Random Generated Dictionaries for Convolutional Sparse Coding: An ELM Interpretation for Simple CSC Applications
2022 IEEE International Conference on Image Processing (ICIP)(2022)
摘要
The most basic ELM (extreme learning machines) architecture consists on a single-hidden layer feedforward neural network, with random input weights, plus a densely connected output layer whose weights must be learned. Among other interpretations, it can be understood as using an untrained dictionary (with random entries) along with a non-linear activation function to obtain a representation. Compared to Neural Networks (NN) or Convolutional NN (CNN), ELM is very fast to train.Inspired by the ELM methodology, in this paper we explore the usefulness of using a randomly generated filterbank (FB) as the convolutional dictionary in convolutional sparse coding (CSC) representations and assess its performance for simple applications such denoising and super resolution, when compared to learned FBs. Our main conclusions are that a randomly generated FB (i) has a competitive (restoration) performance when compared to a learned FB, (ii) its performance depends on the actual distribution of its values, i.e. Gaussian, uniform, lognormal, etc., and problem, and (iii) it may ease or potentially eliminate the need for the CDL (convolutional dictionary learning) step in CSR’s applications.
更多查看译文
关键词
convolutional sparse coding,extreme learning machines
AI 理解论文
溯源树
样例
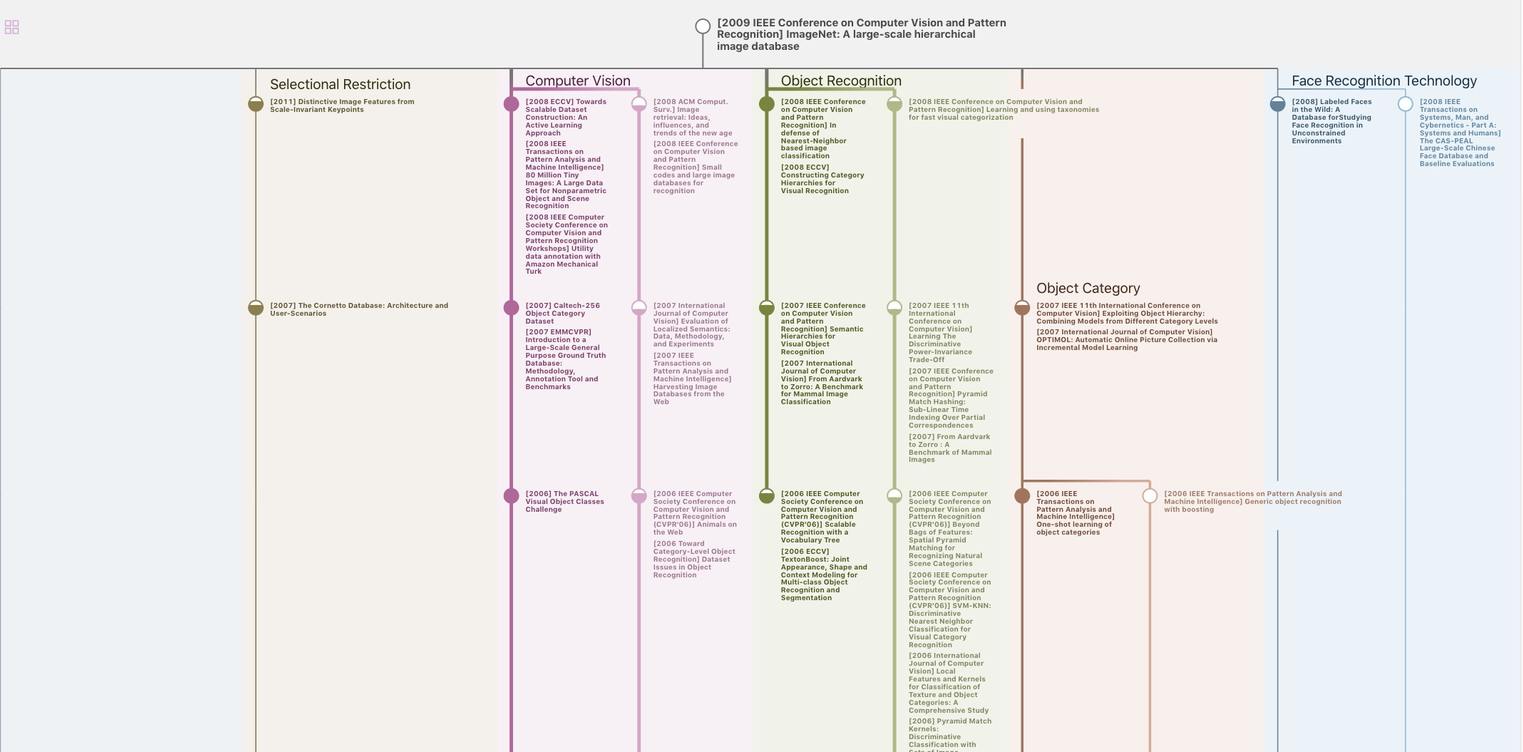
生成溯源树,研究论文发展脉络
Chat Paper
正在生成论文摘要