An Empirical Study on the Bugs Found while Reusing Pre-trained Natural Language Processing Models
arxiv(2022)
摘要
In NLP, reusing pre-trained models instead of training from scratch has gained popularity; however, NLP models are mostly black boxes, very large, and often require significant resources. To ease, models trained with large corpora are made available, and developers reuse them for different problems. In contrast, developers mostly build their models from scratch for traditional DL-related problems. By doing so, they have control over the choice of algorithms, data processing, model structure, tuning hyperparameters, etc. Whereas in NLP, due to the reuse of the pre-trained models, NLP developers are limited to little to no control over such design decisions. They either apply tuning or transfer learning on pre-trained models to meet their requirements. Also, NLP models and their corresponding datasets are significantly larger than the traditional DL models and require heavy computation. Such reasons often lead to bugs in the system while reusing the pre-trained models. While bugs in traditional DL software have been intensively studied, the nature of extensive reuse and black-box structure motivates us to understand the different types of bugs that occur while reusing NLP models? What are the root causes of those bugs? How do these bugs affect the system? To answer these questions, We studied the bugs reported while reusing the 11 popular NLP models. We mined 9,214 issues from GitHub repositories and identified 984 bugs. We created a taxonomy with bug types, root causes, and impacts. Our observations led to several findings, including limited access to model internals resulting in a lack of robustness, lack of input validation leading to the propagation of algorithmic and data bias, and high-resource consumption causing more crashes, etc. Our observations suggest several bug patterns, which would greatly facilitate further efforts in reducing bugs in pre-trained models and code reuse.
更多查看译文
关键词
bugs,language,empirical study,pre-trained
AI 理解论文
溯源树
样例
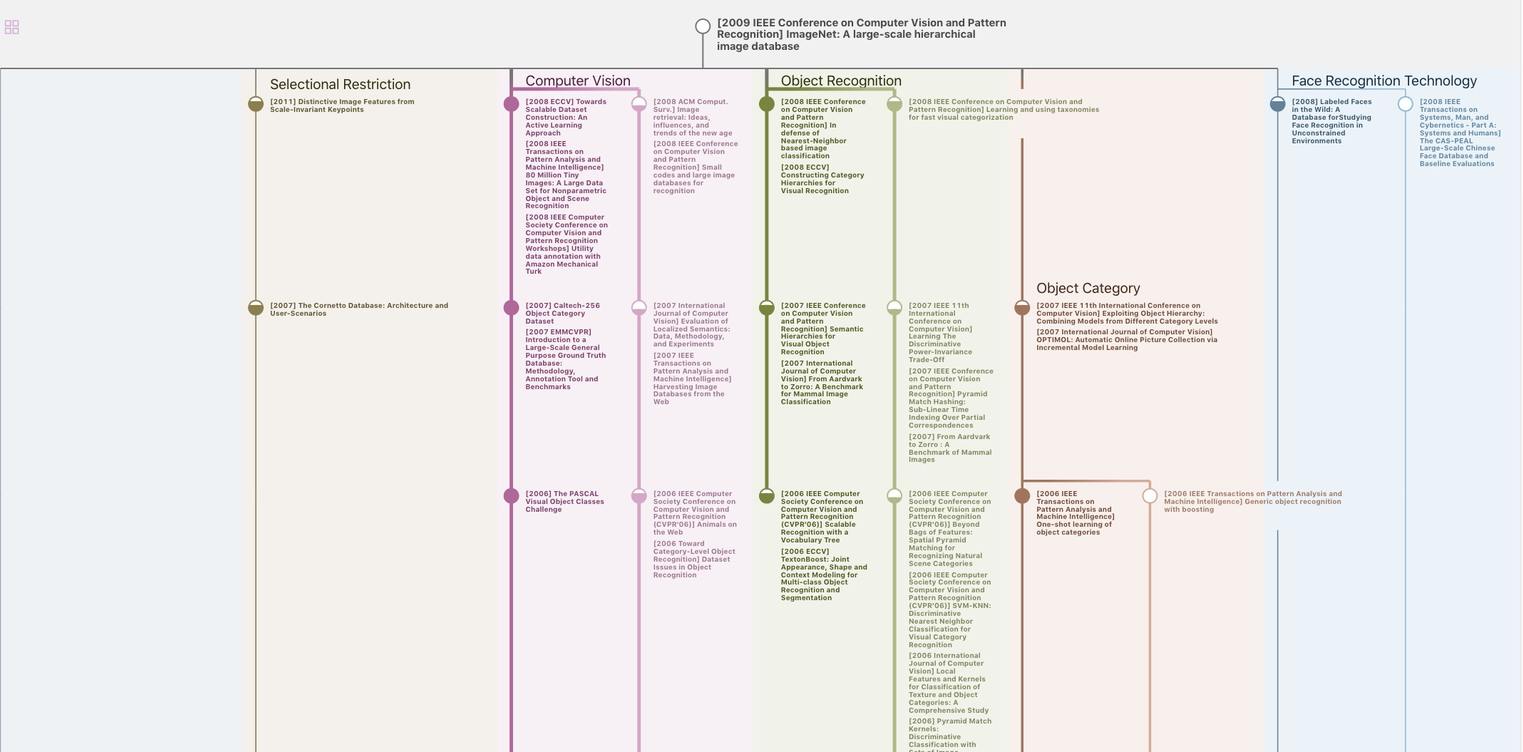
生成溯源树,研究论文发展脉络
Chat Paper
正在生成论文摘要