Explainable computer vision analysis for embryo selection on blastocyst images
2022 IEEE-EMBS International Conference on Biomedical and Health Informatics (BHI)(2022)
摘要
Infertility significantly affects the quality of life on social and psychological levels and is estimated to expand in the coming years. In vitro fertilization is the applied answer of modern medicine to the ever-rising problem of low fertility in economically developed countries. Designated experts base their decision on selecting the most suitable embryo for transfer in the uterus by reviewing blastocysts images. Therefore, subjectivity and erroneous judgement can influence the progress of the whole fertilization process since no repeatable criteria exist to characterize the quality of each embryo. Towards the quantization of the visual content of ‘wannabe babies’ embryos, a comparative study between traditional machine and deep learning techniques is conducted in this paper. The utilization of a novel unsupervised segmentation scheme for the separation of trophectoderm and inner cell mass area provides a significant boost to the performance of traditional machine learning techniques. Moreover, an explainability technique that is based on the information retrieved by the Fisher Vector's generative model provides the necessary connection between the visual stimuli and the predicted results. The classification results of the proposed methodology are comparable with state-of the-art deep learning techniques and are accompanied by corresponding visual explanations that reveal the inner workings of each model and provide useful insight concerning the predictions' validity.
更多查看译文
关键词
In Vitro Fertilization,Explainability,Fisher Vector,Unsupervised Segmentation,Grad-CAM
AI 理解论文
溯源树
样例
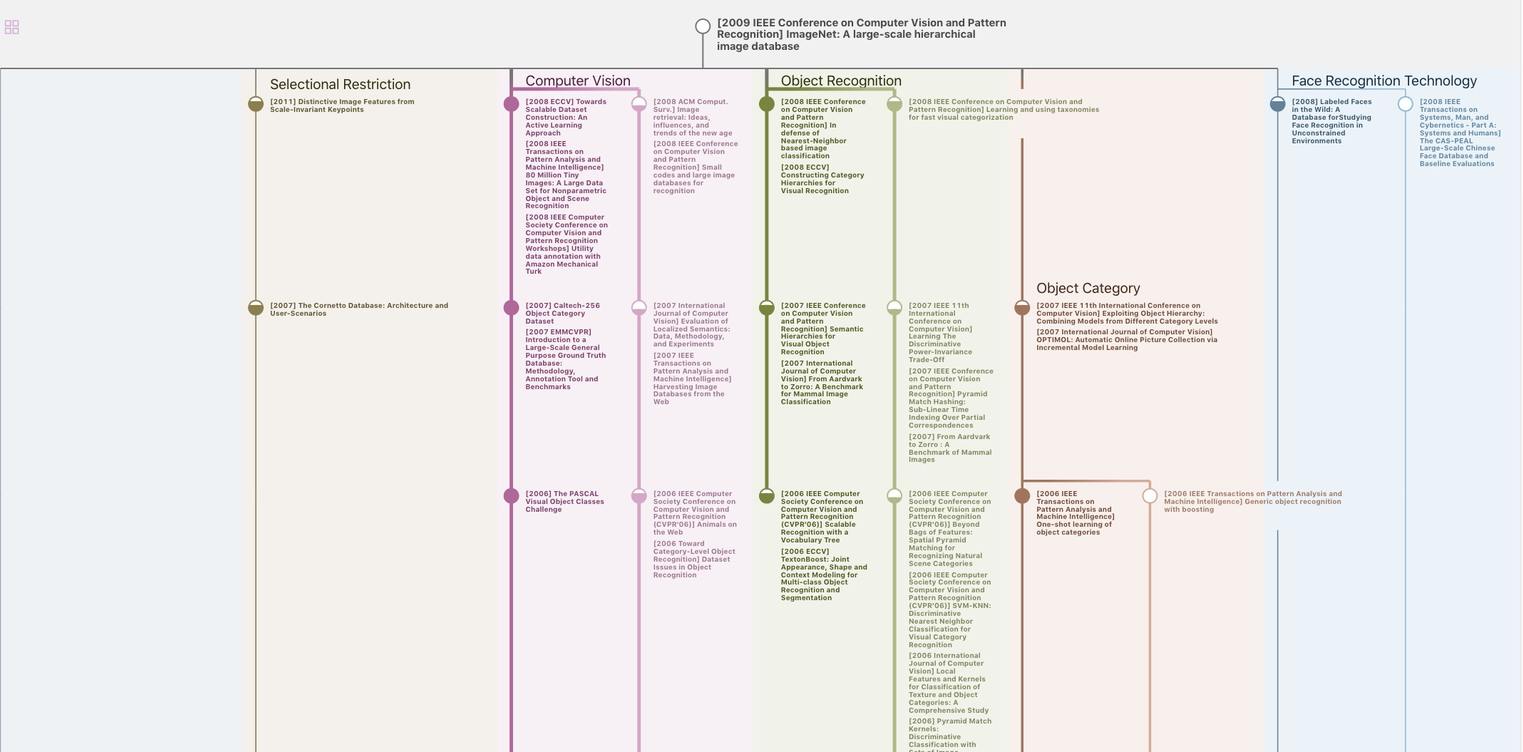
生成溯源树,研究论文发展脉络
Chat Paper
正在生成论文摘要