Occluded Object Detection for Autonomous Vehicles Employing YOLOv5, YOLOX and Faster R-CNN
2022 IEEE 13th Annual Information Technology, Electronics and Mobile Communication Conference (IEMCON)(2022)
摘要
Autonomous vehicles (AV s) are the future of trans-portation and are likely to bring countless benefits compared to human-operated driving. However, there are still a lot of advances to be made before these vehicles can be considered completely safe and before they can reach full autonomy. Perceiving the environment with the utmost accuracy and speed is crucial for autonomous vehicles. Hence, making this process more efficient and streamlined is of paramount importance. In order to perceive the environment, AV s need to classify and localize the different objects in the surrounding. For this research, we deal with the detection of occluded objects to help enhance the perception of AV s. We introduce a new dataset containing occluded instances of road scenes from the perspective of Bangladesh. We utilized transfer learning to train the YOLOv5, YOLOX, and Faster R-CNN models, using their respective pre-trained weights on the COCO dataset. We then evaluate and compare the performance of the three object detection algorithms on our dataset. YOLOv5, YOLOX, and Faster R-CNN achieved mean Average Precision (mAP) at 0.5 of 0.777, 0.849, and 0.688, and mAP at 0.5:0.95 of 0.546, 0.634, and 0.422, respectively, in our test set. Therefore, we find YOLOX to be the best performing model on our dataset, and its high mAP scores demonstrate the effectiveness of the model as well as the dataset.
更多查看译文
关键词
Autonomous Vehicles,Occluded Object Detection,Deep Learning,Transfer Learning,YOLOv5,YOLOX
AI 理解论文
溯源树
样例
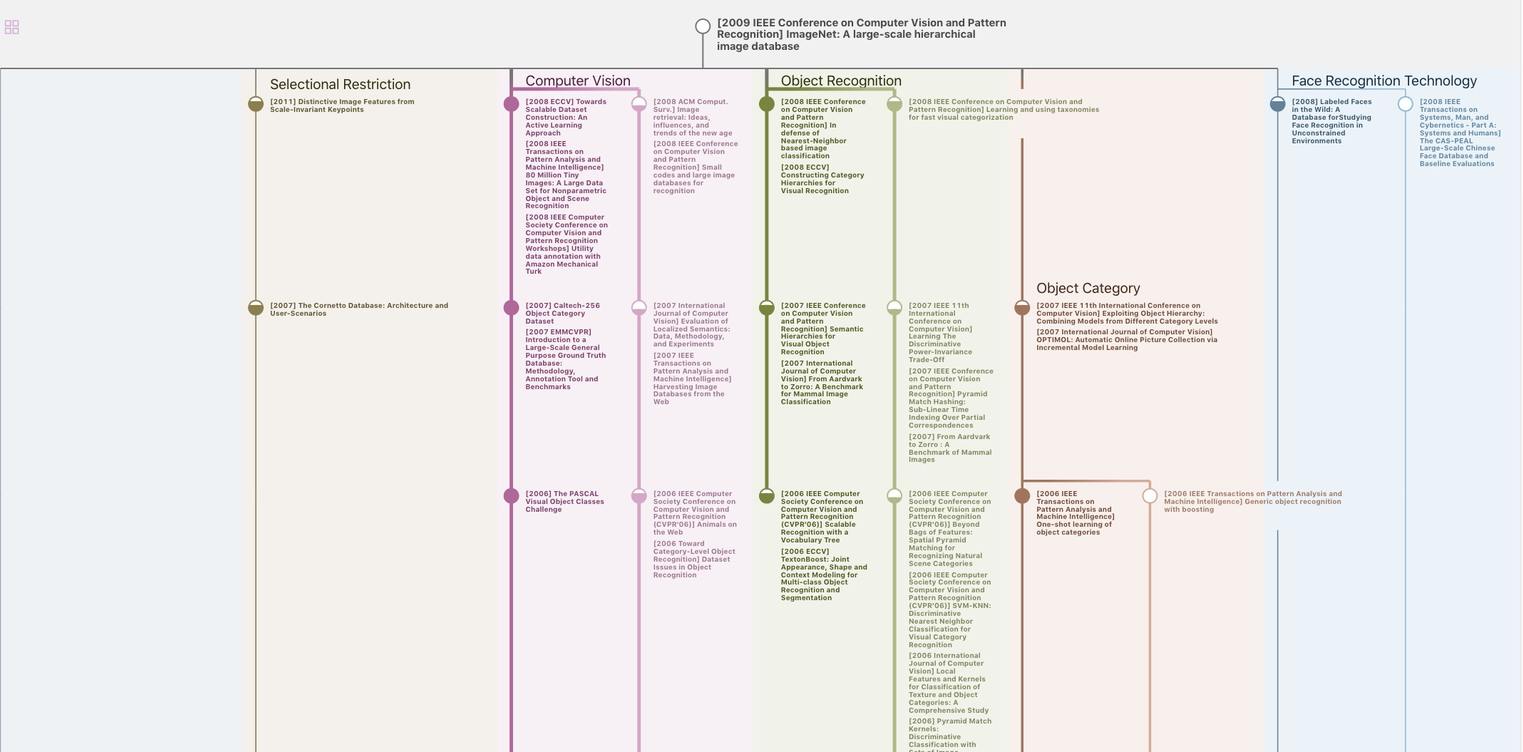
生成溯源树,研究论文发展脉络
Chat Paper
正在生成论文摘要