Continuous diffusion for categorical data
arxiv(2022)
摘要
Diffusion models have quickly become the go-to paradigm for generative modelling of perceptual signals (such as images and sound) through iterative refinement. Their success hinges on the fact that the underlying physical phenomena are continuous. For inherently discrete and categorical data such as language, various diffusion-inspired alternatives have been proposed. However, the continuous nature of diffusion models conveys many benefits, and in this work we endeavour to preserve it. We propose CDCD, a framework for modelling categorical data with diffusion models that are continuous both in time and input space. We demonstrate its efficacy on several language modelling tasks.
更多查看译文
关键词
continuous diffusion,data
AI 理解论文
溯源树
样例
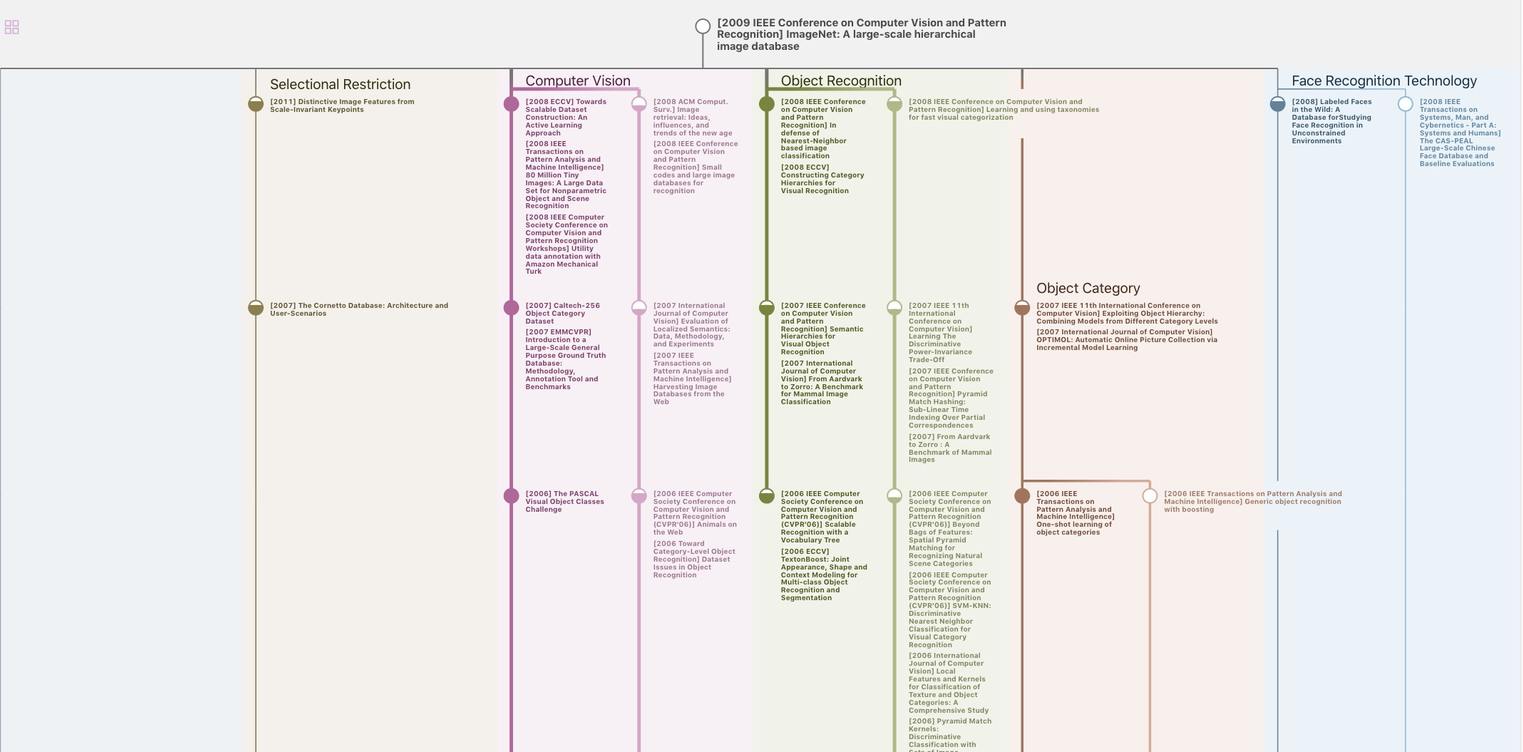
生成溯源树,研究论文发展脉络
Chat Paper
正在生成论文摘要