Machine learning accelerated design of non-equiatomic refractory high entropy alloys based on first principles calculation
Vacuum(2023)
摘要
The properties of High Entropy Alloys (HEAs) strongly depend on the composition and content of elements. However, it was difficult to obtain the optimized element composition through the traditional "trial and error" method. The non-equiatomic HEAs have a large range for composition exploration by changing the content of elements, but the current research methods are difficult to analyze comprehensively. In this work, the prediction model with high accuracy is established by mixture design, the first principles calculation and machine learning. The model is used to predict the elastic properties and Poisson's ratio of non-equiatomic Mo–Nb–Ta–Ti–V HEAs, and the prediction results agree well with experimental data. The optimal element composition range of elastic properties and Poisson's ratio could be obtained. The influence of elements on the elastic properties and Poisson's ratio is analyzed through the calculation of features' importance. The results show that the content of Ti has the greatest contribution to the elastic properties and Poisson's ratio of the alloy. This model can not only obtain a large amount of data quickly and accurately but also help us to establish the relationship between element content and mechanical properties of non-equiatomic Mo–Nb–Ta–Ti–V RHEAs and provide theoretical guidance for experiments.
更多查看译文
关键词
Non-equiatomic refractory high entropy alloys,The first-principles calculation,Machine learning,Elastic properties
AI 理解论文
溯源树
样例
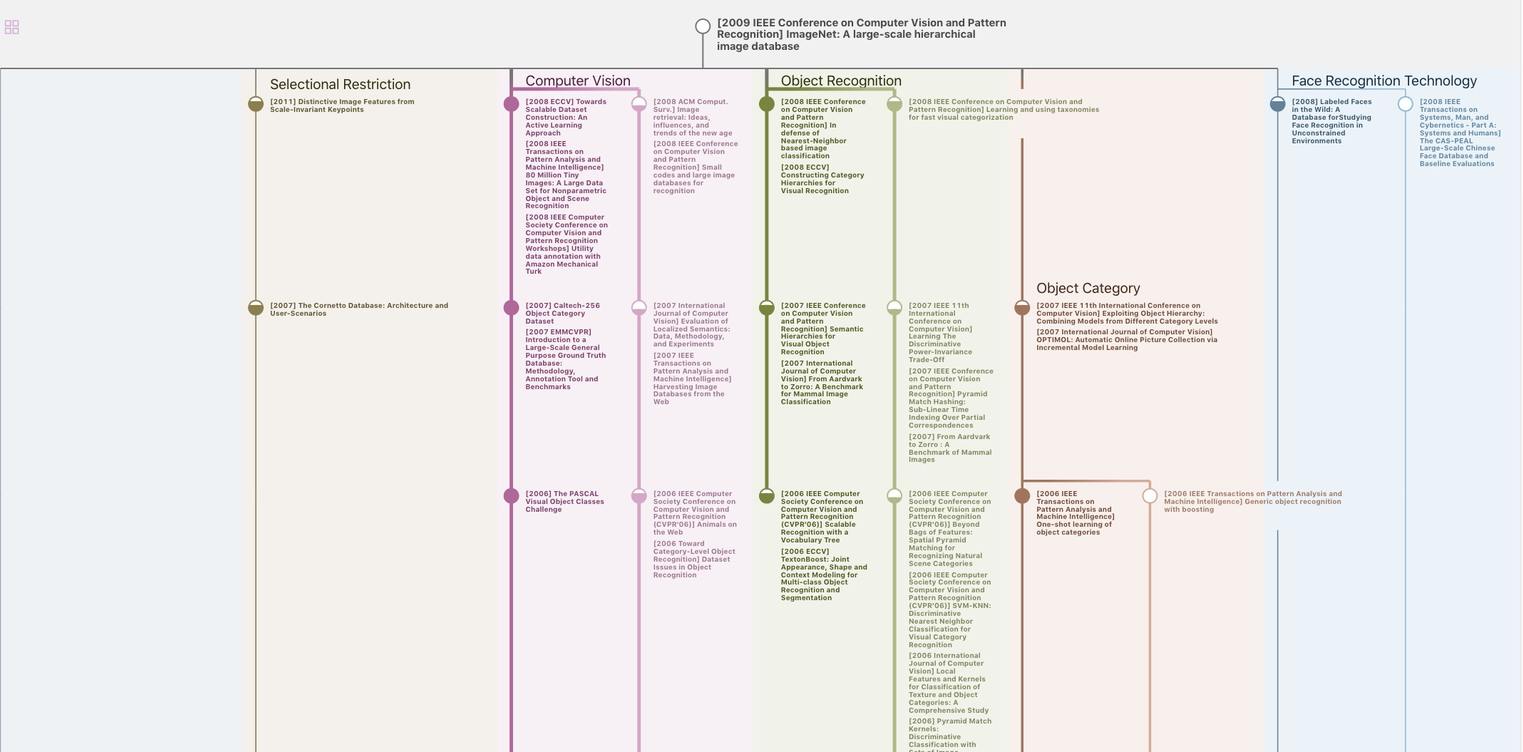
生成溯源树,研究论文发展脉络
Chat Paper
正在生成论文摘要