Tuning-free Plug-and-Play Hyperspectral Image Deconvolution with Deep Priors
IEEE Transactions on Geoscience and Remote Sensing(2023)
Abstract
Deconvolution is a widely used strategy to mitigate the blurring and noisy degradation of hyperspectral images~(HSI) generated by the acquisition devices. This issue is usually addressed by solving an ill-posed inverse problem. While investigating proper image priors can enhance the deconvolution performance, it is not trivial to handcraft a powerful regularizer and to set the regularization parameters. To address these issues, in this paper we introduce a tuning-free Plug-and-Play (PnP) algorithm for HSI deconvolution. Specifically, we use the alternating direction method of multipliers (ADMM) to decompose the optimization problem into two iterative sub-problems. A flexible blind 3D denoising network (B3DDN) is designed to learn deep priors and to solve the denoising sub-problem with different noise levels. A measure of 3D residual whiteness is then investigated to adjust the penalty parameters when solving the quadratic sub-problems, as well as a stopping criterion. Experimental results on both simulated and real-world data with ground-truth demonstrate the superiority of the proposed method.
MoreTranslated text
Key words
deep priors,tuning-free,plug-and-play
AI Read Science
Must-Reading Tree
Example
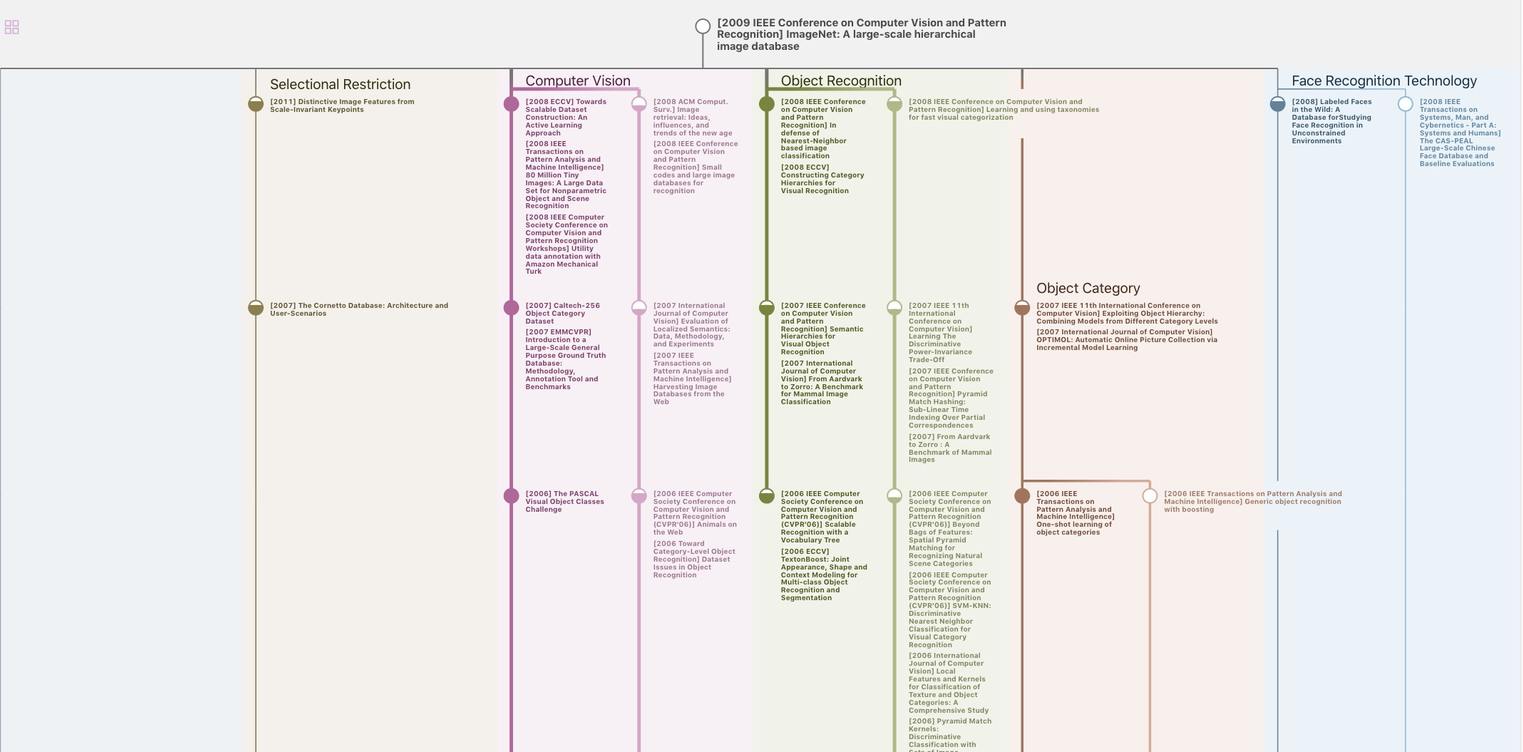
Generate MRT to find the research sequence of this paper
Chat Paper
Summary is being generated by the instructions you defined