Parameter Estimation with Maximal Updated Densities
COMPUTER METHODS IN APPLIED MECHANICS AND ENGINEERING(2023)
Key words
Uncertainty quantification,Inverse problems,Push-forward measure,Pullback measure,Parameter estimation
AI Read Science
Must-Reading Tree
Example
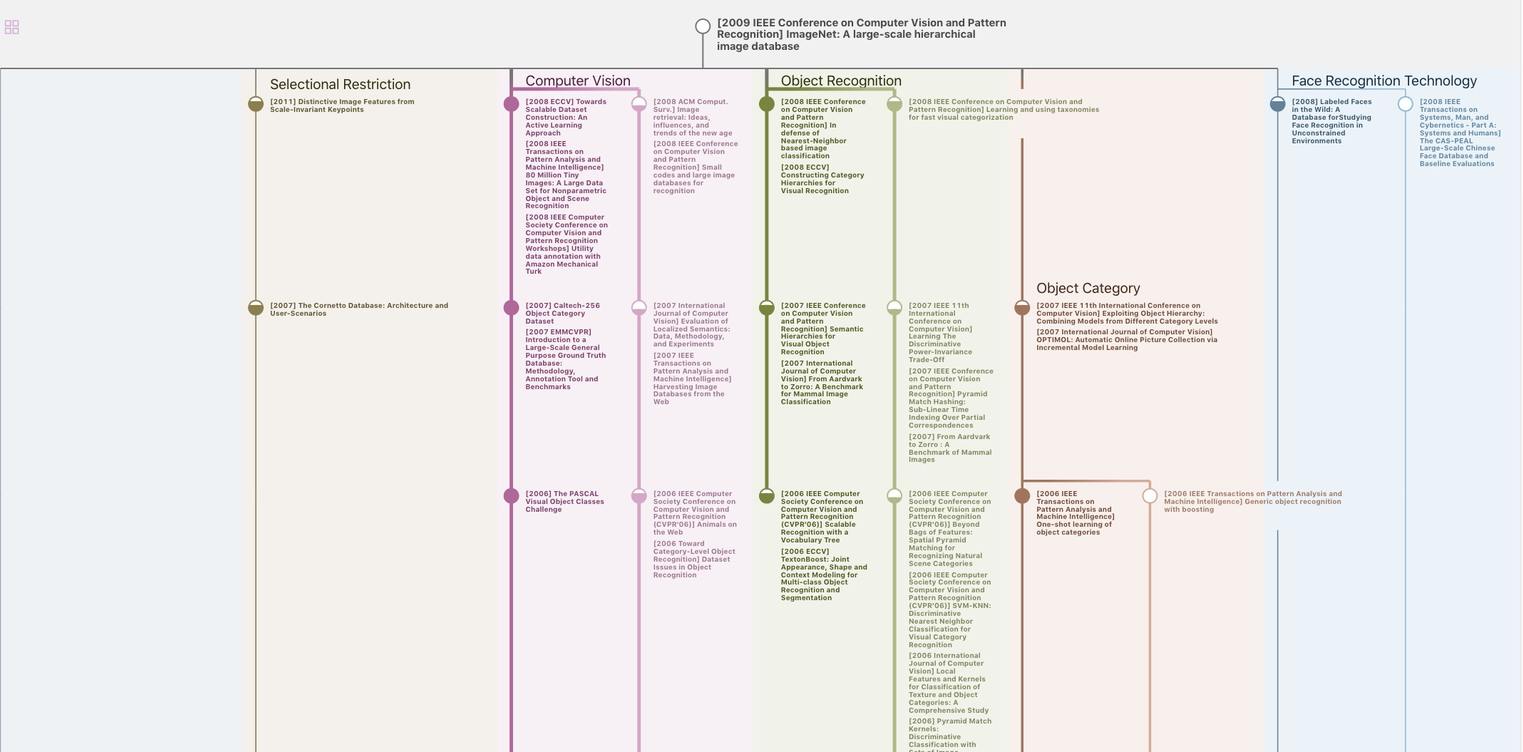
Generate MRT to find the research sequence of this paper
Chat Paper
Summary is being generated by the instructions you defined