Batch Endpoint Prediction using Local Mixture of Batch Time Experts
2022 IEEE International Conference on Metrology for Extended Reality, Artificial Intelligence and Neural Engineering (MetroXRAINE)(2022)
摘要
Some batch processes have large variability in batch-to-batch time completion, possibly because of the different process conditions and external factors. The time of batch completion is commonly inferred from process experts. However, this may lead to inaccuracies due to the uncertainty associated with the batch-to-batch variations, leading the process to run more than is needed. Process engineers could appeal to statistical process monitoring and control mechanisms to estimate the batch time completion. However, the existing tools for batch prediction rely on dynamic time warping, which is not straightforward to implement and unsuitable for estimating the remaining batch time in real-time. This work presents a new framework for remaining batch time prediction in real-time by combining local models of each batch using the mixture of local experts models. A local batch time model is learned for each batch separately and combined to predict the remaining batch time. When a new sample becomes available on real-time data, the framework detects the probabilistic similarity of the current batch sample to the historical batch data. The remaining batch time is estimated by weighting the local batch time models. This approach has been evaluated on a polymerization benchmark data set and has shown promising results. The results show that the mixture of local batch experts can predict the remaining batch time without the need for any data alignment. Thus, simplifying the process of building process monitoring control tools for the remaining batch time prediction.
更多查看译文
关键词
Industrial processes,soft sensors,optimization,batch processes
AI 理解论文
溯源树
样例
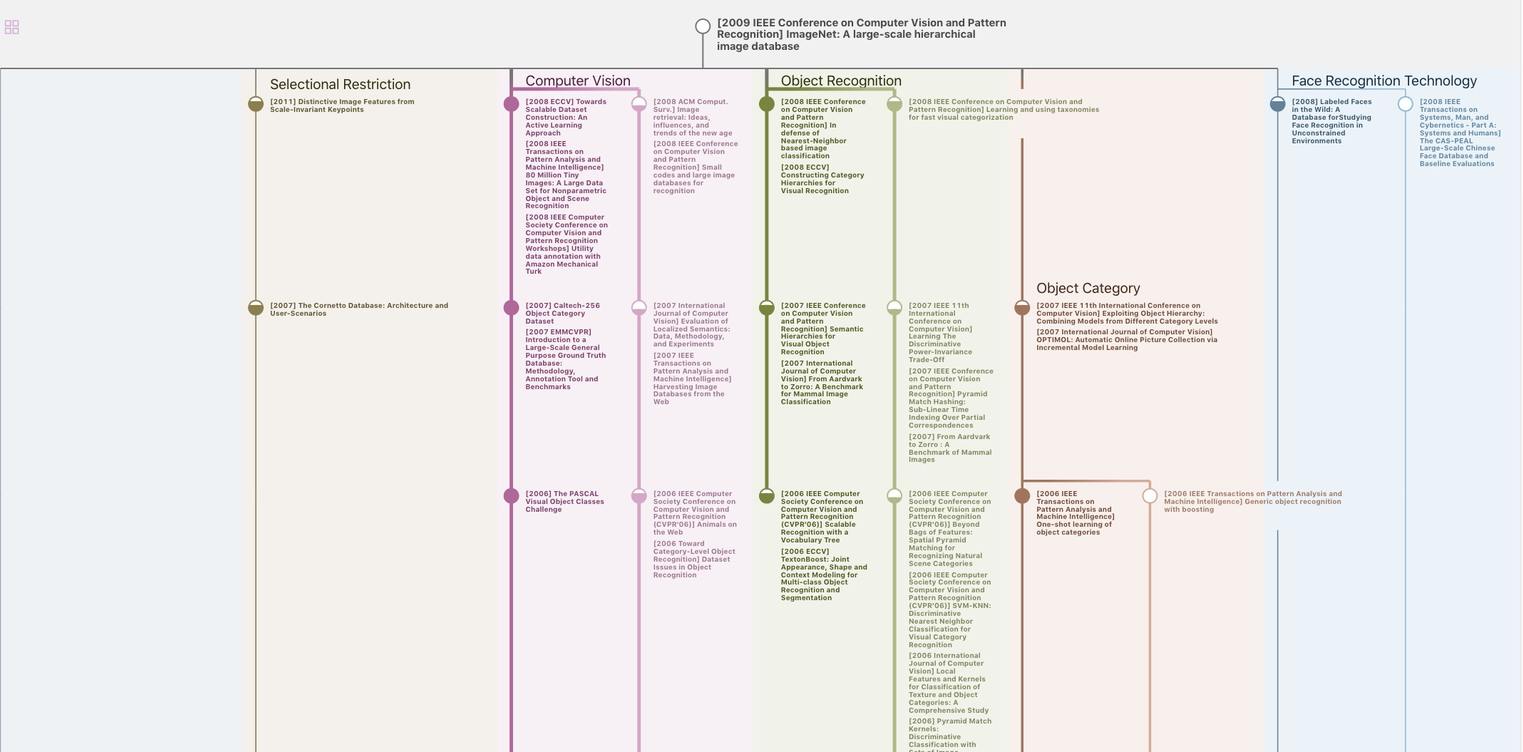
生成溯源树,研究论文发展脉络
Chat Paper
正在生成论文摘要