Predicting Programming Success: How Intermittent Knowledge Assessments, Individual Psychometrics, and Resting-State EEG Predict Python Programming and Debugging Skills
2022 International Conference on Software, Telecommunications and Computer Networks (SoftCOM)(2022)
摘要
Computer programming requires fluid application of acquired chunks of declarative knowledge to accomplish a defined goal. This raises the question-how strongly do declarative knowledge assessments collected during training predict individual learners' future programming capabilities, and how might neurocognitive measures expand these predictions? The current study explored this by using stepwise regression to determine whether neurocognitive characteristics of individual learners and post-module declarative assessments collected in the Codecademy learning platform explain unique or overlapping variance when predicting real-world coding outcomes. Based on data from 80 participants over 16 one-hour Python training sessions, we found that post-module declarative knowledge assessments explained the most variance in each of our seven learning outcomes: multiple-choice test accuracy, programming accuracy, and debugging accuracy (collected at two time points) plus learning rate. However, neurocognitive measures also contributed unique variance, with total variance explained varying across outcomes. Our preliminary results suggest that declarative knowledge and neurocognitive indices combine in different proportions to predict different types of programming outcomes.
更多查看译文
关键词
individual differences,skill learning,computer programming,debugging,complex skills,knowledge assessment,learning technologies
AI 理解论文
溯源树
样例
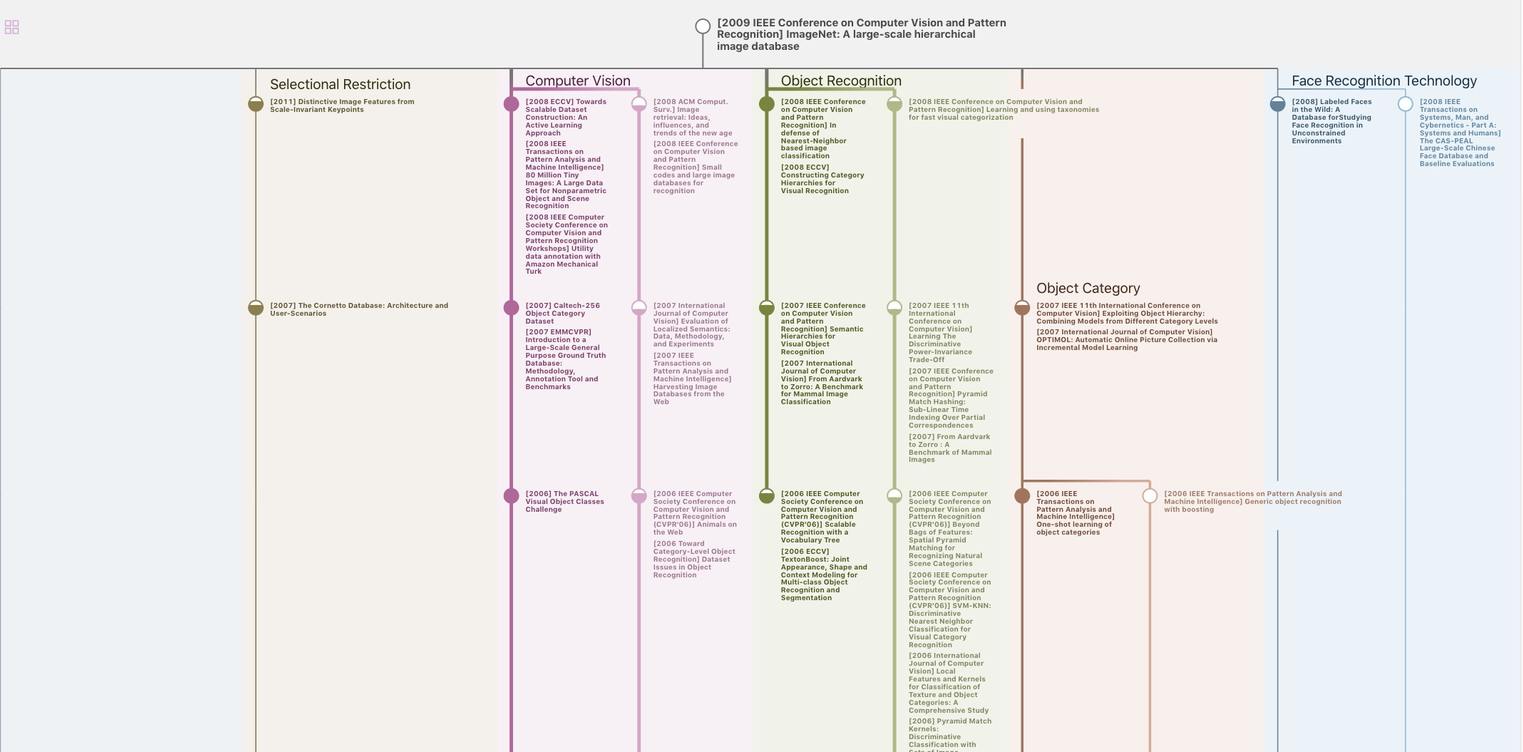
生成溯源树,研究论文发展脉络
Chat Paper
正在生成论文摘要