Multi-granularity Pseudo-label Collaboration for unsupervised person re-identification
Computer Vision and Image Understanding(2023)
摘要
Unsupervised person re-identification (ReID) aims at training a model through using fully unlabeled training images. Most successful approaches combine clustering-based pseudo-label prediction with ReID model learning and perform the two steps in an alternating fashion. However, there are some person images, which have common appearance but different identities or have same identity but large intra-variations, and they are prone to be assigned wrong pseudo-labels. Existing methods typically infer person image pseudo-labels in a single pseudo-label space, which is insufficient due to losing the accurate labels of these hard images. This limits model's power to cope with varying changes. To address this problem, we propose a Multi-granularity Pseudo -label Collaboration (MPC) method for unsupervised person ReID. Firstly, a multi-granularity pseudo-label prediction (MPP) method is proposed to predict the pseudo-labels of person images. MPP generates multiple pseudo-label spaces with each one inferring the person image pseudo-labels under a different granularity at cluster size. Secondly, the multiple pseudo-label spaces are applied to learn a mixture of experts (MoE) model. Each expert model is supervised by the pseudo-labels in a specific pseudo-label space, enabling MoE model to handle different variations. The output of these expert models are fused critically by a bilateral attention aggregation (BAA) to generate aggregation image features. Meanwhile, a label mix-up method is designed for refining pseudo-labels. It fuses labels across different pseudo-label spaces based on label co-occurrence to promote aggregation feature learning. We extensively evaluate the proposed MPC method under both image -based and video-based unsupervised person ReID settings. MPC significantly improves the current baseline method by a margin of 5.4% and 3.7% in mAP, and 3.4% and 3.9% in Rank1 accuracy on the image-based datasets Market-1501 and DukeMTMC-ReID, respectively. In particular, MPC method achieves state-of-the-art performance on video-based datasets with mAP of 71.4% and 87.3% on MARS and DukeMTMC-VideoReID, respectively.
更多查看译文
关键词
Unsupervised person re-identification,Multi-granularity pseudo-label prediction,Mix-up
AI 理解论文
溯源树
样例
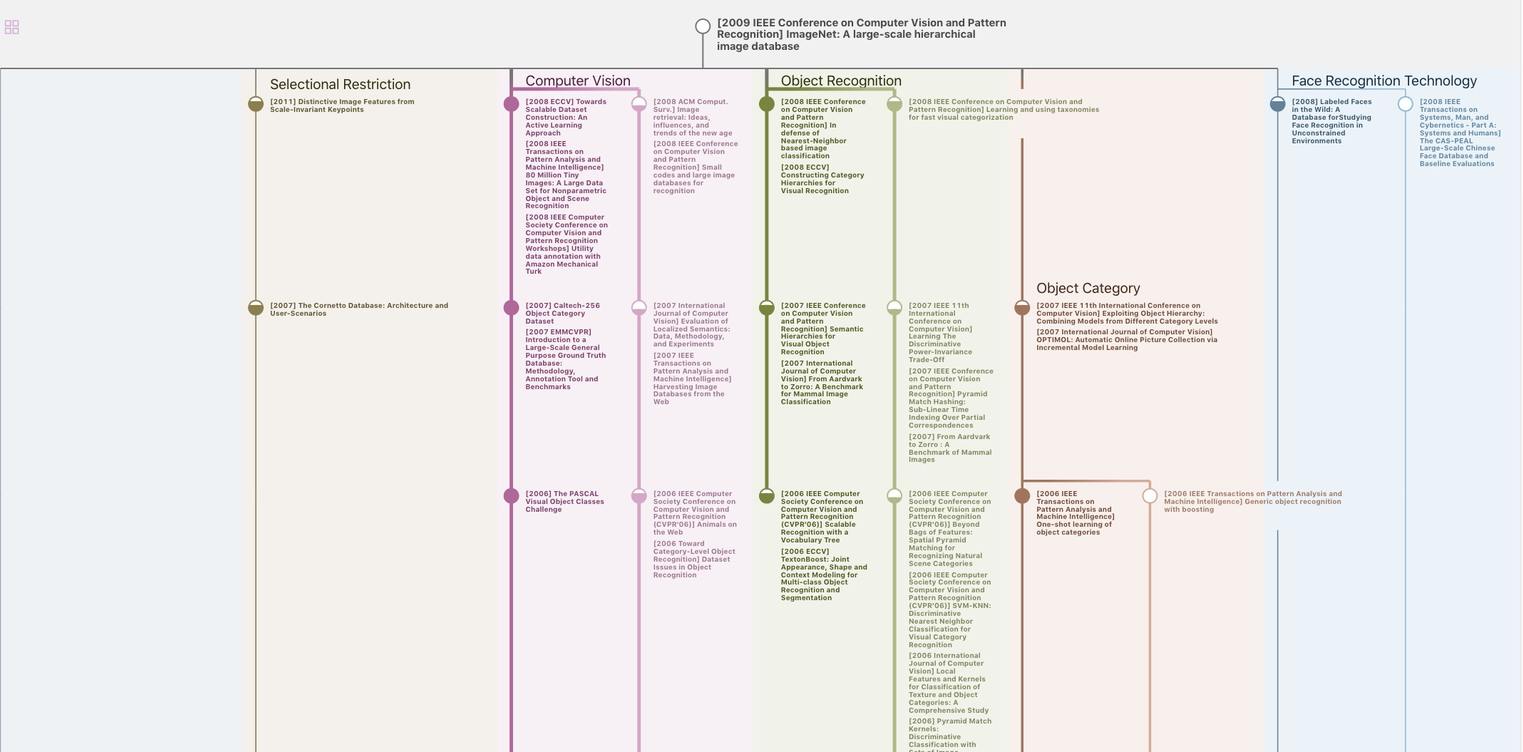
生成溯源树,研究论文发展脉络
Chat Paper
正在生成论文摘要