Attentive Multi-Task Prediction of Atmospheric Particulate Matter: Effect of the COVID-19 Pandemic.
IEEE Access(2022)
摘要
Air pollution, especially the continual increase in atmospheric particulate matter (PM), is a global environmental challenge. To reduce the PM concentration, a remarkable amount of machine learning-based research has been proposed. However, increasing the accuracy of the predictions and providing clear interpretations of the predictions are challenging. In particular, no studies have addressed models that predict and interpret PM before and during the COVID-19 pandemic. In this paper, we present a two-step predictive and explainable model to obtain insights into reducing PM. We first use attentive multi-task learning to predict the air quality of cities. To accurately predict the concentration of particles with sizes of similar to 10 mu m or similar to 2.5 mu m (PM10 and PM2.5, respectively), we demonstrate a performance difference between single-task and multi-task learning, as well as among the state-of-the art methods. The proposed attentive model with multi-task learning outperformed the others in terms of accuracy performance. We then used Shapley additive explanations, a representative explainable artificial intelligence framework, to interpret and determine the significance of features for predicting PM10 and PM2.5. We demonstrated the superiority of the proposed approach in predicting and explaining both PM10 and PM2.5 concentrations, and observed a statistically significant difference in air pollution before and during the COVID-19 pandemic.
更多查看译文
关键词
Air pollution,attentive multi-task learning,explainable artificial intelligence,particulate matter,surrogate model,Shapley value
AI 理解论文
溯源树
样例
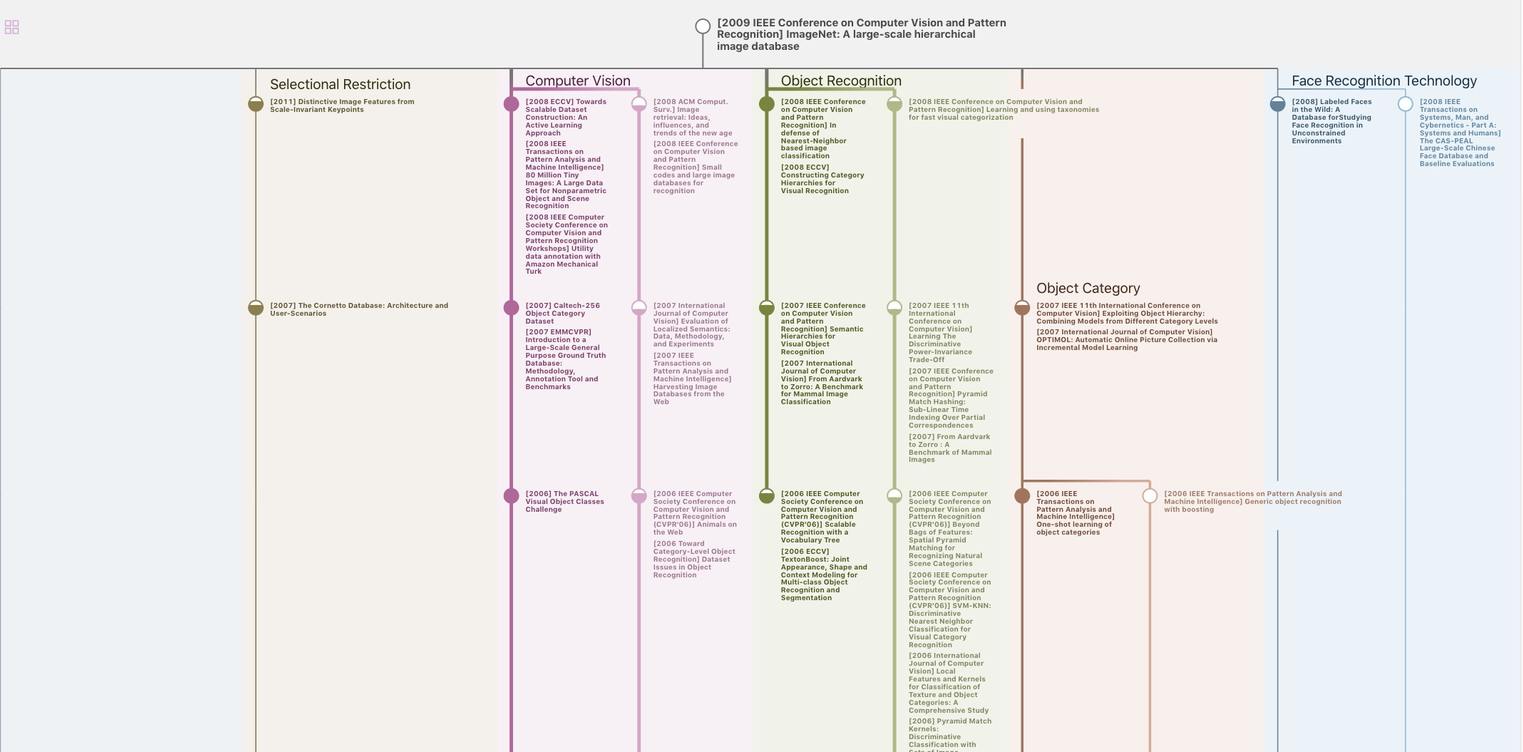
生成溯源树,研究论文发展脉络
Chat Paper
正在生成论文摘要