Task Detection in Continual Learning via Familiarity Autoencoders
2022 IEEE International Conference on Systems, Man, and Cybernetics (SMC)(2022)
摘要
Continual learning requires the ability to reliably transfer previously learned knowledge to new tasks without disrupting established competencies. Methods such as Progressive Neural Network [1] accomplish high-quality transfer learning while nullifying the insidious problem of catastrophic forgetting. However, most module-based continual learning systems require task labels during operation – a constraint that limits their application in many real-world conditions where task indicators are opaque. This paper proposes a task detector neural algorithm to acquire task information while maintaining immunity to forgetting. Our proposed task detector allows progressive neural networks (and many similar systems) to operate without task labels during test time. Our task detector is built from familiarity autoencoders which recognize the nature of the required task from input data. We demonstrate the generality and effectiveness of our approach through experiments in video game playing and automated image repair. Our results show near-perfect task recognition in all domains (>.99 F1), rewards above published single-task scores in MinAtar, and realistic image repairs on damaged human face pictures. The performance of our integrated method is nearly identical to the progressive systems equipped with ground-truth task labels
1
更多查看译文
AI 理解论文
溯源树
样例
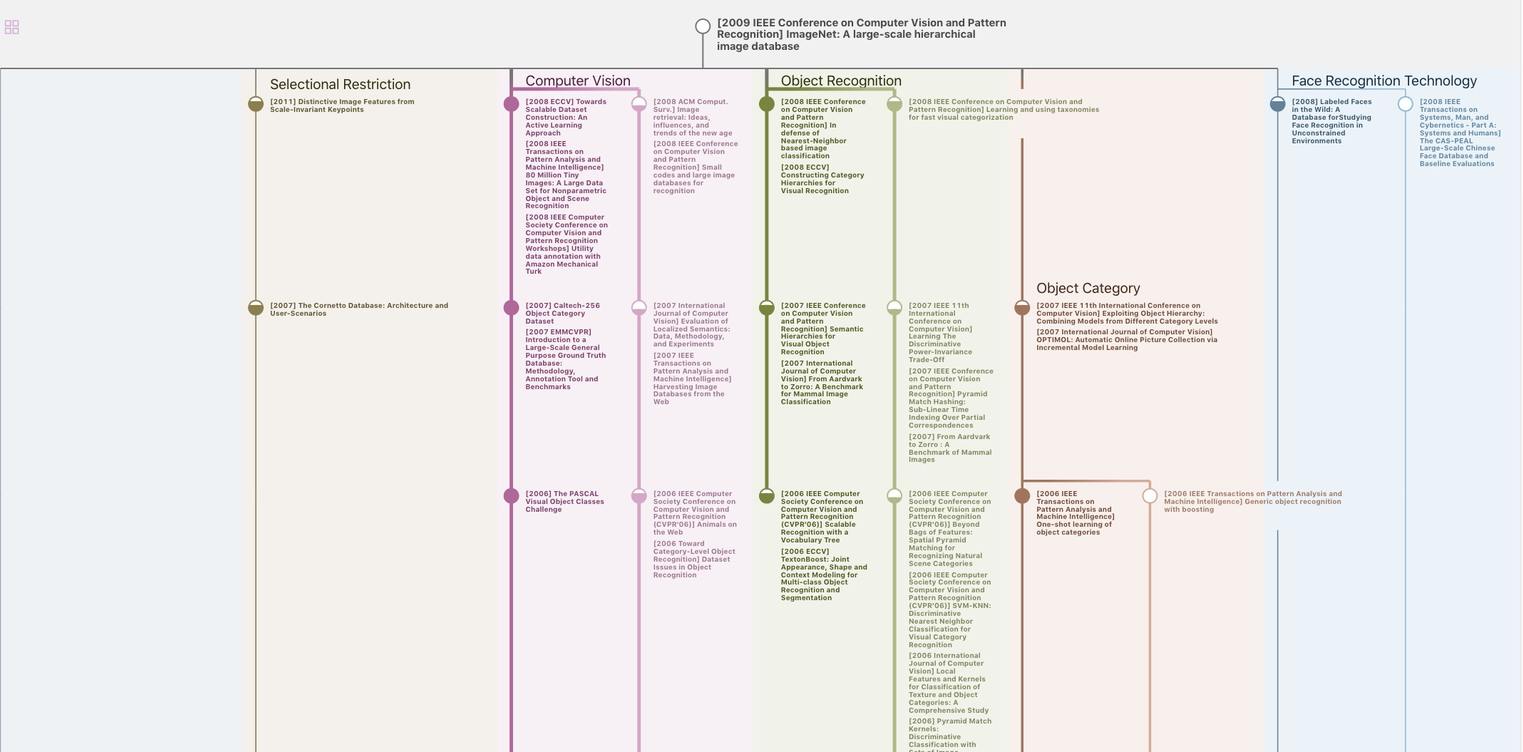
生成溯源树,研究论文发展脉络
Chat Paper
正在生成论文摘要