Speech Enhancement Model Synthesis Based on Federal Learning for Industrial CPS in Multiple Noise Conditions.
Symmetry(2022)
摘要
Real-time acquisition of industrial production data and rapid response to changes in the external environment are key to ensuring the symmetry of a CPS. However, during industrial production, the collected data are inevitably disturbed by environmental noise, which has a huge impact on the subsequent data processing of a CPS. The types of noise vary greatly in different work scenarios in a factory. Meanwhile, barriers such as data privacy protection and copyright restrictions create great difficulties for model synthesis in the information space. A speech enhancement model with teacher-student architecture based on federal knowledge distillation is proposed to alleviate this problem. (1) We pre-train teacher models under different noise conditions to create multiple teacher models with symmetry and excelling in the suppression of a priori noise. (2) We construct a symmetric model-student model of the physical space of the teacher model trained on public data and transfer the knowledge of the teacher model to the student model. The student model can suppress multiple types of noise. Notably, with the TIMIT dataset and the NoiseX92 noise set, the accuracy of the proposed method improved by an average of 1.00% over the randomly specified teacher method in the PESQ metric and 0.17% for STOI.
更多查看译文
关键词
speech enhancement,CPS,symmetry,knowledge distillation,unsupervised
AI 理解论文
溯源树
样例
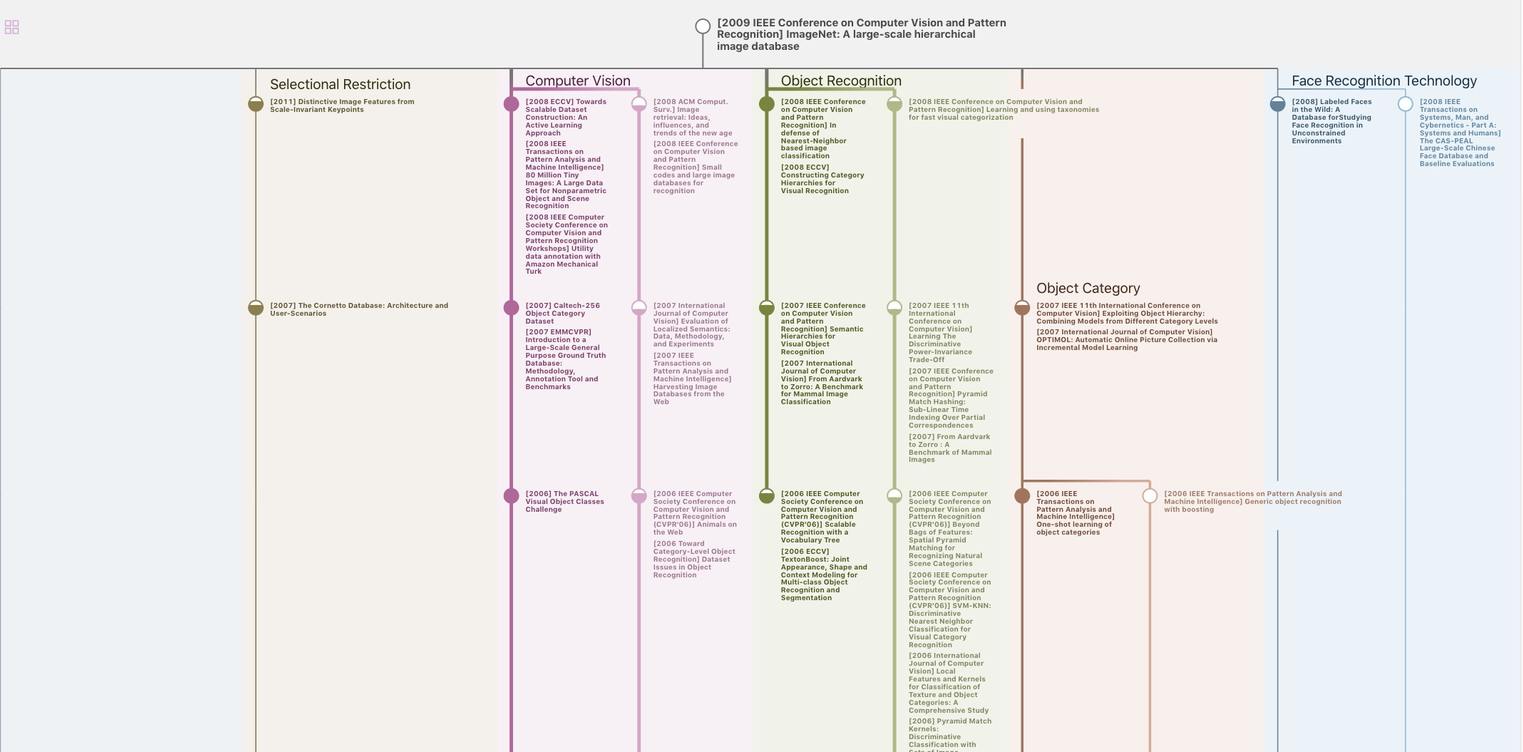
生成溯源树,研究论文发展脉络
Chat Paper
正在生成论文摘要