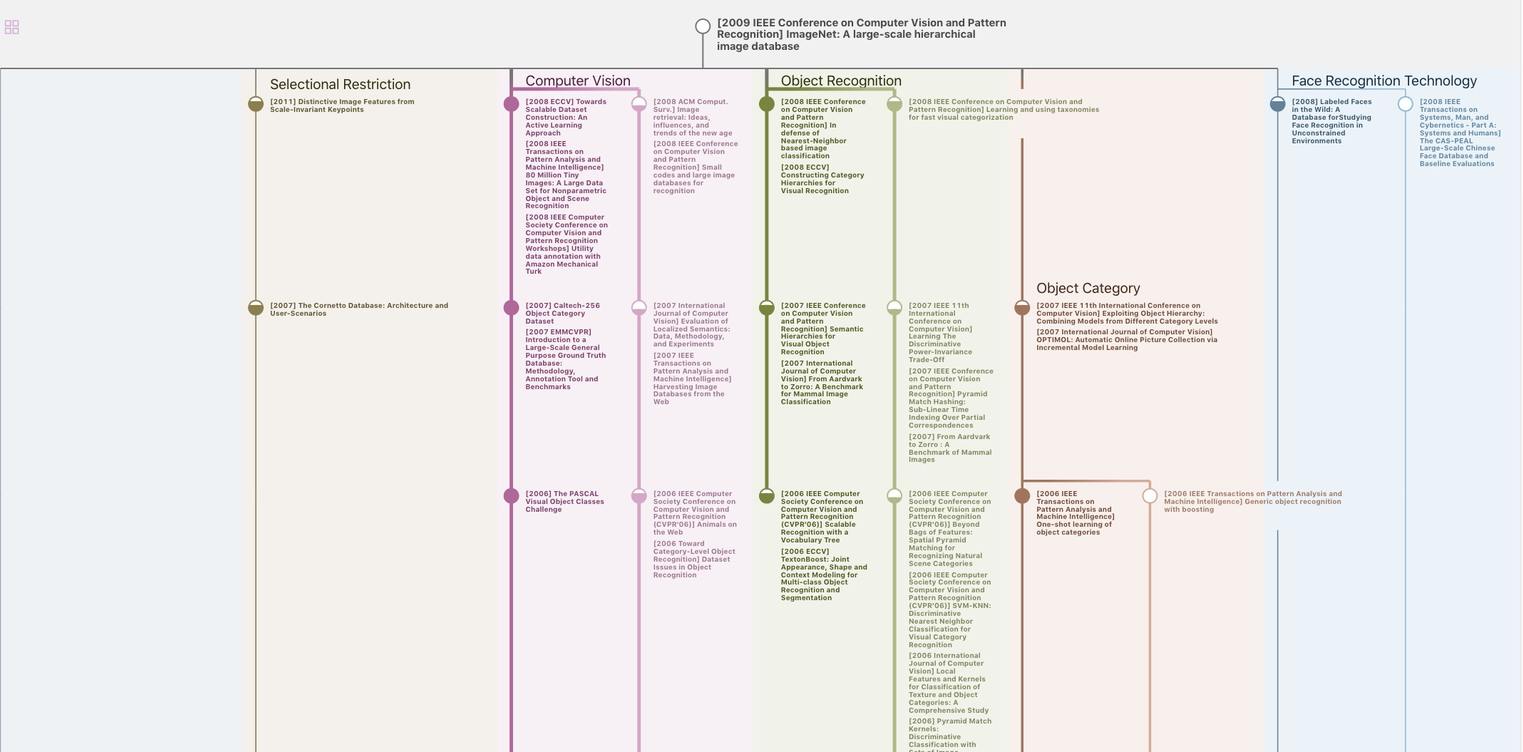
生成溯源树,研究论文发展脉络
Chat Paper
正在生成论文摘要