A comparative study on the most effective machine learning model for blast loading prediction: From GBDT to Transformer
Engineering Structures(2023)
摘要
In this paper, we present a rigorous comparative study to assess and identify the most effective machine learning model for blast loading prediction. Blast loads are known to produce catastrophic effects including structural collapse and personnel fatality. Accurate and efficient prediction of these extreme loads using empirical methods and numerical solvers remains a challenging problem. Machine learning provides a promising alternative solution, which has been increasingly used in various engineering applications. However, there is seldom any analysis or justification of the selection of machine learning method that would lead to the best performance for such applications. For example, most existing machine learning-based approaches for blast loading prediction utilise the classic multi-layer perceptron (MLP) network with no justifications of their suitability and efficiency nor attempts of leveraging other state-of-the-art neural network architectures. In this study, four well-known machine learning models, including one ensemble tree method and three neural networks of different types, are investigated to demonstrate the effectiveness of different machine learning methods for blast loading prediction. It is showcased using BLEVE (boiling liquid expanding vapour explosion) pressure prediction that the Transformer model achieves the best performance, reaching a relative error of 3.5% and R2 0.997 that outperforms the existing MLP approach (relative error 6.0%, R2 0.985) with a clear margin. This study shows that the Transformer network is an effective tool for prediction of blast loading from BLEVE as well as other explosion sources.
更多查看译文
关键词
Blast loading,Machine learning,Transformer,Neural network,BLEVE
AI 理解论文
溯源树
样例
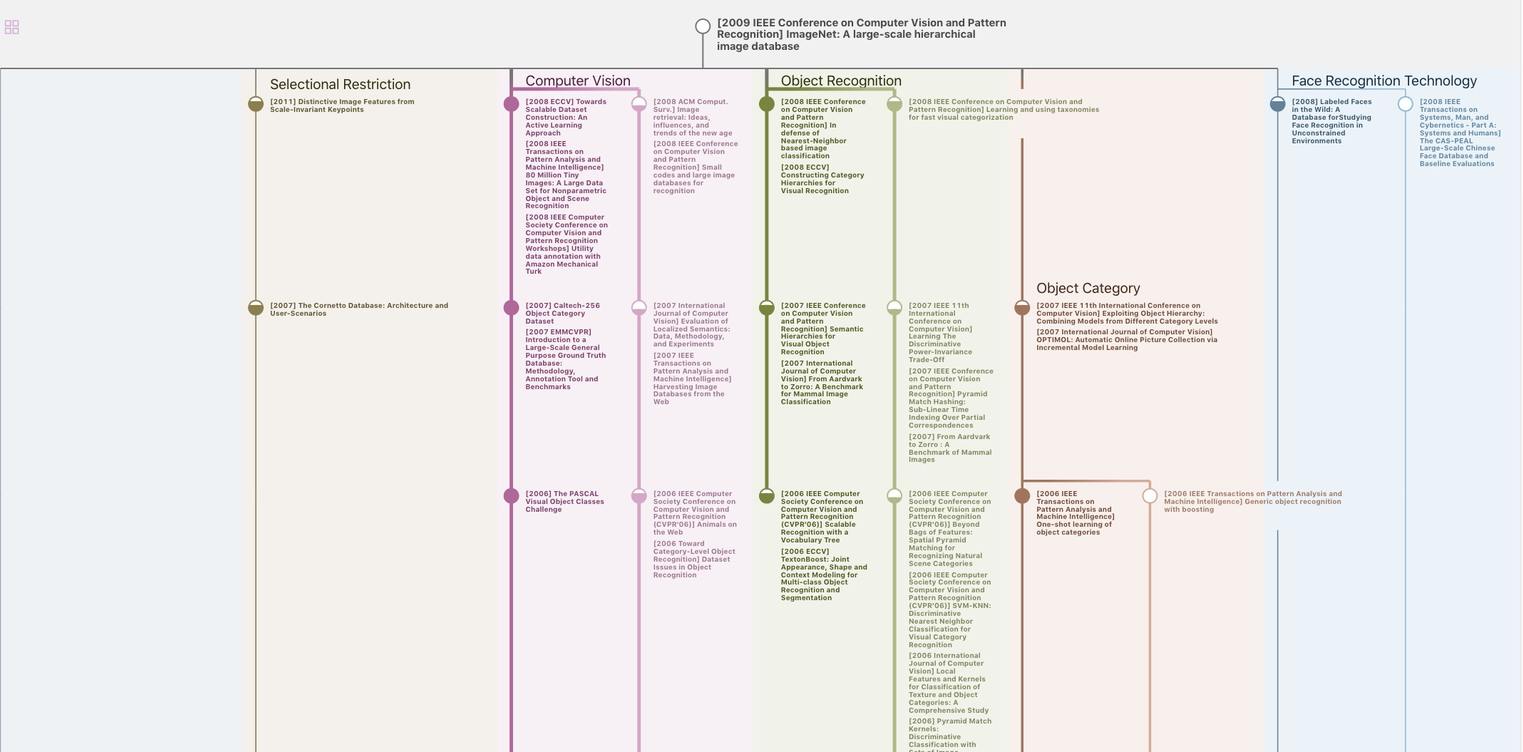
生成溯源树,研究论文发展脉络
Chat Paper
正在生成论文摘要