Determination of the quality of tea from different picking periods: An adaptive pooling attention mechanism coupled with an electronic nose
Postharvest Biology and Technology(2023)
摘要
An efficient nondestructive testing method is important to inspect the quality of agricultural products. The material content of tea differs across different picking periods, leading to variation in the quality. Traditional methods for evaluating the quality of tea are relatively complex, in-efficient, and generally destructive. This prompted our work, which led to the development of nondestructive testing technology comprising an electronic nose (e-nose) combined with an effective data analysis method, to determine the quality of tea from different picking periods. First, the e-nose system was used to acquire information about the volatile components of tea from different picking periods. Second, an adaptive pooling attention mechanism (APAM) is proposed based on the data characteristics of the e-nose system. By adapting the attention to the essential information that affects the classification performance, the feature-learning ability of the gas information is enhanced. Finally, the proposed APAM was combined with a classic convolutional neural network (CNN) to identify the gas information of tea picked during different picking periods. The method was evaluated in comparison with other gas recognition methods to prove its effectiveness. The results show that APAM can effectively realize the adaptive attention to the deep gas information. The MobileNetV1 +APAM has accuracy of 97.62%, precision of 97.57%, recall of 97.71%, and F1-score of 97.64%, confirming the capability of the method to successfully distinguish among the gas information of tea gathered in different picking periods. In summary, integrating APAM with a classic CNN combined with e-nose technology offers an effective nondestructive testing method for agricultural production and tea quality evaluation.
更多查看译文
关键词
Tea quality determination,Nondestructive testing,Electronic nose,Adaptive pooling attention mechanism,Gas identification
AI 理解论文
溯源树
样例
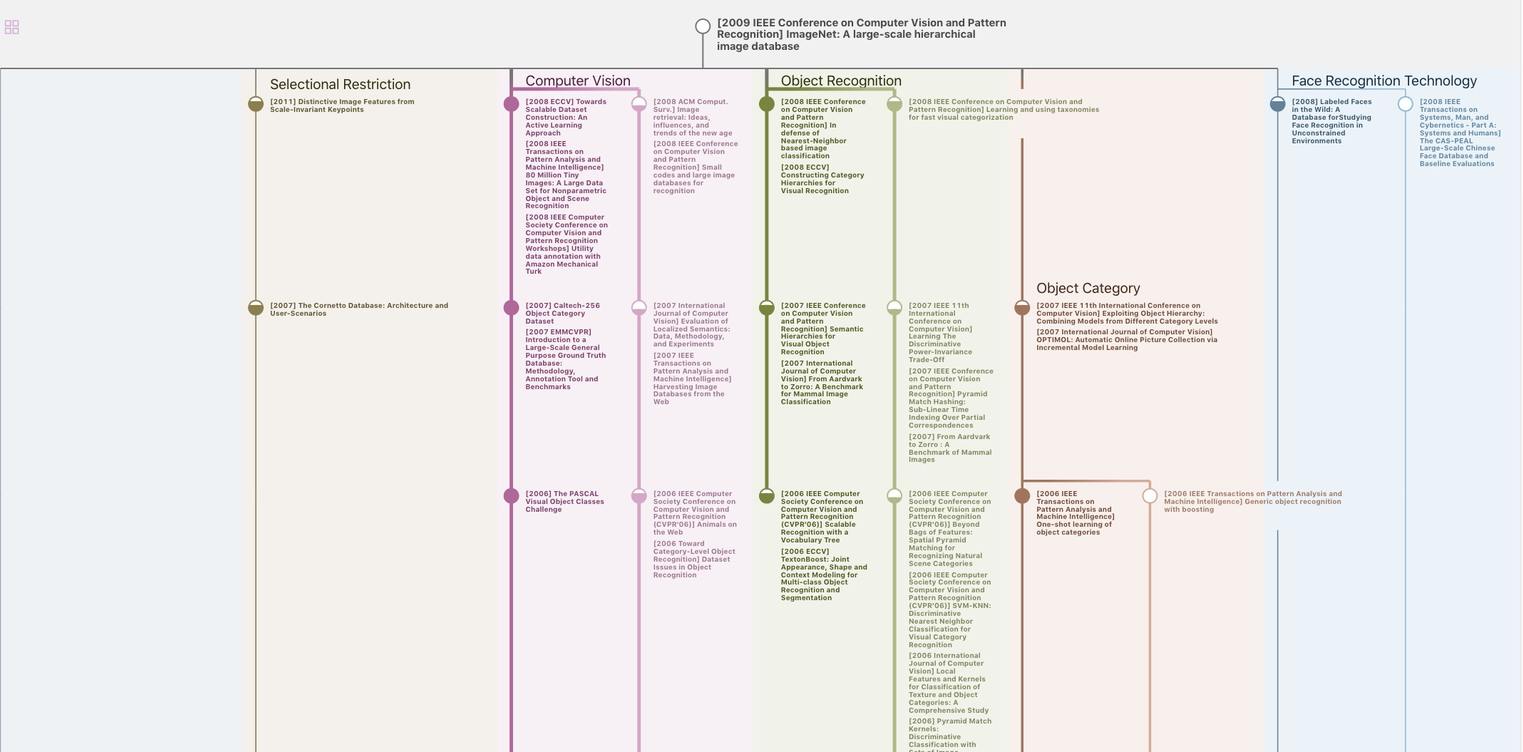
生成溯源树,研究论文发展脉络
Chat Paper
正在生成论文摘要