CACTI: A Framework for Scalable Multi-Task Multi-Scene Visual Imitation Learning
arxiv(2023)
摘要
Large-scale training have propelled significant progress in various sub-fields of AI such as computer vision and natural language processing. However, building robot learning systems at a comparable scale remains challenging. To develop robots that can perform a wide range of skills and adapt to new scenarios, efficient methods for collecting vast and diverse amounts of data on physical robot systems are required, as well as the capability to train high-capacity policies using such datasets. In this work, we propose a framework for scaling robot learning, with specific focus on multi-task and multi-scene manipulation in kitchen environments, both in simulation and in the real world. Our proposed framework, CACTI, comprises four stages that separately handle: data collection, data augmentation, visual representation learning, and imitation policy training, to enable scalability in robot learning . We make use of state-of-the-art generative models as part of the data augmentation stage, and use pre-trained out-of-domain visual representations to improve training efficiency. Experimental results demonstrate the effectiveness of our approach. On a real robot setup, CACTI enables efficient training of a single policy that can perform 10 manipulation tasks involving kitchen objects, and is robust to varying layouts of distractors. In a simulated kitchen environment, CACTI trains a single policy to perform 18 semantic tasks across 100 layout variations for each individual task. We will release the simulation task benchmark and augmented datasets in both real and simulated environments to facilitate future research.
更多查看译文
关键词
visual,learning,multi-task,multi-scene
AI 理解论文
溯源树
样例
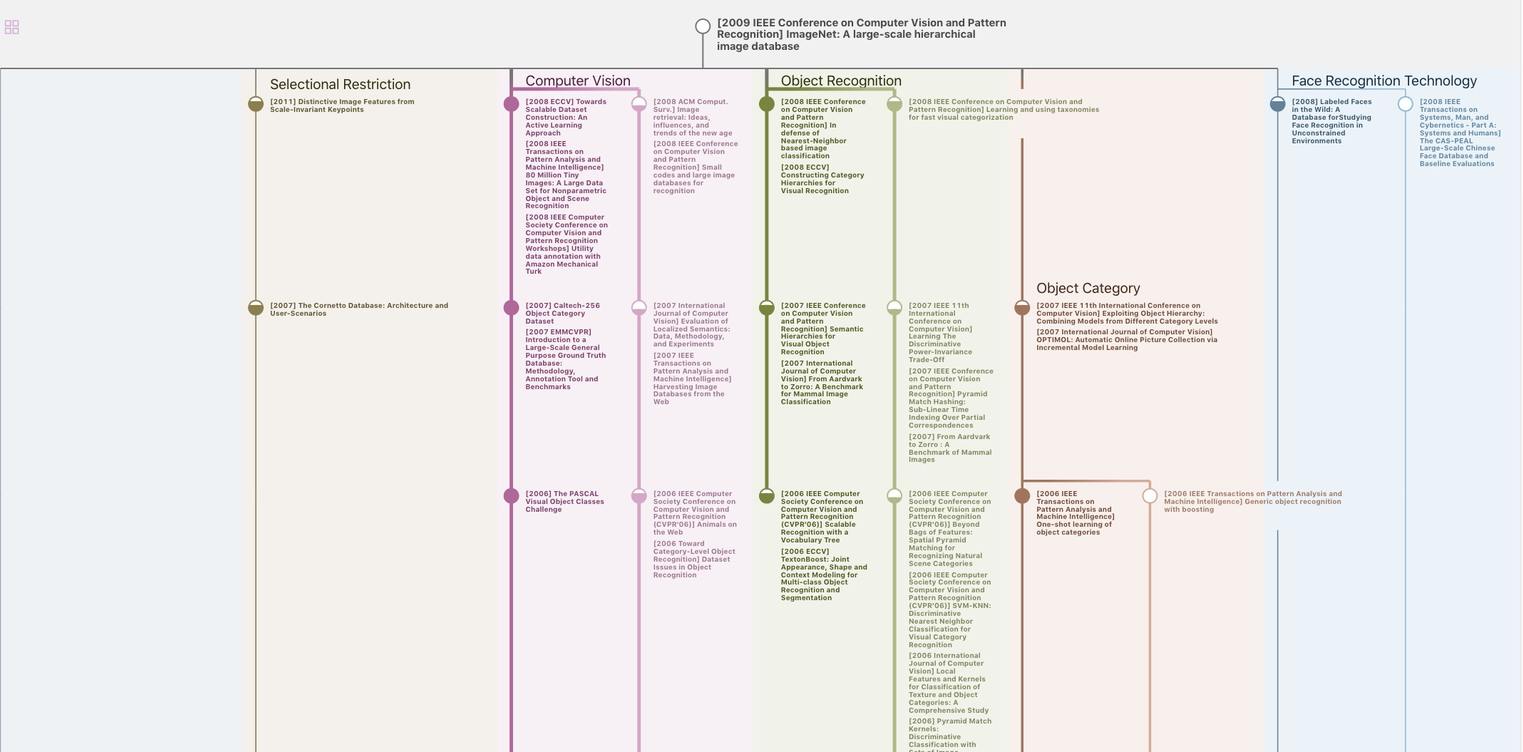
生成溯源树,研究论文发展脉络
Chat Paper
正在生成论文摘要