The Inherited Rate Matrix algorithm for phylogenetic model selection for non-stationary Markov processes
biorxiv(2022)
摘要
In phylogenetic reconstruction, substitution models that assume nucleotide frequencies do not change through time are in very widespread use. DNA sequences can exhibit marked compositional difference. Such compositional heterogeneity implies sequence divergence has arisen from substitution processes that differ between the lineages. Accordingly, there has been considerable interest in exploring the non-stationary model class, which is capable of generating divergent sequence composition. These models are typically parameter rich and this richness is further compounded by the use of time-heterogeneous variants in order to capture compositional heterogeneity. This illuminates two barriers limiting the widespread application of the non-stationary model class: both the compute-time required to fit a model and the risk of model over-fitting increase with the number of parameters and the extent of time-heterogeneity. In this study, we address these issues with a novel model selection algorithm we term the Inherited Rate Matrix algorithm (hereafter IRM). This approach is based on the notion that a species inherits the substitution tendencies of its ancestor. We further present the non-stationary heterogeneous across lineages model (hereafter ns-HAL algorithm) which extends the HAL algorithm of Jayaswal et al (2014) to the general nucleotide Markov process. The IRM algorithm substantially reduces the complexity of identifying a sufficient solution to the problem of time-heterogeneous substitution processes across lineages. We also address the issue of reducing the computing time with development of a constrained-optimisation approach for the IRM algorithm (fast-IRM). Our algorithms are implemented in Python 3. From a simulation study based on 2nd codon position genome sequences of yeast, we establish that IRM is significantly more accurate than both ns-HAL and heterogeneity in the substitution process across lineages (hereafter HAL) for close and dispersed sequences. IRM is up to 70x faster than ns-HAL while fast-IRM is around 140x faster. fast-IRM also showed a marked speed improvement over a C++ implementation of HAL. Our comparison of the accuracy of IRM with fast-IRM showed no difference with identical inferences made for all data sets. These two algorithms greatly improve the compute time for model selection of a non-stationary process, increasing the suite of problems to which this important substitution model class can be applied.
### Competing Interest Statement
The authors have declared no competing interest.
更多查看译文
关键词
phylogenetic model selection,inherited rate matrix algorithm,markov,non-stationary
AI 理解论文
溯源树
样例
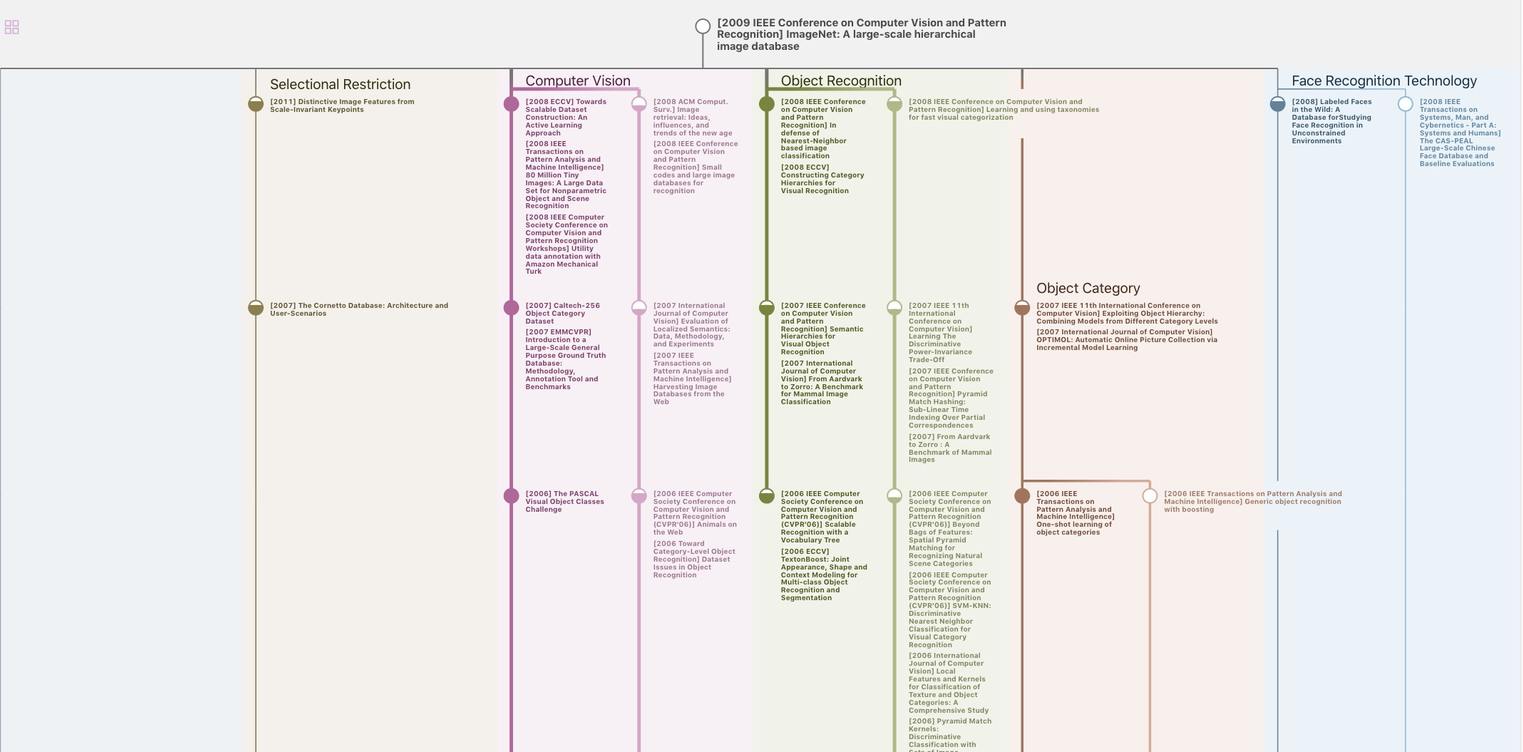
生成溯源树,研究论文发展脉络
Chat Paper
正在生成论文摘要