DLBLS_SS: protein secondary structure prediction using deep learning and broad learning system.
RSC advances(2022)
摘要
Protein secondary structure prediction (PSSP) is not only beneficial to the study of protein structure and function but also to the development of drugs. As a challenging task in computational biology, experimental methods for PSSP are time-consuming and expensive. In this paper, we propose a novel PSSP model DLBLS_SS based on deep learning and broad learning system (BLS) to predict 3-state and 8-state secondary structure. We first use a bidirectional long short-term memory (BLSTM) network to extract global features in residue sequences. Then, our proposed SEBTCN based on temporal convolutional networks (TCN) and channel attention can capture bidirectional key long-range dependencies in sequences. We also use BLS to rapidly optimize fused features while further capturing local interactions between residues. We conduct extensive experiments on public test sets including CASP10, CASP11, CASP12, CASP13, CASP14 and CB513 to evaluate the performance of the model. Experimental results show that our model exhibits better 3-state and 8-state PSSP performance compared to five state-of-the-art models.
更多查看译文
关键词
secondary structure prediction,protein,deep learning
AI 理解论文
溯源树
样例
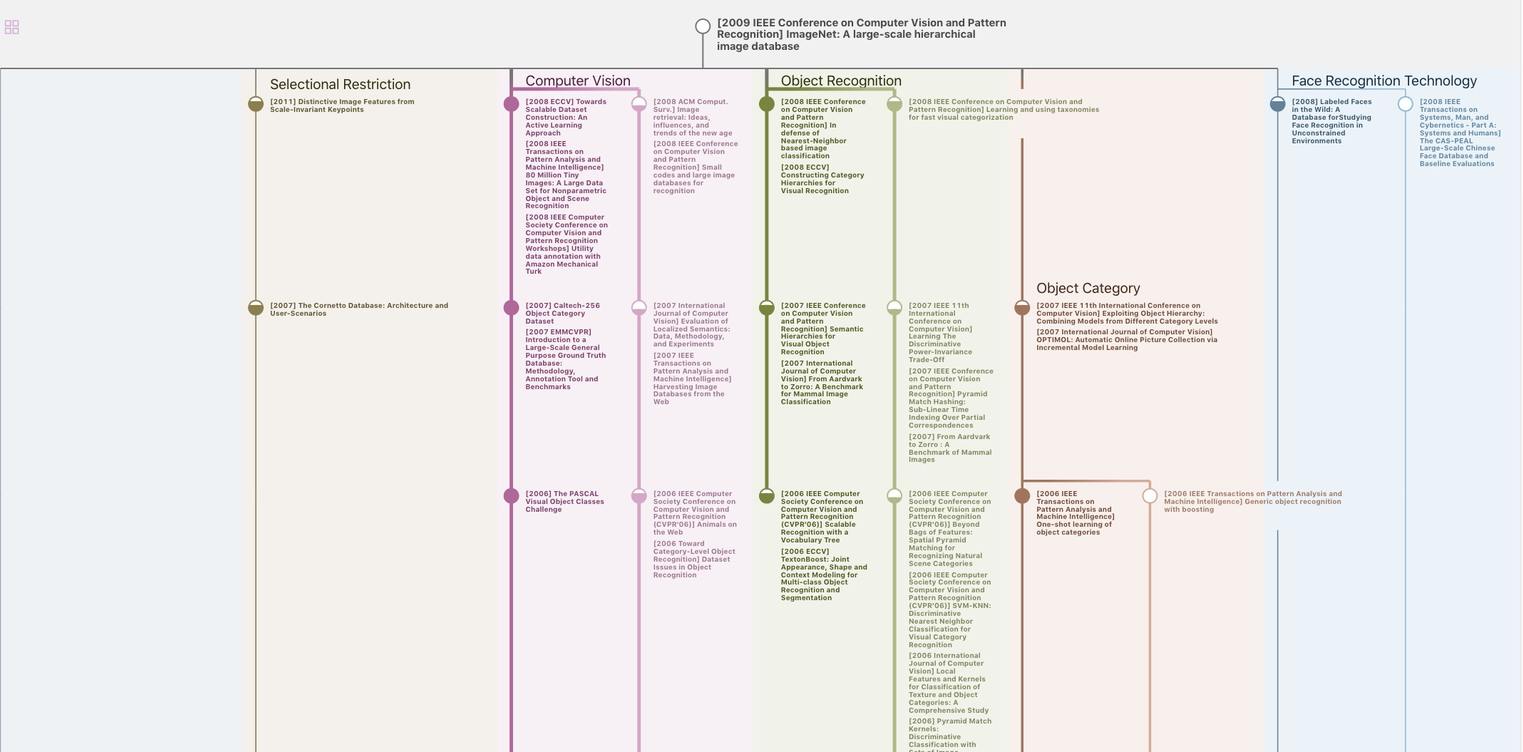
生成溯源树,研究论文发展脉络
Chat Paper
正在生成论文摘要