On the Energy and Communication Efficiency Tradeoffs in Federated and Multi-Task Learning
2022 IEEE 33rd Annual International Symposium on Personal, Indoor and Mobile Radio Communications (PIMRC)(2022)
摘要
Recent advances in Federated Learning (FL) have paved the way towards the design of novel strategies for solving multiple learning tasks simultaneously, by leveraging cooperation among networked devices. Multi-Task Learning (MTL) exploits relevant commonalities across tasks to improve efficiency compared with traditional transfer learning approaches. By learning multiple tasks jointly, significant reduction in terms of energy footprints can be obtained. This article provides a first look into the energy costs of MTL processes driven by the Model-Agnostic Meta-Learning (MAML) paradigm and implemented in distributed wireless networks. The paper targets a clustered multi-task network setup where autonomous agents learn different but related tasks. The MTL process is carried out in two stages: the optimization of a meta-model that can be quickly adapted to learn new tasks, and a task-specific model adaptation stage where the learned meta-model is transferred to agents and tailored for a specific task. This work analyzes the main factors that influence the MTL energy balance by considering a multi-task Reinforcement Learning (RL) setup in a robotized environment. Results show that the MAML method can reduce the energy bill by at least 2 × compared with traditional approaches without inductive transfer. Moreover, it is shown that the optimal energy balance in wireless networks depends on uplink/downlink and sidelink communication efficiencies.
更多查看译文
关键词
communication efficiency tradeoffs,federated,energy,learning,multi-task
AI 理解论文
溯源树
样例
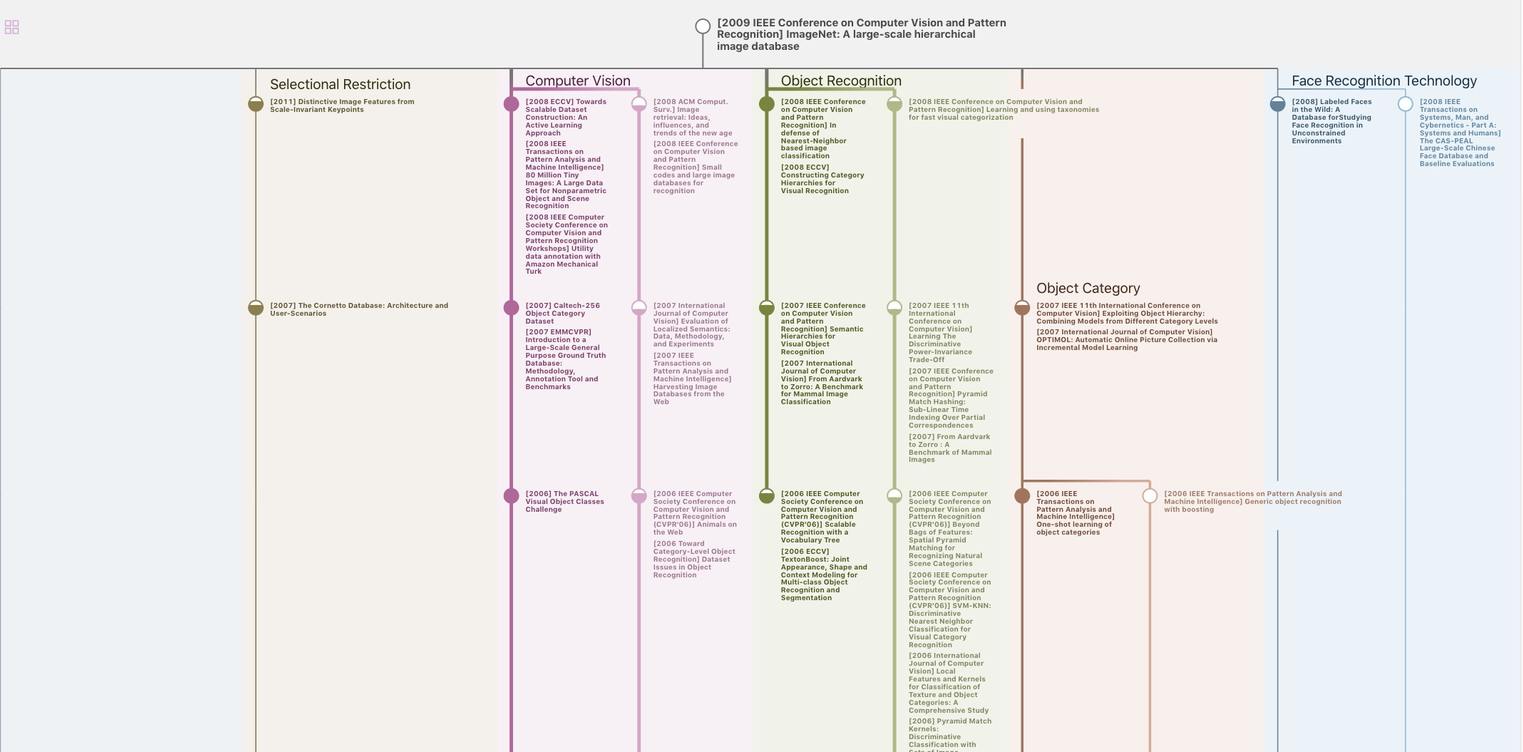
生成溯源树,研究论文发展脉络
Chat Paper
正在生成论文摘要