Adapting without forgetting: KnowBert-UMLS
2022 18th International Conference on Wireless and Mobile Computing, Networking and Communications (WiMob)(2022)
摘要
Domain adaptation in pretrained language models usually comes at some cost, most notably out-of-domain performance. This type of specialization typically relies on pre-training over a large in-domain corpus, which has the side effect of causing catastrophic forgetting on general text. We seek to specialize a language model by incorporating information from a knowledge base into its contextualized representations, thus reducing its reliance on specialized text. We achieve this by following the KnowBert method, applied to the UMLS biomedical knowledge base. We evaluate our model on in-domain and out-of-domain tasks, comparing against BERT and other specialized models. We find that our performance on biomedical tasks is competitive with the state-of-the-art with virtually no loss of generality. Our results demonstrate the applicability of this knowledge integration technique to the biomedical domain as well as its shortcomings. The reduced risk of catastrophic forgetting displayed by this approach to domain adaptation broadens the scope of applicability of specialized language models.
更多查看译文
关键词
Knowledge based systems,Deep learning,Trans-fer learning,Biomedical Computing,Natural language processing
AI 理解论文
溯源树
样例
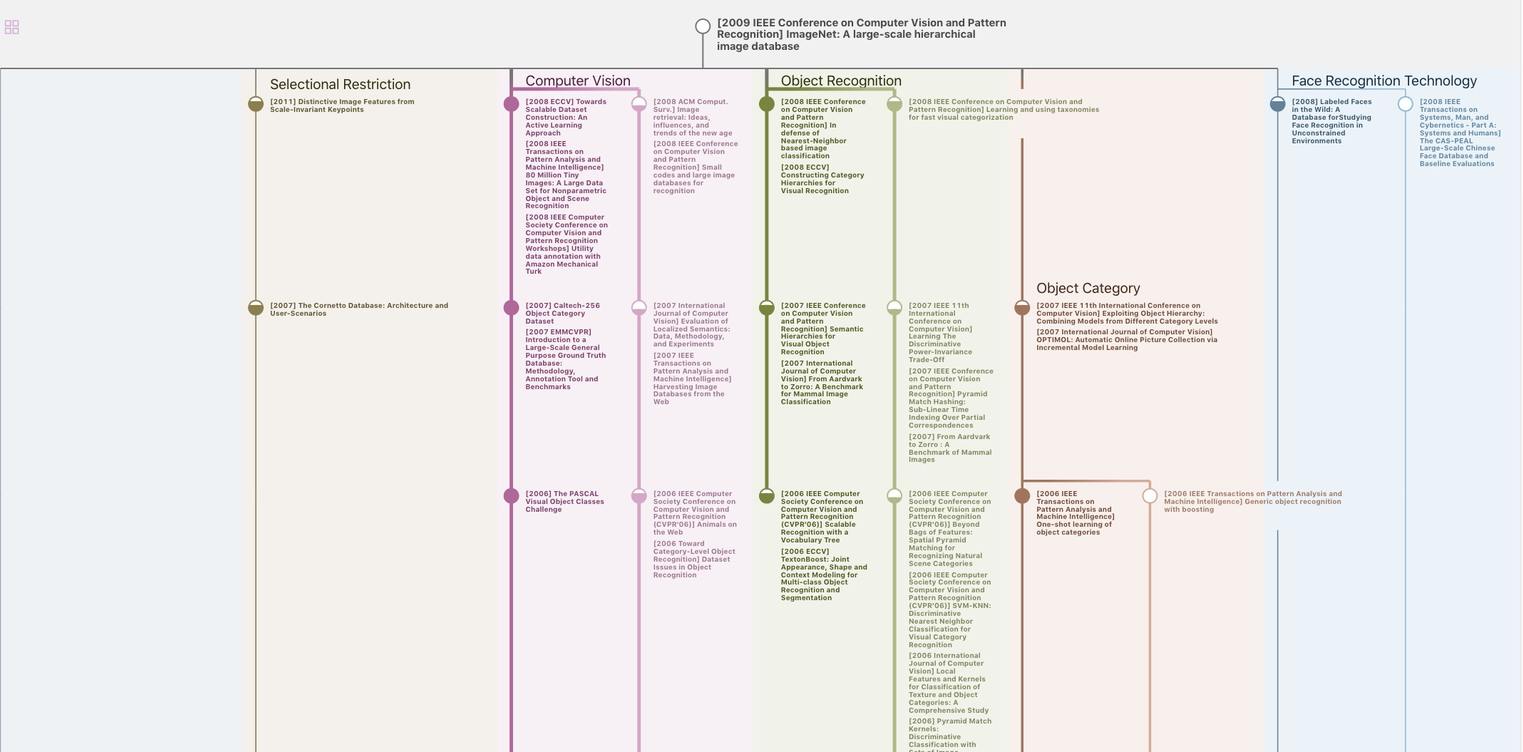
生成溯源树,研究论文发展脉络
Chat Paper
正在生成论文摘要