Weakly Supervised Learning for Network Traffic Classification
2022 IEEE International Conference on Networking, Architecture and Storage (NAS)(2022)
摘要
With advances in deep learning methods and an enormous amount of network traffic data, training deep neural networks for malware traffic classification directly with the raw traffic data has gained popularity and success. Obtaining labeled data to train deeper models, on the other hand, has recently become a significant challenge. Weakly supervised learning approaches that aim to improve the classification accuracy of classifiers previously trained with a small quantity of labeled data using widely available but unlabeled data have grown more popular as a solution to this problem. We propose employing several deep models as labeling functions instead of manual definition based on hand-crafted features, such as Snorkel approach, to estimate the labels that will be utilized to tune the classifier to increase its accuracy for network traffic classification using unlabeled data. In a multi-class classification scenario, our findings demonstrate that utilizing deep models to label the unlabeled chunk can enhance accuracy by 1.5 % and F1-score by 5%.
更多查看译文
关键词
Malware Analysis,Ransomware Detection,Machine Learning,Feature Engineering
AI 理解论文
溯源树
样例
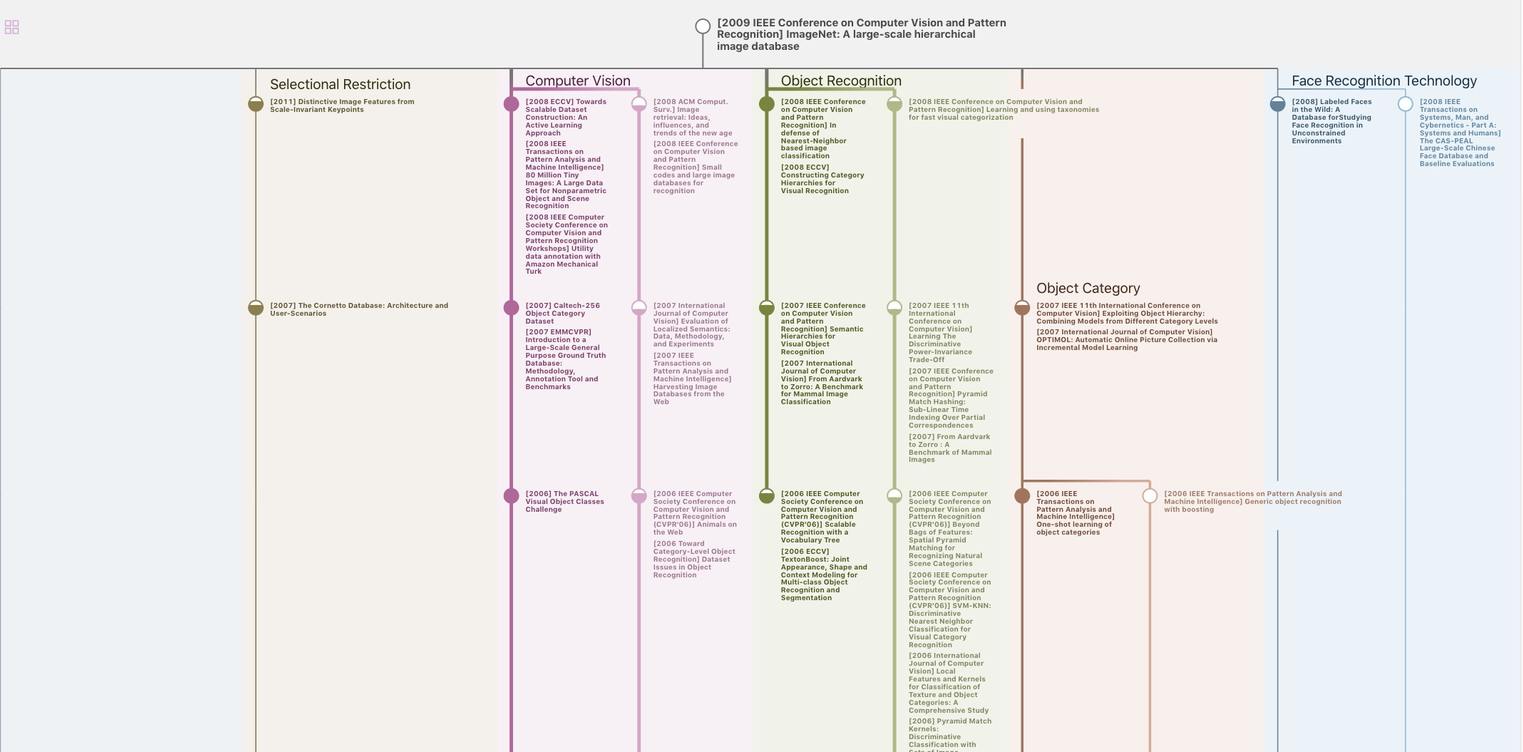
生成溯源树,研究论文发展脉络
Chat Paper
正在生成论文摘要