Application of Residual Network Architecture on Covid-19 Chest x-ray Classification
2022 International Symposium on Information Technology and Digital Innovation (ISITDI)(2022)
摘要
Convolutional Neural Network (CNN) has proven with good performance in the area of feature extraction. Classification of medical images is often faced with the lack of sufficient amounts of data. Therefore, Transfer Learning can be applied to overcome these problems. Chest x-ray data are complex and require deeper layers for specific features. Resnet built with deep layers specifically focuses on problems that often occur in high-depth architectures, which are prone to decreased accuracy and training errors. Some of the aspects are able to affect the performance of the model such as the depth of convolution layers and training procedures, which include data splitting technique and Optimizers. In this study, the Hold Out data splitting and k-fold cross validation of 5 folds with Optimizer Adaptive Moment Estimation (Adam) and Stochastic Gradient Descent (SGD) on the Resnet-50 and Resnet-101 architectures. The training procedure was applied to 15143 Chest x-ray images measuring 224x224 pixels with parameters epoch 50 and batch size 100. The best value was obtained using k-fold cross validation on Resnet-50 using the SGD optimizer with 99% accuracy.
更多查看译文
关键词
Resnet,Hold out,k-fold cross validation,Adam,SGD
AI 理解论文
溯源树
样例
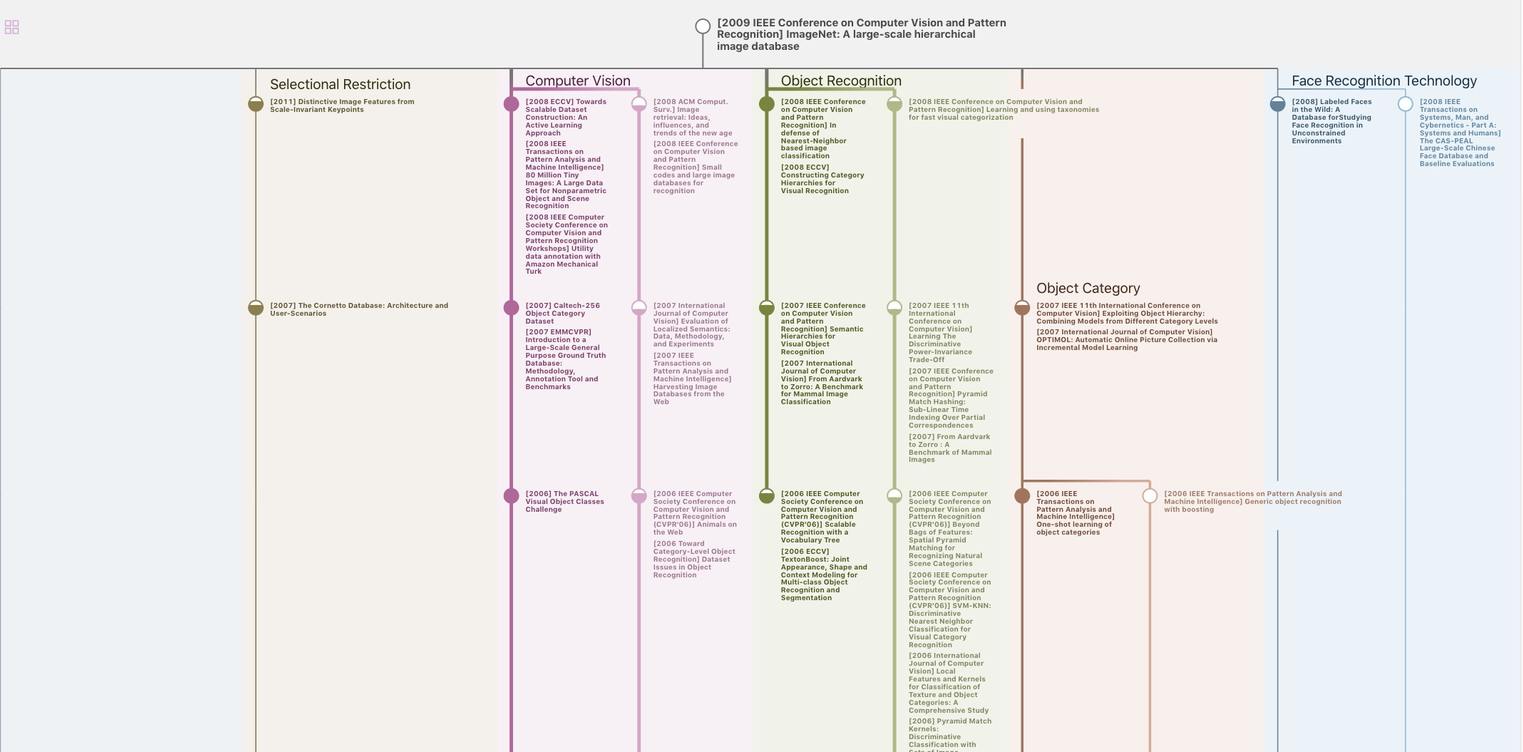
生成溯源树,研究论文发展脉络
Chat Paper
正在生成论文摘要