Single-Phase Fault Detection Based on GCN-TCN Sparse-Attention Model
2022 12th International Conference on Advanced Computer Information Technologies (ACIT)(2022)
摘要
Effective extraction of fault current features is the problem facing single phase fault detection in distribution networks. We propose a hybrid neural network model based on the GCN-TCN sparse attention mechanism (GTHNN-SA) to solve this problem. Specifically, this model uses the convolutional graph network (GCN) to perform graph convolution operation on the graph data established by the fault line transient zero-sequence current, extract the spatial characteristics of the zero-sequence current data with the fault location relationship, use the temporal convolution network (TCN) to extract the temporal characteristics both of threephase currents and new data generated by GAN. Then fuse and input the three feature matrices to the sparse attention mechanism to highlight essential features of current data. Finally, the output of parse-attention is input to the entire connected layer for classification. This model enables us to learn the current data relationship between different waveform faults more comprehensively, and the proposed method has a good effect on single-phase fault detection in distribution networks.
更多查看译文
关键词
graph convolutional network,temporal convolutional network,generative adversarial network,sparse attention,single-phase fault detection
AI 理解论文
溯源树
样例
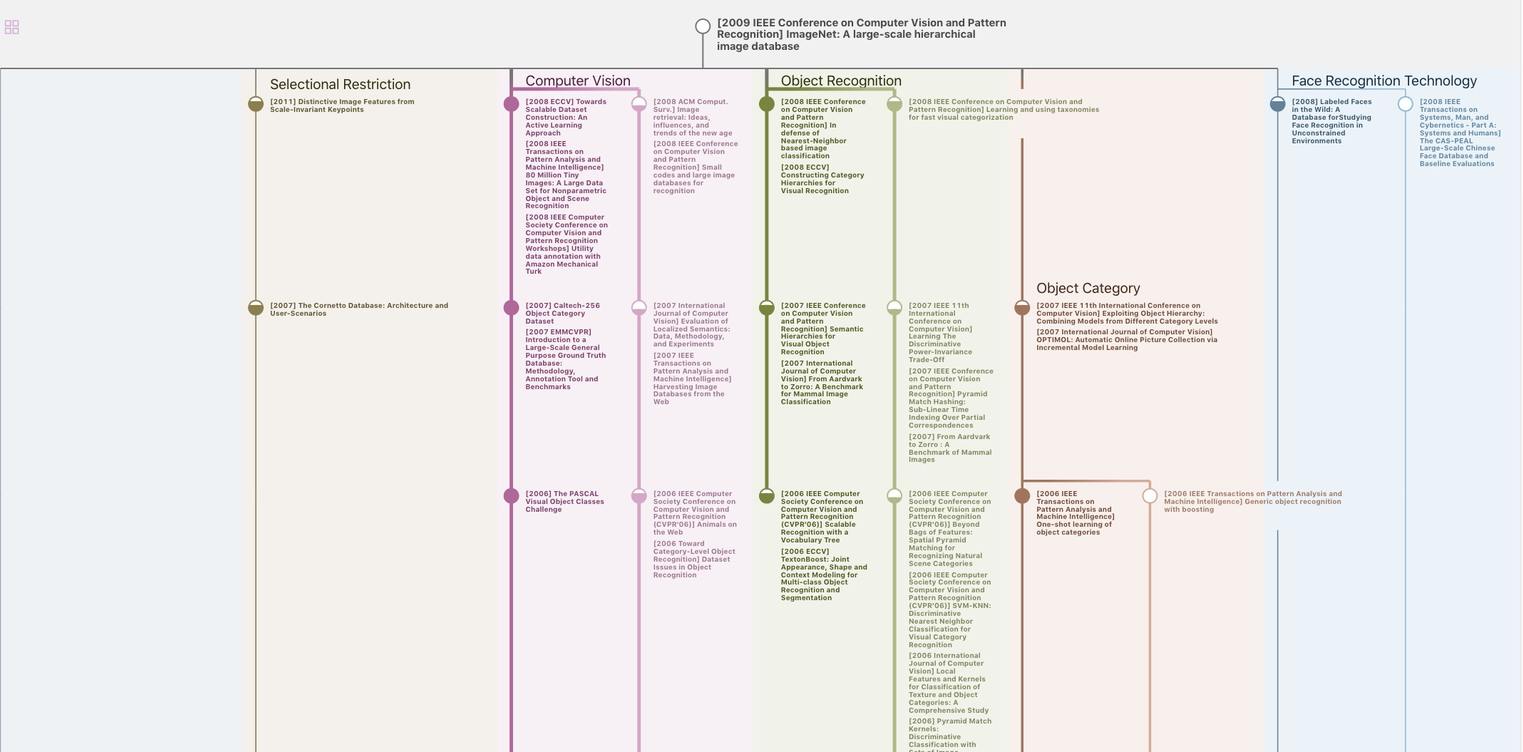
生成溯源树,研究论文发展脉络
Chat Paper
正在生成论文摘要