Unsupervised Acoustic Anomaly Detection Systems Based on Gaussian Mixture Density Neural Network
2022 30th European Signal Processing Conference (EUSIPCO)(2022)
摘要
Failure of critical industrial equipment leads to significant costs due to downtime and unplanned maintenance interventions, which in turn drives the demand for Acoustic Anomaly Detection (AAD). Previous approaches based on a deep Auto-Encoder (AE) followed by Gaussian Mixture Models (GMMs) have made significant progress. However, these tandem approaches suffer from a major weakness in that the dimensionality reduction of the feature stage and the density estimation stage are optimised separately. This paper proposed an unsupervised Gaussian Mixture Density Neural Network (GMDNN). In our method, instead of using a tandem two-stage AAD system, GMDNN jointly optimizes the dimension reduction network that generates a low-dimensional representation, the reconstruction contrast network that produces contrastive features, and the density estimation network with improved end-to-end anomaly detection performance. Experimental results on MIMII DUE industrial datasets show that GMDNN significantly outperforms previous approaches and improves up to 14.7% based on the mean of area under the curve (AUC) score.
更多查看译文
关键词
Acoustic anomalous detection,joint-training,Gaussian mixture density estimation network,unsupervised learning
AI 理解论文
溯源树
样例
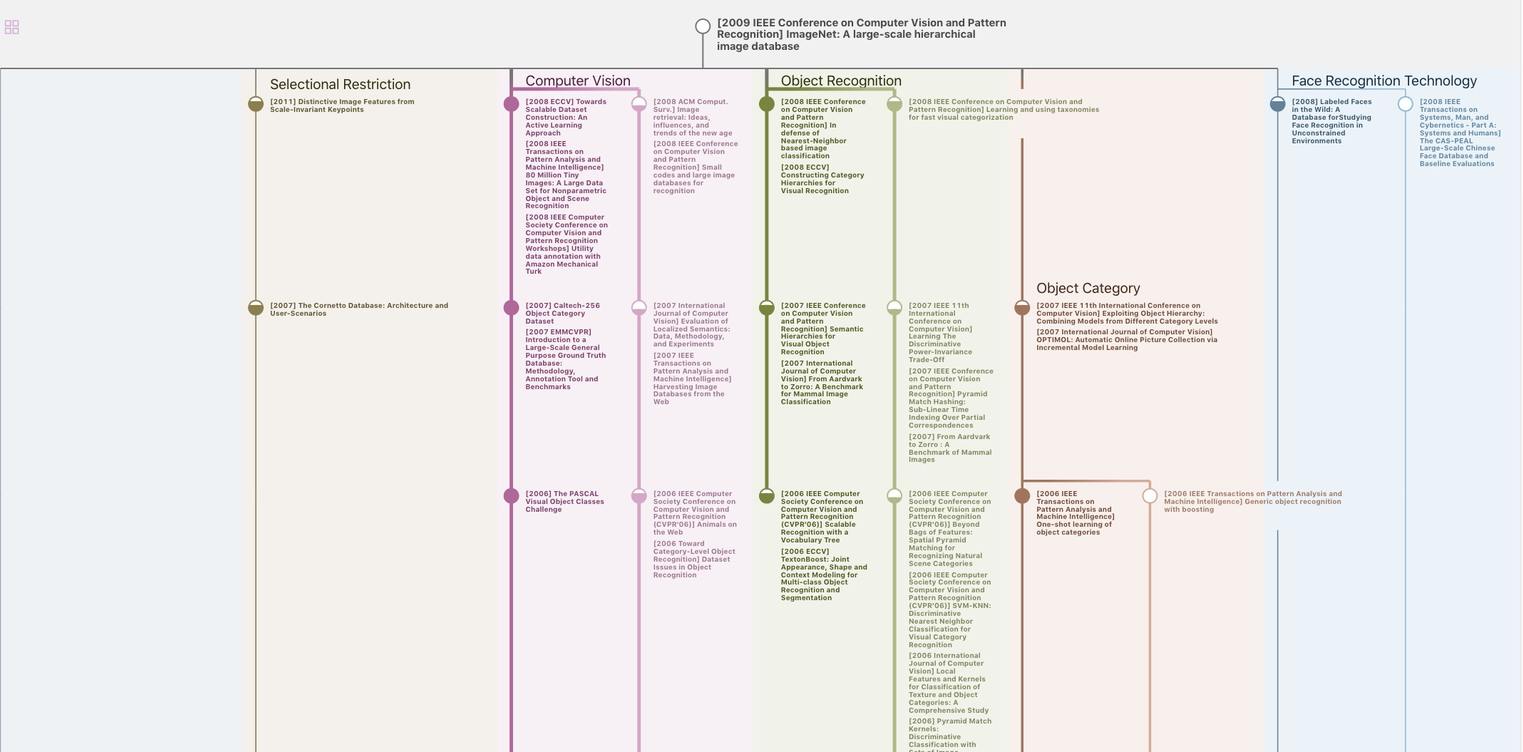
生成溯源树,研究论文发展脉络
Chat Paper
正在生成论文摘要