SoftVAD in iVector-Based Acoustic Scene Classification for Robustness to Foreground Speech
2022 30th European Signal Processing Conference (EUSIPCO)(2022)
摘要
To increase the robustness of Acoustic Scene Clas-sification (ASC) during foreground speech presence, we recently proposed a noise-floor based iVector framework exploiting the statistical estimate of the background signal spectrum. Thereby, ASC accuracy was greatly improved when foreground speech was predominant, at the cost of poorer performance in scenarios with low foreground speech levels. A soft Voice Activity Detector (softVAD) is introduced, here, to improve this trade-off. Three possibilities are investigated: (a) a segment-wise, weighted score fusion system, yielding a sof VAD-based weighted average of the output scores of the (classical) iVector framework and those of the noise-floor based iVector framework; (b) the introduction of weighted Baum-Welch statistics in the iVector extraction stage, with weights that emphasize the background-dominant frames and disregard speech-dominant frames in the test sequence. Based on the performance of these alternatives, a third approach (approach (c)) that performs segment-level score fusion of the frame-wise weighted statistics (approach (b)) and the noise-floor system is proposed. Experiments conclusively demonstrate that all proposals significantly improve the classification accuracy. Especially the last approach outperforms all other methods in a wide range of experimental conditions.
更多查看译文
关键词
Acoustic scene classification,iVector,softVAD,noise-floor estimation,foreground speech robustness
AI 理解论文
溯源树
样例
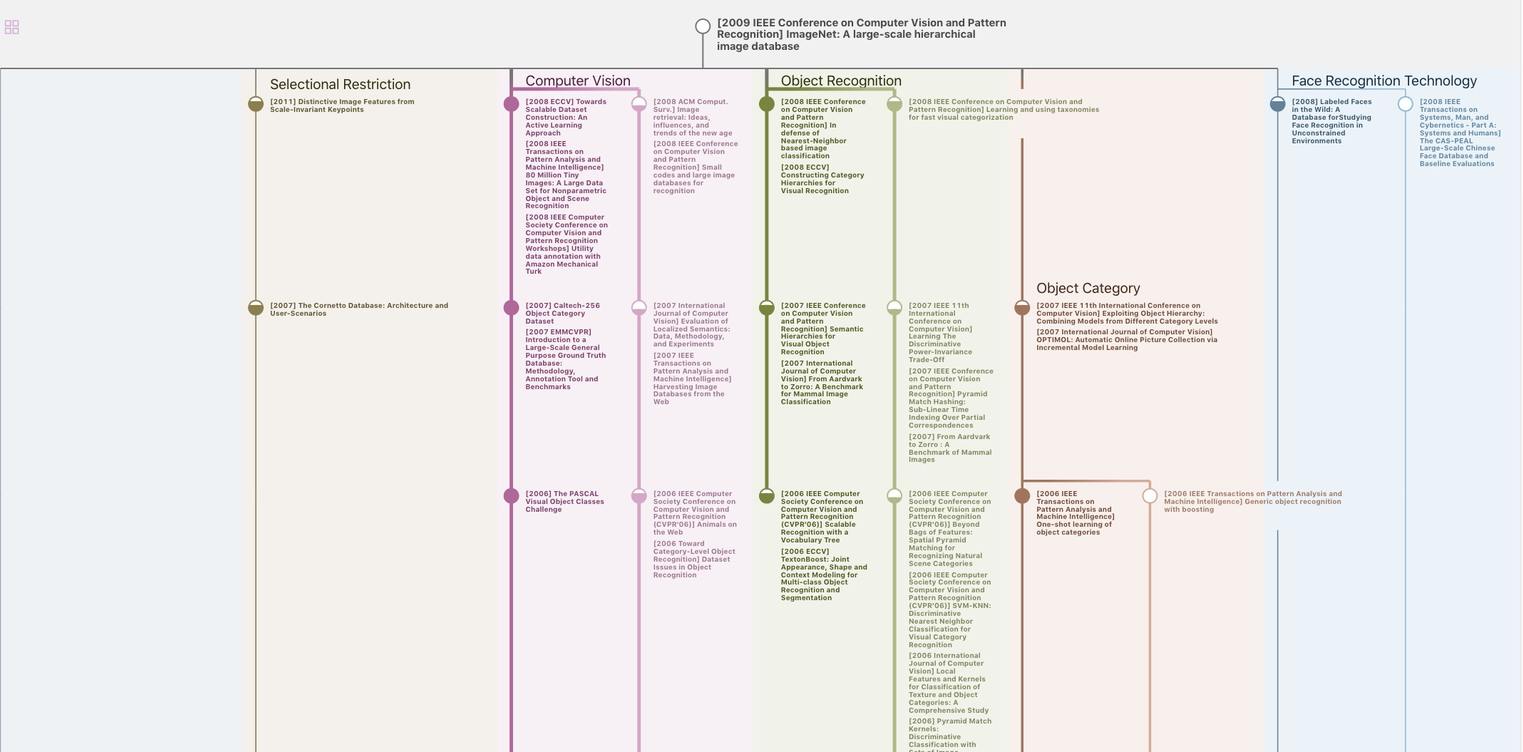
生成溯源树,研究论文发展脉络
Chat Paper
正在生成论文摘要